LIMITED OFFER
Save 50% on book bundles
Immediately download your ebook while waiting for your print delivery. No promo code needed.
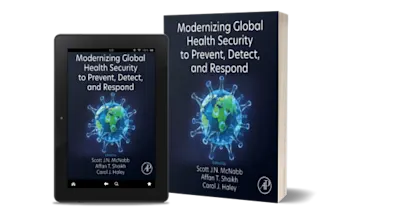
Interpretable Machine Learning for the Analysis, Design, Assessment, and Informed Decision Making for Civil Infrastructure highlights the growing trend of fostering machine l… Read more
LIMITED OFFER
Immediately download your ebook while waiting for your print delivery. No promo code needed.
Interpretable Machine Learning for the Analysis, Design, Assessment, and Informed Decision Making for Civil Infrastructure highlights the growing trend of fostering machine learning to realize contemporary, smart, and safe infrastructure.
This volume delves into the latest advancements in machine learning and artificial intelligence, providing readers with practical insights into their applications in the analysis, design, and assessment of civil infrastructure. From the innovative use of Generative Adversarial Networks in the design of shear wall structures to the application of deep learning for damage inspection of concrete structures, each chapter offers a unique perspective on the integration of cutting-edge technology in the field. Explore the potential of AI-driven fire safety design for smart buildings, the challenges and promises of large-scale evacuation modeling, and the use of machine learning classifiers for evaluating liquefaction potential. The book also features an in-depth discussion on explainable machine learning models for predicting the axial capacity of strengthened CFST columns and the development of spalling detection techniques using deep learning. Whether you are a civil engineer, researcher, or industry professional, this book is an invaluable resource that will equip you with the knowledge and tools to revolutionize civil infrastructure design and management.
This book presents innovative research results supplemented with case studies from leading researchers in this dynamic and emerging field to be used as benchmarks to carry out future experiments and/or facilitate the development of future experiments and advanced numerical models. The book is delivered as a guide for a wide audience, including senior postgraduate students, academic and industrial researchers, materials scientists, and practicing engineers working in civil, environmental, and mechanical engineering.
Academic and industrial researchers, materials scientists and practicing engineers working in civil, structural and mechanical engineering
MN