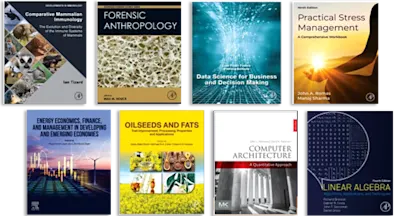
Intelligent Learning Approaches for Renewable and Sustainable Energy
- 1st Edition - February 27, 2024
- Imprint: Elsevier
- Editors: Josep M. Guerrero, Pankaj Gupta, Ritu Kandari, Alexander Micallef
- Language: English
- Paperback ISBN:9 7 8 - 0 - 4 4 3 - 1 5 8 0 6 - 3
- eBook ISBN:9 7 8 - 0 - 4 4 3 - 1 5 8 0 7 - 0
Intelligent Learning Approaches for Renewable and Sustainable Energy provides a practical, systematic overview of the application of advanced intelligent control techniques, adapti… Read more
Purchase options
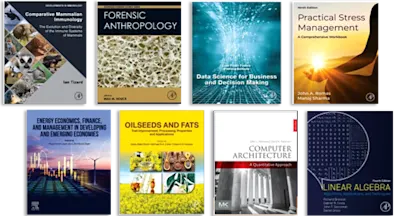
Intelligent Learning Approaches for Renewable and Sustainable Energy provides a practical, systematic overview of the application of advanced intelligent control techniques, adaptive techniques, machine learning algorithms, and predictive control in renewable and sustainable energy. Sections introduce intelligent learning approaches and the roles of artificial intelligence and machine learning in terms of energy and sustainability, grid transformation, large-scale integration of renewable energy, and variability and flexibility of renewable sources. Other sections provide detailed coverage of intelligent learning techniques as applied to key areas of renewable and sustainable energy, including forecasting, supply and demand, integration, energy management, optimization, and more.
This is a useful resource for researchers, scientists, advanced students, energy engineers, R&D professionals, and other industrial personnel with an interest in sustainable energy and integration of renewable energy sources, energy systems, energy engineering, machine learning, and artificial intelligence.
This is a useful resource for researchers, scientists, advanced students, energy engineers, R&D professionals, and other industrial personnel with an interest in sustainable energy and integration of renewable energy sources, energy systems, energy engineering, machine learning, and artificial intelligence.
- Explores cutting-edge intelligent techniques and their implications for future energy systems development
- Opens the door to a range of applications across forecasting, supply and demand, energy management, optimization, and more
- Includes a range of case studies that provide insights into the challenges and solutions in real-world applications
Academic: Researchers, scientists, and advanced students working in sustainable energy and integration of renewable energy sources into utility grids, as well as across energy systems, energy engineering, machine learning, and artificial intelligence. Industry: Energy engineers, R&D professionals, and industry personnel working in the field of renewable and sustainable energy.
Section I: Introduction to intelligent learning approaches for renewable and sustainable energy
1. Artificial Intelligence-based sustainability in energy
2. Machine-learning-based sustainability in energy
3. Transforming the grid: AI, ML, Renewable, Storage, EVs, and Prosumers
4. Role of intelligent techniques in large-scale integration of renewable energy
5. Variability of renewable energy generation and flexibility initiatives
Section II: Applications of intelligence learning approaches for renewable and sustainable energy
6. Intelligent learning models for renewable energy forecasting
7. Intelligent learning models for balancing supply and demand
8. Intelligent learning analysis for a flexibility energy approach towards renewable energy integration
9. Intelligent learning analysis for energy management
10. Intelligent learning approaches for demand-side controller for BIPVs integrated buildings
11. Intelligent learning approaches for single and multi-objective optimization methodology
12. Intelligent learning approaches for optimization of integrated energy systems
1. Artificial Intelligence-based sustainability in energy
2. Machine-learning-based sustainability in energy
3. Transforming the grid: AI, ML, Renewable, Storage, EVs, and Prosumers
4. Role of intelligent techniques in large-scale integration of renewable energy
5. Variability of renewable energy generation and flexibility initiatives
Section II: Applications of intelligence learning approaches for renewable and sustainable energy
6. Intelligent learning models for renewable energy forecasting
7. Intelligent learning models for balancing supply and demand
8. Intelligent learning analysis for a flexibility energy approach towards renewable energy integration
9. Intelligent learning analysis for energy management
10. Intelligent learning approaches for demand-side controller for BIPVs integrated buildings
11. Intelligent learning approaches for single and multi-objective optimization methodology
12. Intelligent learning approaches for optimization of integrated energy systems
- Edition: 1
- Published: February 27, 2024
- Imprint: Elsevier
- Language: English
JG
Josep M. Guerrero
Josep M. Guerrero is a full professor with AAU Energy, Aalborg University, Denmark. He is the director of the Center for Research on Microgrids (CROM). He has published more than 800 journal articles in the fields of microgrids and renewable energy systems, which have been cited more than 80,000 times. His research interests focus on different microgrid aspects, including hierarchical and cooperative control, and energy management systems.
