SUSTAINABLE DEVELOPMENT
Innovate. Sustain. Transform.
Save up to 30% on top Physical Sciences & Engineering titles!
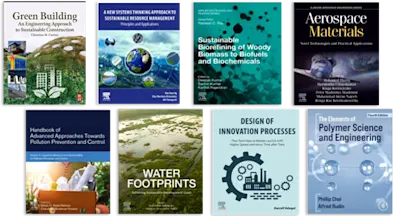
Introduction to Business Analytics Using Simulation, Second Edition employs an innovative strategy to teach business analytics. The book uses simulation modeling and analysis… Read more
SUSTAINABLE DEVELOPMENT
Save up to 30% on top Physical Sciences & Engineering titles!
Introduction to Business Analytics Using Simulation, Second Edition employs an innovative strategy to teach business analytics. The book uses simulation modeling and analysis as mechanisms to introduce and link predictive and prescriptive modeling. Because managers can't fully assess what will happen in the future, but must still make decisions, the book treats uncertainty as an essential element in decision-making. Its use of simulation gives readers a superior way of analyzing past data, understanding an uncertain future, and optimizing results to select the best decision. With its focus on uncertainty and variability, this book provides a comprehensive foundation for business analytics.
Students will gain a better understanding of fundamental statistical concepts that are essential to marketing research, Six-Sigma, financial analysis, and business analytics.
JP