LIMITED OFFER
Save 50% on book bundles
Immediately download your ebook while waiting for your print delivery. No promo code needed.
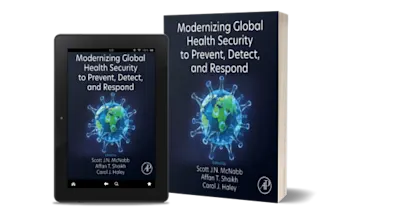
Handbook of Diffusion MR Tractography: Imaging Methods, Biophysical Models, Algorithms and Applications presents methods and applications of MR diffusion tractography, providing… Read more
LIMITED OFFER
Immediately download your ebook while waiting for your print delivery. No promo code needed.
Handbook of Diffusion MR Tractography: Imaging Methods, Biophysical Models, Algorithms and Applications presents methods and applications of MR diffusion tractography, providing deep insights into the theory and implementation of existing tractography techniques and offering practical advice on how to apply diffusion tractography to research projects and clinical applications. Starting from the design of MR acquisition protocols optimized for tractography, the book follows a pipeline approach to explain the main methods behind diffusion modeling and tractography, including advanced analysis of tractography data and connectomics.
An extensive section of the book is devoted to the description of tractography applications in research and clinical settings to give a complete picture of tractography practice today. By focusing on technology, models, and applications, this handbook will be an indispensable reference for researchers and students with backgrounds in computer science, mathematics, physics, neuroscience, and medical science.
FD
Flavio Dell'Acqua is Associate Professor and Reader in Translational Neuroimaging at the Institute of Psychiatry, Psychology and Neuroscience, King's College London, UK. A biomedical engineer and neuroscientist, Dr. Dell'Acqua's research interests span MR physics and medical image analysis. His work focuses on developing and applying advanced diffusion imaging and tractography methods for neuroscience, psychiatric, and clinical research. Dr. Dell'Acqua has co-authored over 100 papers, and his methods have been successfully applied in numerous tractography studies published in high-impact journals including Science, Nature Neuroscience, Nature Communication, Brain, and PNAS. He is a co-founder of the NatBrainLab, a multidisciplinary laboratory dedicated to the study of human neuroanatomy and tractography research. Committed to education in the field, Dr. Dell'Acqua has led educational courses on Diffusion Imaging for the Organization for Human Brain Mapping (OHBM) for multiple years. He is an active member of the International Society for Magnetic Resonance in Medicine (ISMRM) where he has lectured in educational courses, workshops and has served on the Diffusion Study Group committee. In 2023, he was a co-founder of the International Society for Tractography (IST).
MD
AL