SOCIAL SCIENCES & HUMANITIES
Exploring human experience
Up to 30% off Social Sciences & Humanities titles!
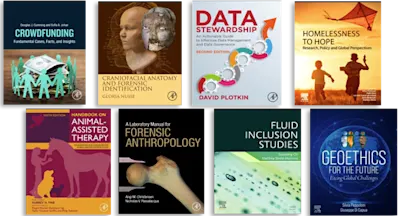
**Selected for 2025 Doody’s Core Titles® in Radiation Oncology**Machine Learning and Artificial Intelligence in Radiation Oncology: A Guide for Clinicians is designed for the appl… Read more
SOCIAL SCIENCES & HUMANITIES
Up to 30% off Social Sciences & Humanities titles!
BR
Dr. Rosenstein is a Professor of Radiation Oncology and a Professor of Genetics & Genomic Sciences at the Icahn School of Medicine at Mount Sinai. The focus of Dr. Rosenstein’s research program for the past 25 years has been the identification of genetic/genomic markers associated with the development of adverse effects resulting from radiotherapy. In this context, he was one of the first investigators to hypothesize that possession of single nucleotide polymorphisms in certain genes may render some cancer patients more susceptible to injuries resulting from radiotherapy. Dr. Rosenstein established and co-led for 14 years the Radiogenomics Consortium (RGC), representing an international consortium currently with 240 members in 33 countries across 135 institutions. Through his efforts, Dr. Rosenstein, has been in the forefront of research in the use of big data in radiation oncology and has collaborated with investigators possessing expertise in bioinformatics and statistics to employ machine learning-based modeling approaches in radiogenomic studies.
TR
Dr. Rattay is Associate Professor in Breast Surgery at the University of Leicester and Consultant Breast Surgeon at University Hospitals of Leicester, UK. He has been working in the field of radiobiology and radiogenomics of the normal tissues for over ten years. His research is specifically focused on the effect of breast radiotherapy on surgical and patient-reported outcomes. This includes Big Data and machine learning (ML) approaches and he is also interested in applying qualitative research methodology to explore breast cancer survivors’ views and experience of treatment and personalised medicine. Dr. Rattay works in a multi-disciplinary research team with nurses, clinical psychologists, geneticists, radiographers and medical physicists, and he has established collaborations with ML experts both nationally and internationally.
JK
Dr. Kang has over 15 years of experience in developing and applying novel computational methods to complex, biomedical data. He is an assistant professor and biomedical informatics lead in the Dept. of Radiation Oncology at the University of Washington. His research focus is on machine learning in oncology with a specific focus on natural language processing and topic modeling and his operations focus is on using informatics to improve patient care and decrease physician burden. Dr. Kang has been invited to speak on AI in oncology at several national and international conferences and workshops.