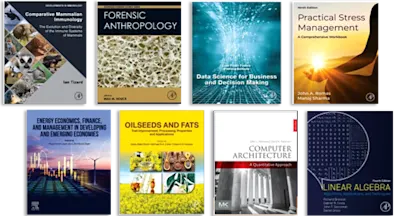
Handbook of Deep Learning in Biomedical Engineering
Techniques and Applications
- 1st Edition - November 12, 2020
- Imprint: Academic Press
- Editors: Valentina Emilia Balas, Brojo Kishore Mishra, Raghvendra Kumar
- Language: English
- Paperback ISBN:9 7 8 - 0 - 1 2 - 8 2 3 0 1 4 - 5
- eBook ISBN:9 7 8 - 0 - 1 2 - 8 2 3 0 4 7 - 3
Deep Learning (DL) is a method of machine learning, running over Artificial Neural Networks, that uses multiple layers to extract high-level features from large amounts of raw… Read more
Purchase options
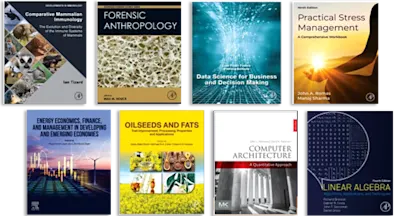
Deep Learning (DL) is a method of machine learning, running over Artificial Neural Networks, that uses multiple layers to extract high-level features from large amounts of raw data. Deep Learning methods apply levels of learning to transform input data into more abstract and composite information. Handbook for Deep Learning in Biomedical Engineering: Techniques and Applications gives readers a complete overview of the essential concepts of Deep Learning and its applications in the field of Biomedical Engineering. Deep learning has been rapidly developed in recent years, in terms of both methodological constructs and practical applications. Deep Learning provides computational models of multiple processing layers to learn and represent data with higher levels of abstraction. It is able to implicitly capture intricate structures of large-scale data and is ideally suited to many of the hardware architectures that are currently available. The ever-expanding amount of data that can be gathered through biomedical and clinical information sensing devices necessitates the development of machine learning and AI techniques such as Deep Learning and Convolutional Neural Networks to process and evaluate the data. Some examples of biomedical and clinical sensing devices that use Deep Learning include: Computed Tomography (CT), Magnetic Resonance Imaging (MRI), Ultrasound, Single Photon Emission Computed Tomography (SPECT), Positron Emission Tomography (PET), Magnetic Particle Imaging, EE/MEG, Optical Microscopy and Tomography, Photoacoustic Tomography, Electron Tomography, and Atomic Force Microscopy. Handbook for Deep Learning in Biomedical Engineering: Techniques and Applications provides the most complete coverage of Deep Learning applications in biomedical engineering available, including detailed real-world applications in areas such as computational neuroscience, neuroimaging, data fusion, medical image processing, neurological disorder diagnosis for diseases such as Alzheimer’s, ADHD, and ASD, tumor prediction, as well as translational multimodal imaging analysis.
- Presents a comprehensive handbook of the biomedical engineering applications of DL, including computational neuroscience, neuroimaging, time series data such as MRI, functional MRI, CT, EEG, MEG, and data fusion of biomedical imaging data from disparate sources, such as X-Ray/CT
- Helps readers understand key concepts in DL applications for biomedical engineering and health care, including manifold learning, classification, clustering, and regression in neuroimaging data analysis
- Provides readers with key DL development techniques such as creation of algorithms and application of DL through artificial neural networks and convolutional neural networks
- Includes coverage of key application areas of DL such as early diagnosis of specific diseases such as Alzheimer’s, ADHD, and ASD, and tumor prediction through MRI and translational multimodality imaging and biomedical applications such as detection, diagnostic analysis, quantitative measurements, and image guidance of ultrasonography
Graduates, PhD students and lecturers in computer science, biomedical engineering and electrical engineering, as well as scientific researchers in biomedical fields and clinicians
