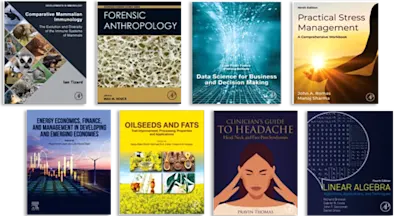
Machine Learning for Small Bodies in the Solar System
- 1st Edition - October 29, 2024
- Imprint: Elsevier
- Editors: Valerio Carruba, Evgeny Smirnov, Dagmara Oszkiewicz
- Language: English
- Paperback ISBN:9 7 8 - 0 - 4 4 3 - 2 4 7 7 0 - 5
- eBook ISBN:9 7 8 - 0 - 4 4 3 - 2 4 7 7 1 - 2
Machine Learning for Small Bodies in the Solar System provides the latest developments and methods in applications of Machine Learning (ML) and Artificial Intelligence… Read more
Purchase options
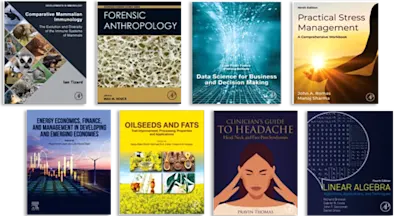
Institutional subscription on ScienceDirect
Request a sales quote- Provides a practical reference to applications of machine learning and artificial intelligence to small bodies in the Solar System
- Approaches the topic from a multidisciplinary perspective, with chapters on dynamics, physical properties and software development
- Includes code and links to publicly available repositories to allow readers practice the methodology covered
- Artificial intelligence and machine learning methods in celestial mechanics
- Identification of asteroid families’ members
- Asteroids inmean-motion resonances
- Asteroid families interacting with secular resonances
- Neural networks in celestial dynamics: capabilities, advantages, and challenges in orbital dynamics around asteroids
- Asteroid spectro-photometric characterization
- Machine learning-assisted dynamical classification of trans-Neptunian objects
- Identification and localization of cometary activity in Solar System objects withmachine learning
- Detectingmoving objects with machine learning
- Chaotic dynamics
- Conclusions and future developments
- Edition: 1
- Published: October 29, 2024
- No. of pages (Paperback): 328
- Imprint: Elsevier
- Language: English
- Paperback ISBN: 9780443247705
- eBook ISBN: 9780443247712
VC
Valerio Carruba
Prof. Valerio Carruba is currently an Associate Professor at the São Paulo State University (UNESP) in Brazil. He is one of the founders of the Machine Learning applied to Small Bodies (MASB) research group. His recent interests involve the use of deep learning for the identification of asteroids in secular resonant configurations and machine learning applied for asteroid families’ identification. Asteroid 10741 has been named “Valeriocarruba” by the International Astronomical Union. His paper “Optimization of artificial neural networks models applied to the identification of images of asteroids’ resonant arguments” recently won the CELMEC prize for "Innovative computational methods in Dynamical Astronomy".
ES
Evgeny Smirnov
Dr. Evgeny Smirnov works in the field of the dynamics of asteroids. In 2017, he introduced a machine learning approach based on the supervised learning for the identification procedure that decreases the computational time from weeks to seconds. In the same year, he proposed a similar approach for asteroid families instead of the classical HCM method. With a strong background in science and software development, Evgeny connects these areas and brings modern software development patterns and techniques into the field of astronomy.
DO
Dagmara Oszkiewicz
Prof. Dagmara Oszkiewicz is a Polish astronomer and planetary scientist. She is an assistant professor at Adam Mickiewicz University in Poznań, Poland, where her research focuses on physical and orbital properties of small Solar System bodies. She has recently expanded her research to include machine learning techniques to the analysis of asteroid spectro-photometric data; her latest work includes applications of machine learning algorithms to the classification of basaltic asteroids in the context of formation of differentiated planetesimals and comparison of various machine learning algorithms for classification of spectro-photometric data from various large sky surveys.