LIMITED OFFER
Save 50% on book bundles
Immediately download your ebook while waiting for your print delivery. No promo code needed.
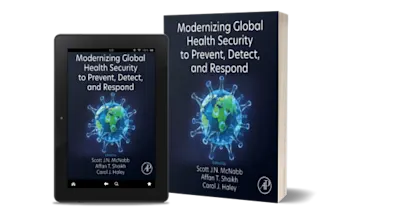
Machine Learning Models and Architectures for Biomedical Signal Processing presents the fundamental concepts of machine learning techniques for bioinformatics in an intera… Read more
LIMITED OFFER
Immediately download your ebook while waiting for your print delivery. No promo code needed.
ST
Suman Lata Tripathi completed her PhD in the area of microelectronics and VLSI from MNNIT, Allahabad. She was also a remote post-doc researcher at Nottingham Trent University, London, UK in 2022. She is a Professor at Lovely Professional University with more than 19 years of experience in academics. She has published more than 89 research papers in refereed journals and conferences. She has also published 13 Indian patents and 2 copyrights. She has organized several workshops, summer internships, and expert lectures for students. She has worked as a session chair, conference steering committee member, editorial board member, and peer reviewer in international/national conferences. She received the “Research Excellence Award” in 2019 and “Research Appreciation Award” in 2020, 2021 at Lovely Professional University, India. She also received funded projects from SERB DST under the scheme TARE in the area of Microelectronics devices. She has edited or authored more than 15 books in different areas of Electronics and electrical engineering. Her areas of expertise includes microelectronics device modeling and characterization, low power VLSI circuit design, VLSI design of testing, and advanced FET design for IoT, Embedded System Design, reconfigurable architecture with FPGAs and biomedical applications.
VB
MM
SB