1. Application of deep learning in biomedical engineering
2. Applications, algorithms, tools directly related to deep learning
3. Computational Neuroscience; Neuroimaging and Time Series data (including MRI/fMRI/CT, EEG/MEG, etc.) studies;
4. Data Fusion for HealthCare, especially Biomedical images of different nature (X-ray, CT, etc.);
5. Deep neural network in medical image processing (RTG, USG, CT, PET, OCT and others)
6. Early diagnosis of specific diseases like Alzheimer, ADHD, ASD etc
7. Manifold learning, classification, clustering and regression in Neuroimaging data analysis;
8. Multimodal imaging techniques: data acquisition, reconstruction; 2D, 3D, 4D imaging, etc.)
9. Optimization by deep neural networks, Multi-dimensional deep learning
10. Prediction of tumor from MRI using deep learning
11. Theoretical understanding of deep learning in biomedical engineering
12. Translational multimodality imaging and biomedical applications (e.g., detection, diagnostic analysis, quantitative measurements, image guidance of ultrasonography)
2. Applications, algorithms, tools directly related to deep learning
3. Computational Neuroscience; Neuroimaging and Time Series data (including MRI/fMRI/CT, EEG/MEG, etc.) studies;
4. Data Fusion for HealthCare, especially Biomedical images of different nature (X-ray, CT, etc.);
5. Deep neural network in medical image processing (RTG, USG, CT, PET, OCT and others)
6. Early diagnosis of specific diseases like Alzheimer, ADHD, ASD etc
7. Manifold learning, classification, clustering and regression in Neuroimaging data analysis;
8. Multimodal imaging techniques: data acquisition, reconstruction; 2D, 3D, 4D imaging, etc.)
9. Optimization by deep neural networks, Multi-dimensional deep learning
10. Prediction of tumor from MRI using deep learning
11. Theoretical understanding of deep learning in biomedical engineering
12. Translational multimodality imaging and biomedical applications (e.g., detection, diagnostic analysis, quantitative measurements, image guidance of ultrasonography)
- Edition: 1
- Published: November 12, 2020
- Imprint: Academic Press
- Language: English
VE
Valentina Emilia Balas
Valentina Emilia Balas is currently a Full Professor in the Department of Automatics and Applied Software at the Faculty of Engineering, “Aurel Vlaicu” University of Arad, Romania. She holds a PhD cum Laude in Applied Electronics and Telecommunications from the Polytechnic University of Timisoara. Dr. Balas is the author of more than 350 research papers. She is the Editor-in-Chief of the 'International Journal of Advanced Intelligence Paradigms' and the 'International Journal of Computational Systems Engineering', an editorial board member for several other national and international publications, and an expert evaluator for national and international projects and PhD theses.
Affiliations and expertise
Full Professor, Department of Automatics and Applied Software, Faculty of Engineering, "Aurel Vlaicu" University of Arad, Arad, RomaniaBM
Brojo Kishore Mishra
Dr. Brojo Kishore Mishra is currently working as a Professor in the Department of Computer Science and Engineering at the GIET University, Gunupur-765022, India. He received his PhD degree in Computer Science from the Berhampur University in 2012. He has published more than 30 research papers in national and international conference proceedings, 25 research papers in peer-reviewed journals, and 22 book chapters; authored 2 books; and edited 4 books. His research interests include data mining, machine learning, soft computing, and security. He has organized and co organized local and international conferences and also edited several special issues for journals. He is the Senior Member of IEEE and Life Member of CSI, ISTE. He is the Editor of CSI Journal of Computing.
Affiliations and expertise
Professor, Department of CSE, School of Engineering and Technology, GIET University, IndiaRK
Raghvendra Kumar
Raghvendra Kumar is working as an Associate Professor in Computer Science and Engineering Department at GIET University, India. He received BTech, MTech, and PhD in Computer Science and Engineering, India, and Postdoc Fellow from the Institute of Information Technology, Virtual Reality and Multimedia, Vietnam. He has published a number of research papers in international journals and conferences. His research areas are computer networks, data mining, cloud computing, and secure multiparty computations, theory of computer science, and design of algorithms. He authored and edited 23 computer science books in field of IoT, data mining, and biomedical engineering.
Affiliations and expertise
Associate Professor, Department of Computer Science and Engineering, GIET University, IndiaRead Handbook of Deep Learning in Biomedical Engineering on ScienceDirect