Limited Offer
Save 50% on book bundles
Immediately download your ebook while waiting for your print delivery. No promo code needed.
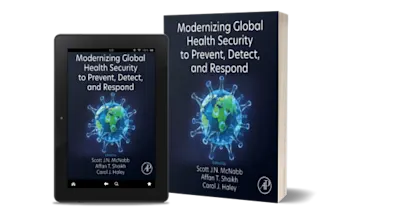
Machine Learning and Pattern Recognition Methods in Chemistry from Multivariate and Data Driven Modeling outlines key knowledge in this area, combining critical introduct… Read more
Limited Offer
Machine Learning and Pattern Recognition Methods in Chemistry from Multivariate and Data Driven Modeling outlines key knowledge in this area, combining critical introductory approaches with the latest advanced techniques. Beginning with an introduction of univariate and multivariate statistical analysis, the book then explores multivariate calibration and validation methods. Soft modeling in chemical data analysis, hyperspectral data analysis, and autoencoder applications in analytical chemistry are then discussed, providing useful examples of the techniques in chemistry applications.
Drawing on the knowledge of a global team of researchers, this book will be a helpful guide for chemists interested in developing their skills in multivariate data and error analysis.
Students, teachers and researchers across chemistry interested in developing their data analysis skills
1. Statistical Methods in Chemical Data Analysis
2. Multivariate Predictive Modeling and Validation
3. Multivariate Pattern Recognition by Machine Learning Methods
4. Tuning the Apparent Thermodynamic Parameters of Chemical Systems
5. The Analytical/Measurement Sources of Multivariate Errors
6. Autoencoders in Analytical Chemistry
7. Uniqueness in Resolving Multivariate Chemical Data
Appendix 1. Introduction to Python
JG