LIMITED OFFER
Save 50% on book bundles
Immediately download your ebook while waiting for your print delivery. No promo code needed.
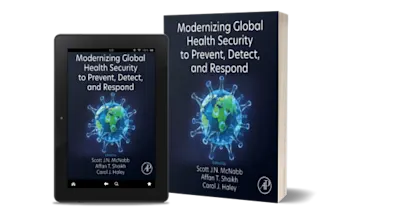
Handbook of Mobility Data Mining, Volume One: Data Preprocessing and Visualization introduces the fundamental technologies of mobile big data mining (MDM), advanced AI methods,… Read more
LIMITED OFFER
Immediately download your ebook while waiting for your print delivery. No promo code needed.
Handbook of Mobility Data Mining, Volume One: Data Preprocessing and Visualization introduces the fundamental technologies of mobile big data mining (MDM), advanced AI methods, and upper-level applications, helping readers comprehensively understand MDM with a bottom-up approach. The book explains how to preprocess mobile big data, visualize urban mobility, simulate and predict human travel behavior, and assess urban mobility characteristics and their matching performance as conditions and constraints in transport, emergency management, and sustainability development systems. The book contains crucial information for researchers, engineers, operators, administrators, and policymakers seeking greater understanding of current technologies' infra-knowledge structure and limitations.
Further, the book introduces how to design MDM platforms that adapt to the evolving mobility environment, new types of transportation, and users based on an integrated solution that utilizes sensing and communication capabilities to tackle significant challenges faced by the MDM field. This volume focuses on how to efficiently pre-process mobile big data to extract and utilize critical feature information of high-dimensional city people flow. The book first provides a conceptual theory and framework, then discusses data sources, trajectory map-matching, noise filtering, trajectory data segmentation, data quality assessment, and more, concluding with a chapter on privacy protection in mobile big data mining.
1. Mobility Data Preprocessing and Visualization: Concept, Theory, and Framework
2. Mobility Data Sources
2.1 GPS Mobility Data Mining
2.2 CDR Mobility Data Mining
2.3 Sensor-based Mobility Data Analytics
3. Trajectory Map-Matching
3.1 Geometric Map-matching Algorithm
3.2 Topological Algorithm
3.3 Probabilistic Algorithm
3.4 Machine Learning- based Map-matching Algorithm
4. Noise Filtering of Mobility Data
4.1 Cluster-based Filter Method
4.2 Kalman Filter Method
4.3 Heuristic-based Outlier Detection Method
5. Trajectory Data Segmentation
5.1 Attribute-Driven Segmentation
5.2 Pattern-Driven Segmentation
6. Stop-Move Detection of Trajectorty Data
6.1 Cluster-based Detection
6.2 Physical Model-based Detection
6.3 Heuristic-based Detection
6.4 Machine Learning-based Detection
7. Travel Mode Detection of Trajectorty Data
7.1 Physical Model-based Detection
7.2 Machine Learning-based Detection
7.3 Deep Learning-based Detection
8. Mobility Data Quality Assessment
8.1 Six Dimensions in Data Quality
8.2 Monte Carlo Data Grading Framework
9. Modifiable Areal Unit Problem
9.1 Mobility Data Aggregation
9.2 Error Analysis
9.3 Grid Shape Impacts
10. Mobility Data Management and Visualization
10.1 Mobility Data Management Tools
10.2 Key Visualization Technologies
10.3 Monitoring
10.4 Analysis and Optimization
10.5 Evaluation strategies involved in evaluating visual interfaces
10.6 Privacy Protection in Mobile Big Data Mining
HZ