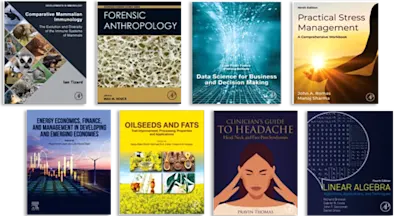
Machine Learning and the Internet of Medical Things in Healthcare
- 1st Edition - April 14, 2021
- Imprint: Academic Press
- Editors: Krishna Kant Singh, Mohamed Elhoseny, Akansha Singh, Ahmed A. Elngar
- Language: English
- Paperback ISBN:9 7 8 - 0 - 1 2 - 8 2 1 2 2 9 - 5
- eBook ISBN:9 7 8 - 0 - 1 2 - 8 2 3 2 1 7 - 0
Machine Learning and the Internet of Medical Things in Healthcare discusses the applications and challenges of machine learning for healthcare applications. The book provides… Read more
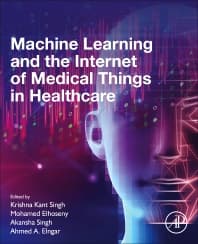
Purchase options
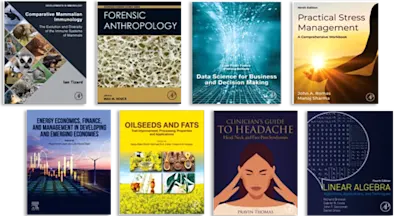
Institutional subscription on ScienceDirect
Request a sales quoteMachine Learning and the Internet of Medical Things in Healthcare
discusses the applications and challenges of machine learning for healthcare applications. The book provides a platform for presenting machine learning-enabled healthcare techniques and offers a mathematical and conceptual background of the latest technology. It describes machine learning techniques along with the emerging platform of the Internet of Medical Things used by practitioners and researchers worldwide.The book includes deep feed forward networks, regularization, optimization algorithms, convolutional networks, sequence modeling, and practical methodology. It also presents the concepts of the Internet of Things, the set of technologies that develops traditional devices into smart devices. Finally, the book offers research perspectives, covering the convergence of machine learning and IoT. It also presents the application of these technologies in the development of healthcare frameworks.
- Provides an introduction to the Internet of Medical Things through the principles and applications of machine learning
- Explains the functions and applications of machine learning in various applications such as ultrasound imaging, biomedical signal processing, robotics, and biomechatronics
- Includes coverage of the evolution of healthcare applications with machine learning, including Clinical Decision Support Systems, artificial intelligence in biomedical engineering, and AI-enabled connected health informatics, supported by real-world case studies
- Cover image
- Title page
- Table of Contents
- Copyright
- List of contributors
- Chapter 1. Machine learning architecture and framework
- Abstract
- 1.1 Introduction
- 1.2 Architecture of machine learning
- 1.3 Machine learning framework
- 1.4 Significance of machine learning in the healthcare system
- 1.5 Conclusion
- References
- Chapter 2. Machine learning in healthcare: review, opportunities and challenges
- Abstract
- 2.1 Introduction
- 2.2 Analysis of domain
- 2.3 Perspective of disease diagnosis using machine learning
- 2.4 Conclusions
- References
- Chapter 3. Machine learning for biomedical signal processing
- Abstract
- 3.1 Introduction
- 3.2 Reviews of ECG signal
- 3.3 Preprocessing of ECG signal using ML based techniques
- 3.4 Feature extraction and classification of ECG signal using ML-based techniques
- 3.5 Discussions and conclusions
- References
- Chapter 4. Artificial itelligence in medicine
- Abstract
- 4.1 Introduction
- 4.2 Conclusion
- References
- Chapter 5. Diagnosing of disease using machine learning
- Abstract
- 5.1 Introduction
- 5.2 Background and related work
- 5.3 Types of machine-learning algorithm
- 5.4 Diagnosis model for disease prediction
- 5.5 Confusion matrix
- 5.6 Disease diagnosis by various machine-learning algorithms
- 5.7 ML algorithm in neurological, cardiovascular, and cancer disease diagnosis
- 5.8 Conclusion and future scope
- References
- Chapter 6. A novel approach of telemedicine for managing fetal condition based on machine learning technology from IoT-based wearable medical device
- Abstract
- 6.1 Introduction
- 6.2 Healthcare and big data
- 6.3 Big data analytics
- 6.4 Need of IOT in the healthcare industry
- 6.5 Healthcare uses machine learning
- 6.6 Need for machine learning
- 6.7 Cardiotocography
- 6.8 Literature review
- 6.9 Methodology
- 6.10 Evaluation
- 6.11 Conclusion and future work
- References
- Chapter 7. IoT-based healthcare delivery services to promote transparency and patient satisfaction in a corporate hospital
