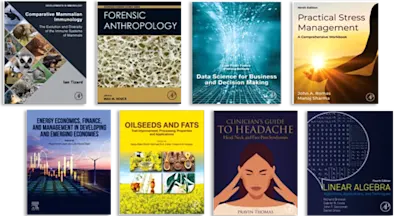
Hyperspectral Imaging
- 1st Edition, Volume 32 - September 29, 2019
- Imprint: Elsevier
- Editor: Jose Manuel Amigo
- Language: English
- Paperback ISBN:9 7 8 - 0 - 4 4 4 - 6 3 9 7 7 - 6
- eBook ISBN:9 7 8 - 0 - 4 4 4 - 6 3 9 7 8 - 3
Hyperspectral Imaging, Volume 32, presents a comprehensive exploration of the different analytical methodologies applied on hyperspectral imaging and a state-of-the-art analysis… Read more
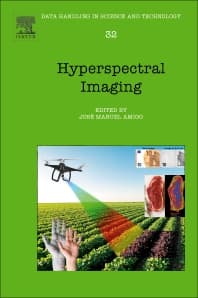
Purchase options
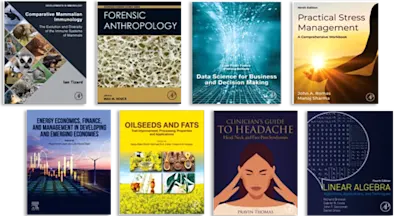
Institutional subscription on ScienceDirect
Request a sales quoteHyperspectral Imaging, Volume 32, presents a comprehensive exploration of the different analytical methodologies applied on hyperspectral imaging and a state-of-the-art analysis of applications in different scientific and industrial areas. This book presents, for the first time, a comprehensive collection of the main multivariate algorithms used for hyperspectral image analysis in different fields of application. The benefits, drawbacks and suitability of each are fully discussed, along with examples of their application. Users will find state-of-the art information on the machinery for hyperspectral image acquisition, along with a critical assessment of the usage of hyperspectral imaging in diverse scientific fields.
- Provides a comprehensive roadmap of hyperspectral image analysis, with benefits and considerations for each method discussed
- Covers state-of-the-art applications in different scientific fields
- Discusses the implementation of hyperspectral devices in different environments
Scientists, academics, and graduate students in various disciplines working with hyperspectral images, including remote sensing, vegetation and crops, food and feed production, forensic sciences, biochemistry, medical imaging, pharmaceutical production, and art studies
- Cover image
- Title page
- Table of Contents
- Copyright
- Contributors
- Author Biography
- Preface
- Personal Thinking and Acknowledgments
- List of Figures
- List of Tables
- Introduction
- Chapter 1.1. Hyperspectral and multispectral imaging: setting the scene
- 1. Images: basic concepts, spatial resolution, and spectral information
- 2. Data mining: chemometrics
- 3. Growing the number of application fields of HSI and MSI
- Chapter 1.2. Configuration of hyperspectral and multispectral imaging systems
- 1. Introduction
- 2. Light–matter interaction
- 3. Acquisition modes
- 4. Light source
- 5. Wavelength dispersion devices
- 6. Detectors
- 7. Calibration
- 8. Conclusions
- Algorithms and methods
- Chapter 2.1. Preprocessing of hyperspectral and multispectral images
- 1. Why preprocessing?
