SUSTAINABLE DEVELOPMENT
Innovate. Sustain. Transform.
Save up to 30% on top Physical Sciences & Engineering titles!
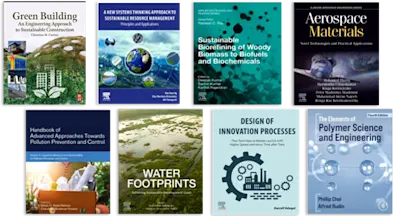
The global race to develop and deploy automated vehicles is still hindered by significant challenges, with the related complexities requiring multidisciplinary research ap… Read more
SUSTAINABLE DEVELOPMENT
Save up to 30% on top Physical Sciences & Engineering titles!
The global race to develop and deploy automated vehicles is still hindered by significant challenges, with the related complexities requiring multidisciplinary research approaches.
Knowledge Graph-Based Methods for Automated Driving offers sought-after, specialized know-how for a wide range of readers both in academia and industry on the use of graphs as knowledge representation techniques which, compared to other relational models, provide a number of advantages for data-driven applications like automated driving tasks. The machine learning pipeline presented in this volume incorporates a variety of auxiliary information, including logic rules, ontology-informed workflows, simulation outcomes, differential equations, and human input, with the resulting operational framework being more reliable, secure, efficient as well as sustainable.
Case studies and other practical discussions exemplify these methods’ promising and exciting prospects for the maturation of scalable solutions with potential to transform transport and logistics worldwide.
RD
MN
Dr. Nalini holds a PhD in Electronics and Communication Engineering from Sri Chandrasekarendra Saraswathi Viswa Maha Vidyalaya, Kanchipuram, India. Her research and publication interests include Artificial Intelligence, biomedical engineering, wireless sensor networks, and Internet of Things. She holds two patents in India and has received a grant to submit another application through the AICTE Quality Improvement Schemes supported by the Gvt. of India.
MS
Dr. Malathy holds a PhD in Information and Communication Engineering from Anna University, Chennai, India. Her research areas include wireless sensor networks, Internet of Things, and applied machine learning. She is a life member of the Indian Society for Technical Education (ISTE) and the International Association of Engineers (IAENG). She is an active author/editor for Springer, CRC Press, and Elsevier. She is also a reviewer for Wireless Networks (Springer) and on the editorial board at many international conferences.
MM