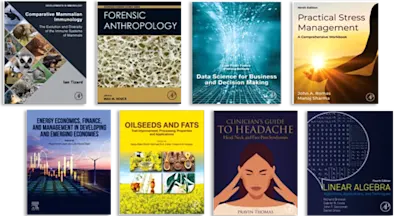
Hardware Accelerator Systems for Artificial Intelligence and Machine Learning
- 1st Edition, Volume 122 - March 28, 2021
- Imprint: Academic Press
- Editors: Shiho Kim, Ganesh Chandra Deka
- Language: English
- Hardback ISBN:9 7 8 - 0 - 1 2 - 8 2 3 1 2 3 - 4
- eBook ISBN:9 7 8 - 0 - 1 2 - 8 2 3 1 2 4 - 1
Hardware Accelerator Systems for Artificial Intelligence and Machine Learning, Volume 122 delves into artificial Intelligence and the growth it has seen with the advent of Deep N… Read more
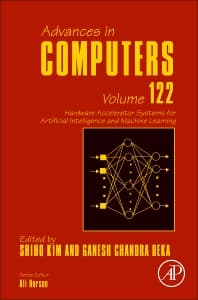
Purchase options
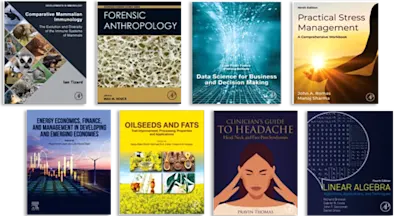
Institutional subscription on ScienceDirect
Request a sales quoteHardware Accelerator Systems for Artificial Intelligence and Machine Learning, Volume 122 delves into artificial Intelligence and the growth it has seen with the advent of Deep Neural Networks (DNNs) and Machine Learning. Updates in this release include chapters on Hardware accelerator systems for artificial intelligence and machine learning, Introduction to Hardware Accelerator Systems for Artificial Intelligence and Machine Learning, Deep Learning with GPUs, Edge Computing Optimization of Deep Learning Models for Specialized Tensor Processing Architectures, Architecture of NPU for DNN, Hardware Architecture for Convolutional Neural Network for Image Processing, FPGA based Neural Network Accelerators, and much more.
- Updates on new information on the architecture of GPU, NPU and DNN
- Discusses In-memory computing, Machine intelligence and Quantum computing
- Includes sections on Hardware Accelerator Systems to improve processing efficiency and performance
Final year Undergraduate student for Project on Embedded system, Master’s and PhD Scholars
- Cover image
- Title page
- Table of Contents
- Copyright
- Contributors
- Preface
- Chapter One: Introduction to hardware accelerator systems for artificial intelligence and machine learning
- Abstract
- 1: Introduction to artificial intelligence and machine learning in hardware acceleration
- 2: Deep learning and neural network acceleration
- 3: HW accelerators for artificial neural networks and machine learning
- 4: SW framework for deep neural networks
- 5: Comparison of FPGA, CPU and GPU
- 6: Conclusion and future scope
- Chapter Two: Hardware accelerator systems for embedded systems
- Abstract
- 1: Introduction
- 2: Neural network computing in embedded systems
- 3: Hardware acceleration in embedded systems
- 4: Software frameworks for neural networks
- Chapter Three: Hardware accelerator systems for artificial intelligence and machine learning
- Abstract
- 1: Introduction
- 2: Background
- 3: Hardware inference accelerators for deep neural networks
- 4: Hardware inference accelerators using digital neurons
- 5: Summary
- Acknowledgments
- Chapter Four: Generic quantum hardware accelerators for conventional systems
- Abstract
- 1: Introduction
- 2: Principles of computation
- 3: Need and foundation for quantum hardware accelerator design
- 4: A generic quantum hardware accelerator (GQHA)
- 5: Industrially available quantum hardware accelerators
- 6: Conclusion and future work
- Chapter Five: FPGA based neural network accelerators
- Abstract
- 1: Introduction
- 2: Background
- 3: Algorithmic optimization
- 4: Accelerator architecture
- 5: Design methodology
- 6: Applications
- 7: Evaluation
- 8: Future research directions
- Chapter Six: Deep learning with GPUs
- Abstract
- 1: Deep learning applications using GPU as accelerator
- 2: Overview of graphics processing unit
- 3: Deep learning acceleration in GPU hardware perspective
- 4: GPU software for accelerating deep learning
- 5: Advanced techniques for optimizing deep learning models on GPUs
- 6: Cons and pros of GPU accelerators
- Acknowledgment
- Chapter Seven: Architecture of neural processing unit for deep neural networks
- Abstract
- 1: Introduction
- 2: Background
- 3: Considerations in hardware design
- 4: NPU architectures
- 5: Discussion
- 6: Summary
- Acknowledgments
- References for advance
- Chapter Eight: Energy-efficient deep learning inference on edge devices
- Abstract
- 1: Introduction
- 2: Theoretical background
- 3: Deep learning frameworks and libraries
- 4: Advantages of deep learning on the edge
- 5: Applications of deep learning at the edge
- 6: Hardware support for deep learning inference at the edge
