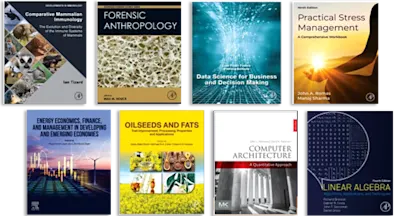
Innovative Data Integration and Conceptual Space Modeling for COVID, Cancer, and Cardiac Care
- 1st Edition - January 18, 2022
- Imprint: Academic Press
- Authors: Amy Neustein, Nathaniel Christen
- Language: English
- Paperback ISBN:9 7 8 - 0 - 3 2 3 - 8 5 1 9 7 - 8
- eBook ISBN:9 7 8 - 0 - 3 2 3 - 8 5 3 5 6 - 9
In recent years, scientific research and translation medicine have placed increased emphasis on computational methodology and data curation across many disciplines, both to ad… Read more
Purchase options
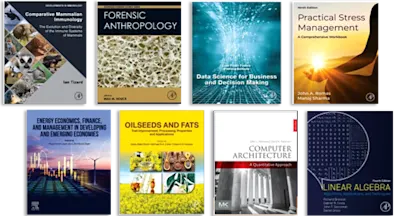
In recent years, scientific research and translation medicine have placed increased emphasis on computational methodology and data curation across many disciplines, both to advance underlying science and to instantiate precision-medicine protocols in the lab and in clinical practice. The nexus of concerns related to oncology, cardiology, and virology (SARS-CoV-2) presents a fortuitous context within which to examine the theory and practice of biomedical data curation.
Innovative Data Integration and Conceptual Space Modeling for COVID, Cancer, and Cardiac Care argues that a well-rounded approach to data modeling should optimally embrace multiple perspectives inasmuch as data-modeling is neither a purely formal nor a purely conceptual discipline, but rather a hybrid of both. On the one hand, data models are designed for use by computer software components, and are, consequently, constrained by the mechanistic demands of software environments; data modeling strategies must accept the formal rigors imposed by unambiguous data-sharing and query-evaluation logic. In particular, data models are not well-suited for software-level deployment if such models do not translate seamlessly to clear strategies for querying data and ensuring data integrity as information is moved across multiple points. On the other hand, data modeling is, likewise, constrained by human conceptual tendencies, because the information which is managed by databases and data networks is ultimately intended to be visualized/utilized by humans as the end-user.
Thus, at the intersection of both formal and humanistic methodology, data modeling takes on elements of both logico-mathematical frameworks (e.g., type systems and graph theory) and conceptual/philosophical paradigms (e.g., linguistics and cognitive science). The authors embrace this two-sided aspect of data models by seeking non-reductionistic points of convergence between formal and humanistic/conceptual viewpoints, and by leveraging biomedical contexts (viz., COVID, Cancer, and Cardiac Care) so as to provide motivating examples and case-studies in this volume.
- Provides an analysis of how conceptual spaces and related cognitive linguistic approaches can inspire programming and query-processing models
- Outlines the vital role that data modeling/curation has played in significant medical breakthroughs
- Presents readers with an overview of how information-management approaches intersect with precision medicine, providing case studies of data-modeling in concrete scientific practice
- Explores applications of image analysis and computer vision in the context of precision medicine
- Examines the role of technology in scientific publishing, replication studies, and dataset curation
Academics (scientists, researchers, MSc, and/or PhD students) from the fields of Biomedical Engineering, Computer Science, Biology, and Information Technology. The audience also includes epidemiologists, computational epidemiologists, software engineers, biostatisticians, and data scientists.
1. Introduction
Part I: Biomedical data formats and data integration
2. Data structures associated with biomedical research
3. Data mining and predictive analytics for cancer and COVID-19
4. Modular design, image biomarkers, and radiomics
Part II: Type theory, graphs, and conceptual spaces
5. Types' internal structure and “non-constructive (“NC4”) type theory
6. Using code models to instantiate data models
Part III: Conceptual spaces and graph-oriented data-modeling paradigms
7. Multi-aspect modules and image annotation
8. Image annotation as a multi-aspect case study
9. Conceptual spaces and scientific data models
- Edition: 1
- Published: January 18, 2022
- Imprint: Academic Press
- Language: English
AN
Amy Neustein
NC