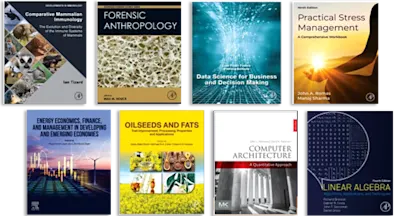
Green Machine Learning and Big Data for Smart Grids
Practices and Applications
- 1st Edition - November 13, 2024
- Imprint: Elsevier
- Editors: V. Indragandhi, R. Elakkiya, V. Subramaniyaswamy
- Language: English
- Paperback ISBN:9 7 8 - 0 - 4 4 3 - 2 8 9 5 1 - 4
- eBook ISBN:9 7 8 - 0 - 4 4 3 - 2 8 9 5 2 - 1
Green Machine Learning and Big Data for Smart Grids: Practices and Applications is a guidebook to the best practices and potential for green data analytics when generating innova… Read more
Purchase options
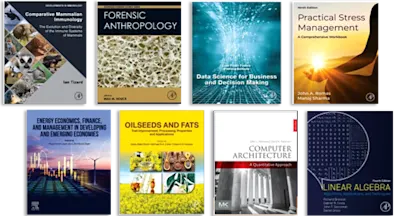
Uses for control of physical components including inverters and converters are examined, along with policy implications. Importantly, real-world case studies and chapter objectives are combined to signpost essential information, and to support understanding and implementation.
- Packages core concepts of green machine learning and smart grids in a clear, understandable way
- Includes real-world, practical applications and case studies for replication and innovative solution development
- Introduces readers with a range of expertise to best practices and the latest technological advances
2. Characteristics and Essential Technologies of Green Machine Learning in the Energy Sector
3. Smart Grid Stability Prediction through Big Data Analytics
4. Descriptive, Predictive, Prescriptive and Diagnostic Analytical Models for Managing Power Systems
5. Integrating Green Machine Learning and Big Data Framework for Renewable Energy Grids
6. Green Machine Learning with Big Data for Grid Operations
7. Big Data Green Machine Learning for Smart Metering
8. Analysis and Real-time Implementation of Power Line Disturbances Test in Smart Grids
9. Analysis and Implementation of Power Optimizer Using Sliding Mode Control enabled String Inverter for Renewable Applications
10. Smart Edge Devices for Electric Grid Computing
11. Combined Flyback Converter and Forward Converter Based Active Cell Balancing in Lithium-Ion Battery Cell for Smart Electric Vehicle Application
12. Predictive Modelling in Asset and Workforce Management
13. Sustainability Consideration of Smart Grid with Big Data Analytics in Social, Economic, Technical and Policy Aspects
14. Real-Time of Big Data and Analytics in Smart Grid and Energy Management Applications
15. Challenges and Future Directions
- Edition: 1
- Published: November 13, 2024
- Imprint: Elsevier
- Language: English
VI
V. Indragandhi
RE
R. Elakkiya
Dr. R. Elakkiya is an Assistant Professor in the Department of Computer Science, Birla Institute of Technology & Science, Pilani, Dubai Campus. She received her PhD from Anna University, Chennai, in 2018. She secured the University First Rank and was awarded the Gold Medal during master’s in software engineering from CEG Campus, Anna University, Chennai. She won the iDEX - DISC 4 challenge and received the grant award from DIO, DRDO in 2021 and Young Achiever Award from INSc in 2019. She had received many extra-mural funded projects from various government and non-government agencies and served as Machine Learning and Data Analytics Consultant and delivered many products to different industry verticals. She is Member of the Association of Computing Machinery and Lifetime Member of International Association of Engineers.
VS
V. Subramaniyaswamy
Dr V. Subramaniyaswamy is currently working as a Professor in the School of Computer Science and Engineering, Vellore Institute of Technology, Vellore, India. In total, he has 18 years of experience in academia. He has published papers in reputed international journals and conferences and filed multiple patents. His technical competencies lie in recommender systems, Artificial Intelligence, the Internet of Things, reinforcement learning, big data analytics, and cognitive analytics. He has edited Electric Motor Drives and their Applications, with Simulation Practice (Elsevier: 2022, ISBN: 9780323911627), among other books.