Affiliations and expertise
Full Professor, AAU Energy, Aalborg University and Director of the Center for Research on Microgrids (CROM), DenmarkPG
Pankaj Gupta
Dr. Pankaj Gupta his received his BE degree in Electrical Engineering from BIT, Durg, India, ME degree in Electrical Engineering from Delhi College of Engineering, Delhi University, and his PhD degree in Electrical Engineering from NIT, Kurukshetra, India. He was conferred with the prestigious POSOCO Power System Award 2017 for outstanding PhD research work on protection issues of grid connected distributed generation by the Power System Operation Corporation Ltd., a subsidiary of Power Grid Corporation, India, in partnership with the Foundation for Innovation and Technology Transfer, IIT, Delhi. Currently, Dr. Gupta is working with Indira Gandhi Delhi Technical University for Women, Delhi, India as Assistant Professor. His research interests include power system protection, microgrid control and protection, smart grid technologies, and islanding detection techniques.
Affiliations and expertise
Assistant Professor, Indira Gandhi Delhi Technical University for Women, Delhi, IndiaRK
Ritu Kandari
Ritu Kandari received her B.Tech. Degree in Electrical and Electronics from Guru Gobind Singh Indraprastha University, India, in 2010, and M.Tech. Degree in Digital Communication from Ambedkar Institute of Advanced Communication Technologies and Research, Delhi, in 2012. She joined HMR Institute of Technology and Management, Delhi as an assistant professor in 2012. She is currently pursuing her PhD. Degree in renewable energy at the Department of Electronics and Communication Engineering, IGDTUW, Delhi. Kandari has co-edited 3 books with Elsevier and was also a guest associate editor of the special issue on electricity islands for the journal Renewable & Sustainable Energy Reviews.
Affiliations and expertise
Senior Research Fellow (SRF) in Renewable Energy, IGDTUW, Delhi, IndiaAM
Alexander Micallef
Dr. Alexander Micallef is a Senior Lecturer with the Department of Industrial Electrical Power Conversion at the University of Malta, Malta. He has also been a guest lecturer for the PhD course on “Power Quality and Synchronization Techniques in Microgrids” at Aalborg University since 2015. Dr. Micallef received the B.Eng. (Hons.), M. Sc. in Engineering, and PhD degrees from the University of Malta (Malta) in 2006, 2009 and 2015 respectively. He has authored or co-authored more than 20 journal and conference papers on microgrids, ship electrification, zero energy buildings, and power quality. Dr. Micallef is an Associate Editor for IEEE Access and the IET Smart Grids journal. He is a Senior Member of IEEE and is a member of the IEEE Standards Association, as well as vice-chair of the IEEE Malta Section and previously Chair of the Smart Buildings and Customer Systems Subcommittee within the IEEE PES SBLCS Technical Committee.
Affiliations and expertise
Senior Lecturer, Department of Industrial Electrical Power Conversion, University of Malta, MaltaRead Intelligent Learning Approaches for Renewable and Sustainable Energy on ScienceDirect