- Abstract
- 7.1 Introduction
- 7.2 Uses of IoT in healthcare
- 7.3 Main problem area of a corporate hospital
- 7.4 Implementation of IoT-based healthcare delivery services
- 7.5 Conclusion
- References
- Chapter 8. Examining diabetic subjects on their correlation with TTH and CAD: a statistical approach on exploratory results
- Abstract
- 8.1 Introduction
- 8.2 Review of literature
- 8.3 Research methodology
- 8.4 Result analysis and discussion
- 8.5 Originality in the presented work
- 8.6 Future scope and limitations
- 8.7 Recommendations and considerations
- 8.8 Conclusion
- References
- Chapter 9. Cancer prediction and diagnosis hinged on HCML in IOMT environment
- Abstract
- 9.1 Introduction to machine learning (ML)
- 9.2 Introduction to IOT
- 9.3 Application of IOT in healthcare
- 9.4 Machine learning use in health care
- 9.5 Cancer in healthcare
- 9.6 Breast cancer in IoHTML
- 9.7 Case study in breast cancer
- 9.8 Breast cancer algorithm
- 9.9 Conclusion
- References
- Chapter 10. Parameterization techniques for automatic speech recognition system
- Abstract
- 10.1 Introduction
- 10.2 Motivation
- 10.3 Speech production
- 10.4 Data collection
- 10.5 Speech signal processing
- 10.6 Features for speech recognition
- 10.7 Speech parameterization
- 10.8 Speech recognition
- 10.9 Speech classification
- 10.10 Summary and discussion
- Reference
- Chapter 11. Impact of big data in healthcare system—a quick look into electronic health record systems
- Abstract
- 11.1 A leap into the healthcare domain
- 11.2 The real facts of health record collection
- 11.3 A proposal for the future
- 11.4 Discussions and concluding comments on health record collection
- 11.5 Background of electronic health record systems
- 11.6 Review of challenges and study methodologies
- 11.7 Conclusion and discussion
- References
- Index
- Edition: 1
- Published: April 14, 2021
- Imprint: Academic Press
- No. of pages: 290
- Language: English
- Paperback ISBN: 9780128212295
- eBook ISBN: 9780128232170
KS
Krishna Kant Singh
Dr. Krishna Kant Singh, currently the esteemed Director of Delhi Technical Campus in Greater Noida, India, is a highly experienced educator and researcher in the field of engineering and technology. He is a B.Tech and M.Tech degree, a Postgraduate Diploma in Machine Learning and Artificial Intelligence from IIIT Bangalore, a Master of Science in Machine Learning and Artificial Intelligence from Liverpool John Moores University, United Kingdom, and a Ph.D. from IIT Roorkee. Dr. Singh has made significant contributions to the academic and research community. With over 19 years of teaching experience, he has played a vital role in educating and mentoring future professionals. Dr. Singh also serves as an Associate Editor at IEEE Access, an Editorial Board Member at Applied Computing and Geosciences (Elsevier), and a Guest Editor for Complex and Intelligent Systems. His extensive publication record includes over 132 research papers. His areas of interest include Machine Learning, Deep Learning, computer vision and so on.
ME
Mohamed Elhoseny
AS
Akansha Singh
Prof. Akansha Singh, Professor at the School of Computer Science and Engineering, Bennett University, Greater Noida, boasts a comprehensive academic background with a B.Tech, M.Tech, and Ph.D. in Computer Science. Her doctoral studies, conducted at the prestigious IIT Roorkee, were focused on the cutting-edge fields of image processing and machine learning. A prolific author and scholar, Dr. Singh has contributed over 100 research papers and penned more than 25 books. Her editorial expertise is recognized by leading publishers such as Elsevier, Taylor and Francis, and Wiley, where she has edited books on a variety of emerging topics.Dr. Singh serves as the Associate Editor in IEEE Access, Discover Applied Science, PLOS One and guest editor in several journals. Her research interests are diverse and influential, spanning image processing, remote sensing, the Internet of Things (IoT), Blockchain and machine learning. Prof. Singh’s work in these areas not only advances the field of computer science but also significantly contributes to the broader scientific and technological community.
AE