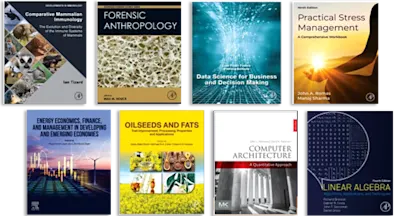
Intelligent Biomedical Technologies and Applications for Healthcare 5.0
- 1st Edition, Volume 16 - October 22, 2024
- Imprint: Academic Press
- Editors: Lalit Garg, Gayatri Mirajkar, Sanjay Misra, Vijay Kumar Chattu
- Language: English
- Paperback ISBN:9 7 8 - 0 - 4 4 3 - 2 2 0 3 8 - 8
- eBook ISBN:9 7 8 - 0 - 4 4 3 - 2 2 0 3 9 - 5
Intelligent Biomedical Technologies and Applications for Healthcare 5.0, Volume Sixteen covers artificial health intelligence, biomedical image analysis, 5G, the Internet of Medica… Read more
Purchase options
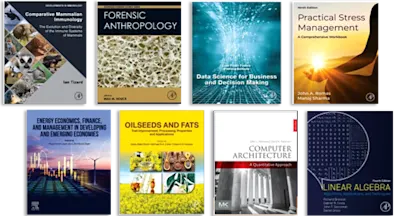
Intelligent Biomedical Technologies and Applications for Healthcare 5.0, Volume Sixteen covers artificial health intelligence, biomedical image analysis, 5G, the Internet of Medical Things, intelligent healthcare systems, and extended health intelligence (EHI). This volume contains four sections. The focus of the first section is health data analytics and applications. The second section covers research on information exchange and knowledge sharing. The third section is on the Internet of Things (IoT) and the Internet of Everything (IoE)-based solutions. The final section focuses on the implementation, assessment, adoption, and management of healthcare informatics solutions.
This new volume in the Advances in Ubiquitous Sensing Applications for Healthcare series focuses on innovative methods in the healthcare industry and will be useful for biomedical engineers, researchers, and students working in interdisciplinary fields of research. This volume bridges these newly developing technologies and the medical community in the rapidly developing healthcare world, introducing them to modern healthcare advances such as EHI and Smart Healthcare Systems.
- Provides a comprehensive technological review of cutting-edge information in the wide domain of Healthcare 5.0
- Introduces concepts that combine computational methods, network standards, and healthcare systems to provide a much improved, more affordable experience delivered by healthcare services to its customers
- Presents innovative solutions utilizing informatics to deal with various healthcare technology issues
2. A cost-effective way of implementing Big data in Health Research in developing countries – case of Malawi
3. Characterizing Hospital Admission Patterns and Length of Stay in the Emergency Department at Mater Dei Hospital Malta
4. Machine Learning Approach for Enhancement in Healthcare
5. Disease Detection and Treatment Methods
6. Hybrid Fuzzy Based Improved Particle Swarm Optimization Technique for Cancer Cell Detection
7. A review on Covid19 (SARS-CoV-2) Pandemic: Using Artificial Intelligence and Machine Learning Protocols
8. Validity, Reliability and Usability Assessment of Smartphone-based Health 5.0 Application for Measuring Chronic Low Back Pain
9. Healthcare 5.0 Opportunities and Challenges: A Literature Review
10. An Impact of Reliability in Healthcare Internet of Things (HIoT)
11. Investigating the Effect of a Software Intervention Based on a Theoretical Behaviour Framework to Encourage Ergonomic Compliance During Computing Device Usage
12. Machine Learning Approach for Post-COVID Disease Prediction
13. Improving Interoperability Between Health Information Technology Systems Used by Mental Health and Acute Hospitals
14. Data management in the healthcare sector - A review
15. Emerging biomedical technologies in healthcare
16. Healthcare Cyber Risk and its Impact on Healthcare
17. Machine and Deep learning techniques for Smart Healthcare Industry: Big-Picture, and Open Research Challenges
18. Different Machine Learning Algorithms for Precise Brain Stroke Prediction
- Edition: 1
- Volume: 16
- Published: October 22, 2024
- Imprint: Academic Press
- Language: English
LG
Lalit Garg
GM
Gayatri Mirajkar
SM
Sanjay Misra
VC
Vijay Kumar Chattu
Dr Vijay Kumar Chattu is a Global Health Specialist at the University of Toronto and an Adjunct Professor at the School of Computer Science Engineering (SCOPE), Vellore Institute of Technology. Besides, he is an Adjunct Professor at the University of Alberta, a Senior Fellow at the WHO CC for KT and HTA in Health Equity at Ottawa and a Visiting Research Fellow at United Nations University-CRIS, Belgium. His interdisciplinary research intersects Medicine, Health Sciences, Health Technology, Health Policy and International Relations. During his academic career, Dr Chattu studied at various universities in 5 continents and travelled to over 45 countries. He did his MBBS and MD from India, MPH from Belgium, MPhil from South Africa, Fellowship in Digital Health/Occupational Medicine from Canada and PhD from Trinidad and Tobago. Besides, he also holds a Post Master’s Certificate in Mental Health from Harvard University and a Graduate Certificate in Global Health Diplomacy from Graduate Institute Geneva. He is a recipient of the NIH-Fogarty AITRP Grant at UCLA, the Fogarty MHIRT Grant at the U of Michigan and numerous prestigious awards from DGDC Belgium, Salzburg Global Seminar Austria and WHO-IARC France, to name a few. He published over 400 articles and has consistently ranked among the World’s Top 2% Scientists in Public Health by the Stanford University & Elsevier Rankings since 2021. Dr Chattu serves as an Associate Editor for BMJ Global Health and other prestigious journals. As a Global Health Policy advisor, he regularly contributes to the T20 Policy briefs for G20 Summits and G7 Forums. He has a long track record of consulting for multilateral organizations such as UNAIDS-TSF, WHO and the World Bank. Some of his co-authored works include applications of Blockchain technology, Artificial Intelligence, Machine Learning, and Deep Learning in health domains such as Disease Surveillance, Maternal and Child Health, Health Equity, Sleep Medicine etc. His current research interests include AI-driven diagnostics, Telemedicine, Remote patient consultations in the Metaverse, and Virtual Reality applications in medical education and training. Dr Chattu is also the Founder and CEO of Global Health Research and Innovations Canada Inc. (GHRIC) based in Toronto.