LIMITED OFFER
Save 50% on book bundles
Immediately download your ebook while waiting for your print delivery. No promo code needed.
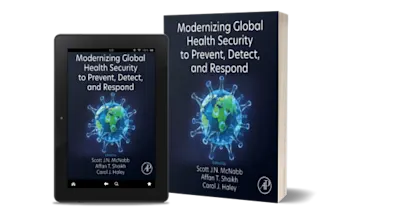
*Textbook and Academic Authors Association (TAA) McGuffey Longevity Award Winner, 2024*A trusted market leader for four decades, Sheldon Ross’s Introduction to Probability M… Read more
LIMITED OFFER
Immediately download your ebook while waiting for your print delivery. No promo code needed.
*Textbook and Academic Authors Association (TAA) McGuffey Longevity Award Winner, 2024*
A trusted market leader for four decades, Sheldon Ross’s Introduction to Probability Models offers a comprehensive foundation of this key subject with applications across engineering, computer science, management science, the physical and social sciences and operations research. Through its hallmark exercises and real examples, this valuable course text
Introduction to Probability Models provides the reader with a comprehensive course in the subject, from foundations to advanced topics.
SR