- 2. Geometric corrections of distortions coming from the instrument
- 3. Dead pixels, spikes, and missing scan lines
- 4. Spectral preprocessing
- 5. Background removal
- 6. Some advices on preprocessing
- Chapter 2.2. Hyperspectral compression
- 1. Introduction
- 2. Lossless approaches
- 3. Lossy approaches
- 4. Conclusions
- Chapter 2.3. Pansharpening
- 1. Introduction
- 2. Classification of pansharpening methods
- 3. Quality assessment of pansharpening products
- 4. Sharpening of hyperspectral data
- 5. Concluding remarks
- Chapter 2.4. Unsupervised exploration of hyperspectral and multispectral images
- 1. Unsupervised exploration methods
- 2. Projection methods: Principal component analysis
- 3. Clustering
- 4. Final observations
- Chapter 2.5. Multivariate curve resolution for hyperspectral image analysis
- 1. Hyperspectral images: Structures and related models
- 2. Multivariate curve resolution: The direct link with the measurement model
- 3. Multivariate curve resolution-alternating least squares
- 4. Image fusion (or multiset analysis)
- 5. Use of resolved maps and spectral signatures: going beyond MCR
- 6. Conclusions
- Chapter 2.6. Nonlinear spectral unmixing
- 1. Introduction
- 2. Multilayered model
- 3. Intimate mixtures model
- 4. Examples
- 5. Conclusions
- Chapter 2.7. Variability of the endmembers in spectral unmixing
- 1. Introduction
- 2. Reminder on linear spectral unmixing
- 3. Endmember variability in remote sensing
- 4. Handling variability in the spatial domain
- 5. Summary and future research avenues
- Chapter 2.8. An overview of regression methods in hyperspectral and multispectral imaging
- 1. Introduction
- 2. Brief theory of most popular regression methods
- 3. Considerations to apply regression methods in HSI and MSI
- 4. Applications
- 5. Conclusions
- Chapter 2.9. Classical least squares for detection and classification
- 1. Introduction
- 2. Classical least squares models
- 3. Characterization of the measured signal
- 4. Example applications
- 5. Conclusions
- Chapter 2.10. Supervised classification methods in hyperspectral imaging—recent advances
- 1. Hyperspectral imaging and the importance of contextual information
- 2. Ensemble strategies for image classification
- 3. Methods for the inclusion of contextual analysis
- 4. Conclusion
- Chapter 2.11. Fusion of hyperspectral imaging and LiDAR for forest monitoring
- 1. Introduction
- 2. Motivation
- 3. Schemes of fusion
- 4. Applications
- 5. Datasets
- Chapter 2.12. Hyperspectral time series analysis: hyperspectral image data streams interpreted by modeling known and unknown variations
- 1. Introduction
- 2. Methods
- 3. Results
- 4. Overall model assessments
- 5. Conclusions
- Chapter 2.13. Statistical biophysical parameter retrieval and emulation with Gaussian processes
- 1. Introduction
- 2. Gaussian process models for inverse modeling
- 3. Advanced Gaussian processes for inverse modeling
- 4. Emulation and optimization of radiative transfer models with Gaussian processes
- 5. Conclusions
- Application fields
- Chapter 3.1. Applications in remote sensing—natural landscapes
- 1. Introduction
- 2. Planetary sciences
- 3. Coastal areas
- 4. Cryosphere
- 5. Vegetation
- 6. Discussion and conclusion
- Chapter 3.2. Applications in remote sensing—anthropogenic activities
- 1. Introduction
- 2. Urban areas
- 3. Soils
- 4. Atmosphere
- 5. Precision agriculture
- 6. Conclusion
- Chapter 3.3. Hyperspectral imaging in crop fields: precision agriculture
- 1. Crop fields, precision agriculture, and chemometrics. A general overview
- 2. Detection of contaminants
- 3. Water stress and health status
- 4. Crop diseases
- 5. Pre-harvest applications
- 6. Conclusions and further challenges
- Chapter 3.4. Food and feed production
- 1. Introduction
- 2. Prediction of quality properties
- 3. Classification by variety or species or origin
- 4. Determination of industrial properties
- 5. Detection of physical defects or damage
- 6. Detection of biological damage and contamination
- 7. Detection of adulterations and frauds
- 8. Detection of allergens
- 9. Conclusions
- Chapter 3.5. Hyperspectral imaging for food-related microbiology applications
- 1. Introduction
- 2. Hyperspectral imaging
- 3. Chapter organization
- 4. Visible-near-infrared applications
- 5. Near-InfraRed
- 6. Raman
- 7. Conclusions
- Chapter 3.6. Hyperspectral imaging in medical applications
- 1. Introduction
- 2. Tissue optics
- 3. Hardware and systems
- 4. Image analysis
- 5. Medical applications
- 6. Discussions
- 7. Conclusion
- Chapter 3.7. Hyperspectral imaging as a part of pharmaceutical product design
- 1. Background
- 2. RGB imaging
- 3. NIR and IR imaging
- 4. Raman imaging
- 5. UV imaging
- 6. Challenges and future needs in the pharmaceutical field
- Chapter 3.8. Hyperspectral imaging for artworks investigation
- 1. Imaging spectroscopy for cultural heritage applications: a brief historical overview
- 2. Hyperspectral imaging for investigations of artworks: basics
- 3. Instrumentations, setups, and specific issues
- 4. Approaches to data processing and data handling
- 5. An example of application: noninvasive examination of a renaissance panel painting
- 6. Summary
- Chapter 3.9. Growing applications of hyperspectral and multispectral imaging
- 1. Forensics
- 2. Waste sorting
- 3. Archaeology
- 4. Entomology
- 5. Conclusions and perspectives
- Author Index
- Subject Index
- Edition: 1
- Volume: 32
- Published: September 29, 2019
- No. of pages (Paperback): 800
- No. of pages (eBook): 800
- Imprint: Elsevier
- Language: English
- Paperback ISBN: 9780444639776
- eBook ISBN: 9780444639783
JA