Hamiltonian Monte Carlo Methods in Machine Learning
- 1st Edition - February 3, 2023
- Authors: Tshilidzi Marwala, Rendani Mbuvha, Wilson Tsakane Mongwe
- Language: English
- Paperback ISBN:9 7 8 - 0 - 4 4 3 - 1 9 0 3 5 - 3
- eBook ISBN:9 7 8 - 0 - 4 4 3 - 1 9 0 3 6 - 0
Hamiltonian Monte Carlo Methods in Machine Learning introduces methods for optimal tuning of HMC parameters, along with an introduction of Shadow and Non-canonical HMC methods w… Read more
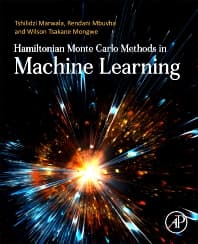
Purchase options
Institutional subscription on ScienceDirect
Request a sales quoteHamiltonian Monte Carlo Methods in Machine Learning introduces methods for optimal tuning of HMC parameters, along with an introduction of Shadow and Non-canonical HMC methods with improvements and speedup. Lastly, the authors address the critical issues of variance reduction for parameter estimates of numerous HMC based samplers. The book offers a comprehensive introduction to Hamiltonian Monte Carlo methods and provides a cutting-edge exposition of the current pathologies of HMC-based methods in both tuning, scaling and sampling complex real-world posteriors. These are mainly in the scaling of inference (e.g., Deep Neural Networks), tuning of performance-sensitive sampling parameters and high sample autocorrelation.
Other sections provide numerous solutions to potential pitfalls, presenting advanced HMC methods with applications in renewable energy, finance and image classification for biomedical applications. Readers will get acquainted with both HMC sampling theory and algorithm implementation.
- Provides in-depth analysis for conducting optimal tuning of Hamiltonian Monte Carlo (HMC) parameters
- Presents readers with an introduction and improvements on Shadow HMC methods as well as non-canonical HMC methods
- Demonstrates how to perform variance reduction for numerous HMC-based samplers
- Includes source code from applications and algorithms
1. Introduction to Hamiltonian Monte Carlo
2. Sampling Benchmarks and Performance Metrics
3. Stochastic Volatility Metropolis-Hastings
4. Quantum-Inspired Magnetic Hamiltonian Monte Carlo
5. Generalised Magnetic and Shadow Hamiltonian Monte Carlo
6. Shadow Hamiltonian Monte Carlo Methods
7. Adaptive Shadow Hamiltonian Monte Carlo Methods
8. Adaptive Noncanonical Hamiltonian Monte Carlo
9. Antithetic Hamiltonian Monte Carlo Techniques
10. Application: Bayesian Neural Network Inference in Wind Speed Forecasting
11. Application: A Bayesian Analysis of Lockdown Alert Level Framework for Combating COVID-19
12. Application: Probabilistic Inference of Equity Option Prices Under Jump-Di
13. Application: Bayesian Inference of Local Government Audit Outcomes
14. Open Problems in Sampling
Appendix
A: Separable Shadow Hamiltonian
B: Automatic Relevance Determination
C: Audit Outcome Literature Survey
- No. of pages: 220
- Language: English
- Edition: 1
- Published: February 3, 2023
- Imprint: Academic Press
- Paperback ISBN: 9780443190353
- eBook ISBN: 9780443190360
TM
Tshilidzi Marwala
RM
Rendani Mbuvha
WM