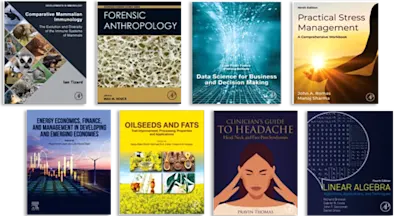
Machine Learning and Artificial Intelligence in Chemical and Biological Sensing
- 1st Edition - July 7, 2024
- Imprint: Elsevier Science
- Editors: Jeong-Yeol Yoon, Chenxu Yu
- Language: English
- Paperback ISBN:9 7 8 - 0 - 4 4 3 - 2 2 0 0 1 - 2
- eBook ISBN:9 7 8 - 0 - 4 4 3 - 2 2 0 0 0 - 5
Machine Learning and Artificial Intelligence in Chemical and Biological Sensing covers the theoretical background and practical applications of various ML/AI methods toward ch… Read more
Purchase options
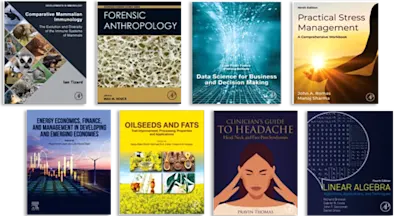
Institutional subscription on ScienceDirect
Request a sales quoteMachine Learning and Artificial Intelligence in Chemical and Biological Sensing covers the theoretical background and practical applications of various ML/AI methods toward chemical and biological sensing. No comprehensive reference text has been available previously to cover the wide breadth of this topic. The book's editors have written the first three chapters to firmly introduce the reader to fundamental ML theories that can be used for chemical/biosensing. Subsequent chapters then cover the practical applications with contributions by various experts in the field.
Sections show how ML and AI-based techniques can provide solutions for: 1) identifying and quantifying target molecules when specific receptors are unavailable 2) analyzing complex mixtures of target molecules, such as gut microbiome and soil microbiome 3) analyzing high-throughput and high-dimensional data, such as drug screening, molecular interaction, and environmental toxicant analysis, 4) analyzing complex data sets where fingerprinting approach is needed This book is written primarily for upper undergraduate students, graduate students, research staff, and faculty members at teaching and research universities and colleges who are working on chemical sensing, biosensing, analytical chemistry, analytical biochemistry, biomedical imaging, medical diagnostics, environmental monitoring, and agricultural applications.
- Presents the first comprehensive reference text on the use of ML and AI for chemical and biological sensing
- Provides a firm grounding in the fundamental theories on ML and AI before covering the practical applications with contributions by various experts in the field
- Includes a wide array of practical applications covered, including: E-nose, Raman, SERS, lens-free imaging, multi/hyperspectral imaging, NIR/optical imaging, receptor-free biosensing, paper microfluidics, single molecule analysis in biomedicine, in situ protein characterization, microbial population dynamics, and all-in-one sensor systems
2. Fundamentals of Machine Learning (ML) and Artificial Intelligence (AI)
3. Use of ML/AI in Chemical Sensors and Biosensors
4. ML-Assisted E-nose and Gas Sensors
5. ML-Assisted FTIR and Raman Spectroscopic Sensing in Agricultural and Food Systems
6. ML-Assisted Surface-Enhanced Raman Spectroscopic Characterization of Biological Systems
7. AI-Assisted Microscopic Imaging Analysis for High Throughput Phenotyping
8. ML-Assisted Lens-Free Imaging
9. ML-Assisted Multispectral and Hyperspectral Imaging
10. AI/ML-Assisted NIR/Optical Biosensing for Plant Phenotyping
11. ML-Assisted Receptor-Free Biosensing
12. ML-Assisted Flow Velocity Analysis in Paper Microfluidics
13. AI/ML Tools for Single Molecule Data Analysis in Biomedicine
14. ML-Assisted Characterization of In Situ Protein Dynamics at Solid-Liquid Interfaces
15. AI-Assisted Microbial Population Dynamics Modeling
16. AI-Assisted All-in-One Sensor System
- Edition: 1
- Published: July 7, 2024
- No. of pages (Paperback): 408
- Imprint: Elsevier Science
- Language: English
- Paperback ISBN: 9780443220012
- eBook ISBN: 9780443220005
JY
Jeong-Yeol Yoon
CY