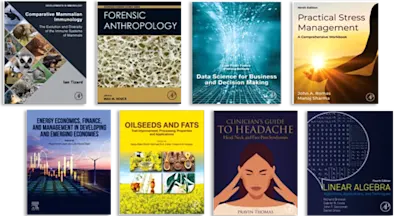
Hidden Semi-Markov Models
Theory, Algorithms and Applications
- 1st Edition - October 22, 2015
- Imprint: Elsevier
- Author: Shun-Zheng Yu
- Language: English
- Paperback ISBN:9 7 8 - 0 - 1 2 - 8 0 2 7 6 7 - 7
- eBook ISBN:9 7 8 - 0 - 1 2 - 8 0 2 7 7 1 - 4
Hidden semi-Markov models (HSMMs) are among the most important models in the area of artificial intelligence / machine learning. Since the first HSMM was introduced in 1980 for ma… Read more
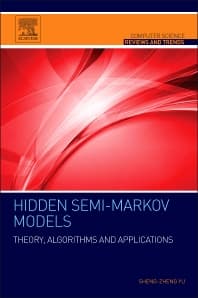
Purchase options
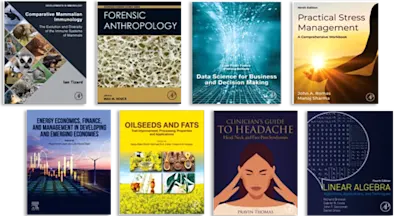
Institutional subscription on ScienceDirect
Request a sales quoteHidden semi-Markov models (HSMMs) are among the most important models in the area of artificial intelligence / machine learning. Since the first HSMM was introduced in 1980 for machine recognition of speech, three other HSMMs have been proposed, with various definitions of duration and observation distributions. Those models have different expressions, algorithms, computational complexities, and applicable areas, without explicitly interchangeable forms.
Hidden Semi-Markov Models: Theory, Algorithms and Applications provides a unified and foundational approach to HSMMs, including various HSMMs (such as the explicit duration, variable transition, and residential time of HSMMs), inference and estimation algorithms, implementation methods and application instances. Learn new developments and state-of-the-art emerging topics as they relate to HSMMs, presented with examples drawn from medicine, engineering and computer science.
- Discusses the latest developments and emerging topics in the field of HSMMs
- Includes a description of applications in various areas including, Human Activity Recognition, Handwriting Recognition, Network Traffic Characterization and Anomaly Detection, and Functional MRI Brain Mapping.
- Shows how to master the basic techniques needed for using HSMMs and how to apply them.
- Preface
- Acknowledgments
- Chapter 1. Introduction
- Abstract
- 1.1 Markov Renewal Process and Semi-Markov Process
- 1.2 Hidden Markov Models
- 1.3 Dynamic Bayesian Networks
- 1.4 Conditional Random Fields
- 1.5 Hidden Semi-Markov Models
- 1.6 History of Hidden Semi-Markov Models
- Chapter 2. General Hidden Semi-Markov Model
- Abstract
- 2.1 A General Definition of HSMM
- 2.2 Forward–Backward Algorithm for HSMM
- 2.3 Matrix Expression of the Forward–Backward Algorithm
- 2.4 Forward-Only Algorithm for HSMM
- 2.5 Viterbi Algorithm for HSMM
- 2.6 Constrained-Path Algorithm for HSMM
- Chapter 3. Parameter Estimation of General HSMM
- Abstract
- 3.1 EM Algorithm and Maximum-Likelihood Estimation
- 3.2 Re-estimation Algorithms of Model Parameters
- 3.3 Order Estimation of HSMM
- 3.4 Online Update of Model Parameters
- Chapter 4. Implementation of HSMM Algorithms
- Abstract
- 4.1 Heuristic Scaling
- 4.2 Posterior Notation
- 4.3 Logarithmic Form
- 4.4 Practical Issues in Implementation
- Chapter 5. Conventional HSMMs
- Abstract
- 5.1 Explicit Duration HSMM
- 5.2 Variable Transition HSMM
- 5.3 Variable-Transition and Explicit-Duration Combined HSMM
- 5.4 Residual Time HSMM
- Chapter 6. Various Duration Distributions
- Abstract
- 6.1 Exponential Family Distribution of Duration
- 6.2 Discrete Coxian Distribution of Duration
- 6.3 Duration Distributions for Viterbi HSMM Algorithms
- Chapter 7. Various Observation Distributions
- Abstract
- 7.1 Typical Parametric Distributions of Observations
- 7.2 A Mixture of Distributions of Observations
- 7.3 Multispace Probability Distributions
- 7.4 Segmental Model
- 7.5 Event Sequence Model
- Chapter 8. Variants of HSMMs
- Abstract
- 8.1 Switching HSMM
- 8.2 Adaptive Factor HSMM
- 8.3 Context-Dependent HSMM
- 8.4 Multichannel HSMM
- 8.5 Signal Model of HSMM
- 8.6 Infinite HSMM and HDP-HSMM
- 8.7 HSMM Versus HMM
- Chapter 9. Applications of HSMMs
- Abstract
- 9.1 Speech Synthesis
- 9.2 Human Activity Recognition
- 9.3 Network Traffic Characterization and Anomaly Detection
- 9.4 fMRI/EEG/ECG Signal Analysis
- References
- Edition: 1
- Published: October 22, 2015
- Imprint: Elsevier
- No. of pages: 208
- Language: English
- Paperback ISBN: 9780128027677
- eBook ISBN: 9780128027714
SY