Integrative Omics
Concept, Methodology, and Application
- 1st Edition - May 3, 2024
- Editors: Manish Kumar Gupta, Pramod Katara, Sukanta Mondal, Ram Lakhan Singh
- Language: English
- Paperback ISBN:9 7 8 - 0 - 4 4 3 - 1 6 0 9 2 - 9
- eBook ISBN:9 7 8 - 0 - 4 4 3 - 1 6 0 9 3 - 6
Integrative Omics: Concept, Methodology and Application provides a holistic and integrated view of defining and applying network approaches, integrative tools, and methods t… Read more
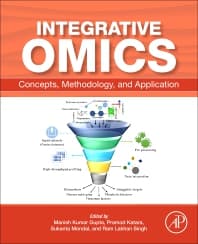
Purchase options
Institutional subscription on ScienceDirect
Request a sales quoteIntegrative Omics: Concept, Methodology and Application provides a holistic and integrated view of defining and applying network approaches, integrative tools, and methods to solve problems for the rationalization of genotype to phenotype relationships. The reference provides systemic ‘step-by-step’ coverage that begins with basic concepts from Omic to Multi Integrative Omics approaches followed by applications and emerging and future trends. All areas of Omics are covered, including biological databases, sequence alignment, pharmacogenomics, nutrigenomics and microbial omics, integrated omics for Food Science and Identification of genes associated with disease, clinical data integration and data warehousing, translational omics, technology policy, and society research. This book covers recent concepts, methodologies, advancements in technologies and is also well-suited for researchers from both academic and industry background, undergraduate and graduate students who are mainly working in the area of computational systems biology, integrative omics and translational science.
- Provides a holistic, integrated view of a defining and applying network approach, integrative tools, and methods to solve problems for rationalization of genotype to phenotype relationships
- Offers an interdisciplinary approach to Databases, data analytics techniques, biological tools, network construction, analysis, modeling, prediction and simulation of biological systems leading to ‘translational research’, i.e., drug discovery, drug target prediction, and precision medicine
- Covers worldwide methods, concepts, databases, and tools used in the construction of integrated pathways
Students, researchers, and academicians in bioinformatics. Government/non-government agencies and professionals working in Bioinformatics, Computational Biology and Data Science, Drug Discovery and IT industry
- From Omics to Multi-integrative Omics Approach
- Types Of Omics Data: Genomics, Metagenomics, Epigenomics, Transcriptomics, Proteomics, Metabolomics and Phenomics
- Biological Omics databases and Tools
- Systematic Benchmarking of Omics Computational Tools
- Pharmacogenomics, Nutrigenomics, and Microbial Omics
- Proteomics: Present and Future Prospectives
- Foodomics: Integrated Omics for the Food and Nutrition Science
- Vaccinomics
- Integrative Omics Approach for Identification of Genes Associated with Disease
- Integrative Omics Approaches for Identification of Biomarkers
- Omics Approach for Personalized and Diagnostics Medicine
- Role of Bioinformatics in Genome Analysis
- Data Management in Cross Omics
- Omics and Clinical Data Integration and Data Warehousing
- Integrative Omics Data Mining: Challenges and Opportunities
- Data Science and Analytics, Modeling, Simulation, and Issues of Omics Data Set
- Emerging Trends in Translational Omics
- Omics Technology for Crop Improvement
- Ecology and Environmental Omics
- Current Trends and Approaches in Clinical Metagenomics
- Bio-molecular Networks
- Machine Learning Fundamentals to Explore Complex OMICS Data
- Omics Technology Policy and Society Research
- No. of pages: 430
- Language: English
- Edition: 1
- Published: May 3, 2024
- Imprint: Academic Press
- Paperback ISBN: 9780443160929
- eBook ISBN: 9780443160936
MG
Manish Kumar Gupta
PK
Pramod Katara
SM
Sukanta Mondal
RS