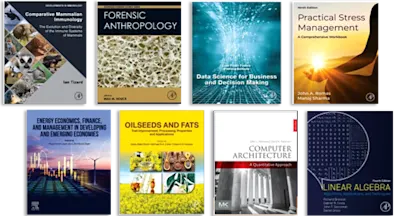
Harnessing Automation and Machine Learning for Resource Recovery and Value Creation
From Waste to Value
- 1st Edition - March 31, 2025
- Imprint: Elsevier
- Editors: Kishor Kumar Sadasivuni, Nebojsa Bacanin, Jaehwan Kim, Neha B Vashisht
- Language: English
- Paperback ISBN:9 7 8 - 0 - 4 4 3 - 2 7 3 7 4 - 2
- eBook ISBN:9 7 8 - 0 - 4 4 3 - 2 7 3 7 5 - 9
Harnessing Automation and Machine Learning for Resource Recovery and Value Creation: From Waste to Value provides a comprehensive understanding of how automation and machine learni… Read more
Purchase options
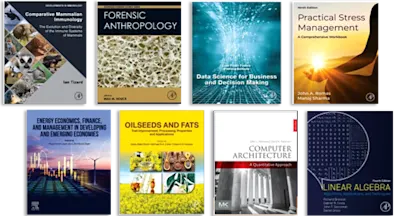
- Provides insights into the potential of automation and machine learning in waste management inspiring readers to adopt sustainable waste management practices
- Offers a comprehensive understanding of how waste management can be transformed into a profitable business by adopting innovative and sustainable solutions
- Offers an opportunity to explore case studies from different industries and regions to showcase the revolutionary applications of automation and machine learning in waste management
- Provides guidance for waste management professionals, policymakers, and business leaders to optimize waste management processes and improve their bottom line
2. Basics of Machine learning
3. Basics of Automation
4. Waste classification into plastics, industrial, domestic, and agriculture waste
5. Plastics recycling and the automation role in the recycling process
6. Artificial intelligence for reutilizing the Plastics
7. Handling Metals waste to Salvage with Automation
8. Machine learning: A better means for Metal waste to reprocess
9. Automation in the recycling of Industrial waste
10. Machine learning in Industrial Waste to value added
11. Waste to Value Added: Role of automation in Organic waste
12. Impact of Artificial Intelligence for the recycling of Organic waste
13. Future of Agriculture: Automated vertical farming
14. Agriculture: The Next Machine-Learning Frontier
15. Recycling robots to tackle electrical waste
16. Machine learning for sustainable development in electronics
17. Automated Sorting of Recyclable Domestic waste
18. Machine learning for recycling domestic waste
19. Future aspects of Machine learning/automation for the waste management
- Edition: 1
- Published: March 31, 2025
- Imprint: Elsevier
- Language: English
KS
Kishor Kumar Sadasivuni
Dr. Kishor Kumar Sadasivuni is an Assistant Professor at the Center for Advanced Materials, Qatar University, with expertise in polymer composite materials and high-performance polymer nanocomposites for industrial applications. Since 2009, he has promoted interdisciplinary collaborations in nanocomposites and industrial technologies. His research includes sensor technology, piezoelectrics, energy storage, and flexible electronics. He’s a very prolific author and his work has contributed to advancements in materials science and electronics, which have earned him the recognition of being ranked among the top researchers in the AD Scientific Index 2022.
NB
Nebojsa Bacanin
Dr. Nebojsa Bacanin received his Ph.D. degrees from Faculty of Mathematics, University of Belgrade in 2015 (study program Computer Science, average grade 10,00). He was the vice-dean of the Graduate School of Computer Science and Faculity of Informatics and Computing in Belgrade, Serbia. He currently works as a Full Professor and as a Vice-Rector for Scientific Research at Singidunum University. He is involved in scientific research in the field of computer science and his specialty includes artificial intelligence, machine learning, deep learning, stochastic optimization algorithms, swarm intelligence, soft-computing, optimization and modeling, image processing, computer vision and cloud and distributed computing. He actively works in the domain of novel and prospective research field, hybrid methods between machine learning and metaheuristics, where metaheuristics are applied for addressing non-deterministic polynomial hard (NP-hard) challenges from machine learning domain such as hyper-parameters optimization (tuning), training and feature selection. Besides improving machine learning/deep learning models for tackling various practical tasks for classification and regression, his research also involves optimized deep learning models for univariate and multivariate time-series forecasting. Moreover, he is an expert from the area of metaheuristics, and he has been actively doing research in enhancing swarm intelligence, as well as other types of metaheuristics, by incorporating minor changes (e.g., modification in exploitation/exploration expressions, parameters’ adjustments, etc.) and/or major modifications by performing hybridization with other methods (e.g., low-level and high-level hybrid metaheuristics methods). He has been applying his methods to wide variety of practical research areas, e.g., cloud computing scheduling, wireless sensor networks (WSNs) localization, coverage and energy consumption, X-ray images classification, stock price forecasting, portfolio optimization, as well as many others.
JK
Jaehwan Kim
NV