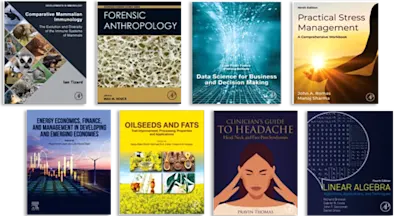
Machine Learning Applications in Industrial Solid Ash
- 1st Edition - December 1, 2023
- Imprint: Elsevier
- Authors: Chongchong Qi, Qiusong Chen, Erol Yilmaz
- Language: English
- Paperback ISBN:9 7 8 - 0 - 4 4 3 - 1 5 5 2 4 - 6
- eBook ISBN:9 7 8 - 0 - 4 4 3 - 1 5 5 2 5 - 3
Machine Learning Applications in Industrial Solid Ash begins with fundamentals in solid ash, covering the status of solid ash generation and management. The book moves on to founda… Read more
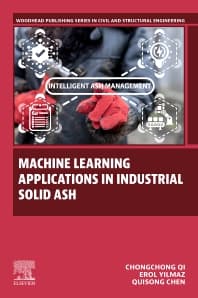
Purchase options
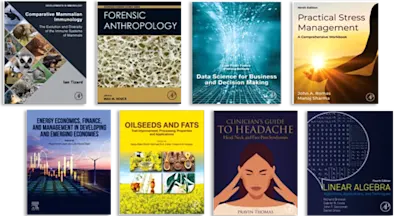
Institutional subscription on ScienceDirect
Request a sales quoteMachine Learning Applications in Industrial Solid Ash begins with fundamentals in solid ash, covering the status of solid ash generation and management. The book moves on to foundational knowledge on ML in solid ash management, which provides a brief introduction of ML for solid ash applications. The reference then goes on to discuss ML approaches currently used to address problems in solid ash management and recycling, including solid ash generation, clustering analysis, origin identification, reactivity prediction, leaching potential modelling and metal recovery evaluation, etc. Finally, potential future trends and challenges in the field are discussed.
Offering the ability to process large or complex datasets, machine learning (ML) holds huge potential to reshape the whole status for solid ash management and recycling. This book is the first published book about ML in solid ash management and recycling. It highlights fundamental knowledge and recent advances in this topic, offering readers new insight into how these tools can be utilized to enhance their own work.
- Helps readers increase their existing knowledge on data mining and ML
- Teaches how to apply ML techniques that work best in solid ash management and recycling through providing illustrative examples and complex practice solutions
- Provides an accessible introduction to the current state and future possibilities for ML in solid ash management and recycling
- Cover image
- Title page
- Table of Contents
- Copyright
- Dedication
- Preface
- Acknowledgments
- 1. Industrial solid ashes generation
- Abstract
- 1.1 Introduction
- 1.2 Making and types of industrial solid ashes
- 1.3 Production amounts of industrial solid ashes
- References
- 2. Properties of industrial solid ashes
- Abstract
- 2.1 Introduction
- 2.2 Characteristics of coal ashes
- 2.3 Characteristics of MSW bottom/fly ash
- 2.4 Characteristics of biomass bottom/fly ash
- References
- 3. Ash management, recycling, and sustainability
- Abstract
- 3.1 Introduction
- 3.2 Management of CFA and CBA
- 3.3 MSW bottom ash and fly ash
- 3.4 Biomass bottom/fly ashes
- References
- 4. Emerging innovative techniques for ash management
- Abstract
- 4.1 Introduction
- 4.2 Geopolymer
- 4.3 Recovery of REEs
- 4.4 Carbon nanotubes
- 4.5 Catalysis
- 4.6 Silica aerogel
- References
- 5. Legal framework for ashes
- Abstract
- 5.1 Introduction
- 5.2 Review of coal fly/bottom ash regulations
- 5.3 MSW/biomass ash regulations
- References
- 6. Background of machine learning
- Abstract
- 6.1 History of machine learning
- 6.2 Machine learning categories
- 6.3 Deep learning
- 6.4 Introduction to machine learning techniques
- 6.5 Implementation of machine learning
- References
- 7. Machine learning modeling methodology for industrial solid ash
- Abstract
- 7.1 Introduction
- 7.2 Dataset collection and preprocessing
- 7.3 Machine learning modeling
- 7.4 Modeling evaluation
- 7.5 Machine learning–based knowledge discovery
- References
- 8. The application of clustering algorithms for industrial solid ashes based on physicochemical properties
- Abstract
- 8.1 Background
- 8.2 Methodology
- 8.3 Results and discussion
- 8.4 Summary
- References
- 9. The accurate production forecast of solid ashes: application and comparison of machine learning techniques
- Abstract
- 9.1 Background
- 9.2 Dataset
- 9.3 Methodology
- 9.4 Results and discussion
- 9.5 Comparison with multiple linear regression
- 9.6 Feature importance analysis
- 9.7 Significance and outlook
- 9.8 Summary
- References
- 10. FIELD: fast mobility evaluation and environmental index for solid ashes with machine learning
- Abstract
- 10.1 Background
- 10.2 Methodology
- 10.3 The application of FIELD to coal fly ash
- 10.4 Summary
- References
- 11. Identifying the amorphous content in solid ashes: a machine learning approach using an international dataset
- Abstract
- 11.1 Background
- 11.2 Modeling methodology for the amorphous phase
- 11.3 Results and discussion
- References
- 12. The reactivity classification of coal fly ash based on the random forest method
- Abstract
- 12.1 Background
- 12.2 Basics of machine learning modeling
- 12.3 Fly ash reactivity classification modeling
- 12.4 Tuning of model parameters and analysis of evaluation results
- 12.5 Postanalysis of the model
- 12.6 Summary
- References
- 13. Forecasting the uniaxial compressive strength of solid ash-based concrete
- Abstract
- 13.1 Background
- 13.2 Materials and method
- 13.3 Results and discussion
- 13.4 Summary
- References
- 14. Challenges and future perspectives of machine learning in industrial solid ashes management
- Abstract
- 14.1 Major challenges of current studies
- 14.2 Future perspectives
- References
- Appendix
- Index
- Edition: 1
- Published: December 1, 2023
- Imprint: Elsevier
- No. of pages: 314
- Language: English
- Paperback ISBN: 9780443155246
- eBook ISBN: 9780443155253
CQ