- 7: Static optimizations for deep learning inference at the edge
- 8: Dynamic (input-dependent) optimizations for deep learning inference at the edge
- 9: Open challenges and future directions
- Chapter Nine: “Last mile” optimization of edge computing ecosystem with deep learning models and specialized tensor processing architectures
- Abstract
- 1: Introduction
- 2: State of the art
- 3: Methodology
- 4: Results
- 5: Discussion
- 6: Conclusions
- Acknowledgments
- Chapter Ten: Hardware accelerator for training with integer backpropagation and probabilistic weight update
- Abstract
- 1: Introduction
- 2: Integer back propagation with probabilistic weight update
- 3: Consideration of hardware implementation of the probabilistic weight update
- 4: Simulation results of the proposed scheme
- 5: Discussions
- 6: Summary
- Acknowledgments
- Chapter Eleven: Music recommender system using restricted Boltzmann machine with implicit feedback
- Abstract
- 1: Introduction
- 2: Types of recommender systems
- 3: Problem statement
- 4: Explanation of RBM
- 5: Proposed architecture
- 6: Minibatch size used for training and selection of weights and biases
- 7: Types of activation function that can be used in this model
- 8: Evaluation metrics that can be used to measure for music recommendation
- 9: Experimental setup
- 10: Result
- 11: Conclusion
- 12: Future works
- Edition: 1
- Volume: 122
- Published: March 28, 2021
- Imprint: Academic Press
- No. of pages: 416
- Language: English
- Hardback ISBN: 9780128231234
- eBook ISBN: 9780128231241
SK
Shiho Kim
Shiho Kim is a professor in the school of integrated technology at Yonsei University, Seoul, Korea. His previous assignment includes, System on chip design engineer, at LG Semicon Ltd. (currently SK Hynix), Korea, Seoul [1995-1996], Director of RAVERS (Research center for Advanced Hybrid Electric Vehicle Energy Recovery System, a government-supported IT research center. Associate Director of the ICT consilience program, which is a Korea National program for cultivating talented engineers in the field of information and communication Technology, Korea [2011-2012], Director of Seamless Transportation Lab, at Yonsei university, Korea [since 2011-]. His main research interest includes Development of software and hardware technologies for intelligent vehicles, Blockchain technology for intelligent transportation systems, and reinforcement learning for autonomous vehicles. He is the member of the editorial board and reviewer for various Journals and International conferences. So far he has organized 2 International Conference as Technical Chair/General Chair. He is a member of IEIE (Institute of Electronics and Information Engineers of Korea), KSAE (Korean Society of Automotive Engineers), vice president of KINGC (Korean Institute of Next Generation Computing), and a senior member of IEEE. He is the co-author for over 100 papers and holding more than 50 patents in the area of information and communication technology.
Affiliations and expertise
School of Integrated Technology, Yonsei University, Seoul, KoreaGD
Ganesh Chandra Deka
Ganesh Chandra Deka is currently Deputy Director (Training) at Directorate General of Training, Ministry of Skill Development and Entrepreneurship, Government of India, New Delhi-110001, India. His research interests include e-Governance, Big Data Analytics, NoSQL Databases and Vocational Education and Training.
He has 2 books on Cloud Computing published by LAP Lambert, Germany. He is the Co-author for 4 text books on Fundamentals of Computer Science (3 books published by Moni Manik Prakashan, Guwahati, Assam, India and 1 IGI Global, USA). As of now he has edited 14 books (6 IGI Global, USA, 5 CRC Press, USA, 2 Elsevier & 1 Springer) on Big data, NoSQL and Cloud Computing and authored 10 Book Chapters.
He has published around 47 research papers in various IEEE conferences. He has organized 08 IEEE International Conferences as Technical Chair in India. He is the Member of the editorial board and reviewer for various Journals and International conferences. Member of IEEE, the Institution of Electronics and Telecommunication Engineers, India and Associate Member, the Institution of Engineers, India
Affiliations and expertise
Ministry of Skill Development and Entrepreneurship, New Delhi, IndiaRead Hardware Accelerator Systems for Artificial Intelligence and Machine Learning on ScienceDirect