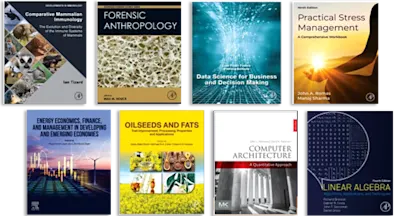
Genetic Optimization Techniques for Sizing and Management of Modern Power Systems
- 1st Edition - September 29, 2022
- Imprint: Elsevier
- Authors: Juan Miguel Lujano Rojas, Rodolfo Dufo Lopez, Jose Antonio Dominguez Navarro
- Language: English
- Paperback ISBN:9 7 8 - 0 - 1 2 - 8 2 3 8 8 9 - 9
- eBook ISBN:9 7 8 - 0 - 1 2 - 8 2 4 2 0 6 - 3
Genetic Optimization Techniques for Sizing and Management of Modern Power Systems explores the design and management of energy systems using a genetic algorithm as the primar… Read more
Purchase options
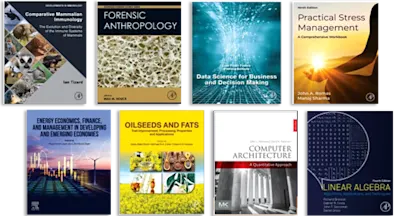
- Presents a range of essential techniques for using genetic algorithms in power system analysis, including
economic dispatch, forecasting, and optimal power fl ow, among other topics. - Addresses relevant optimization problems, such as neural network training and clustering analysis, using
genetic algorithms. - Discusses clearly and straightforwardly the implementation of genetic algorithms and its combination with
other heuristic techniques. - Describes the iHOGA® and MHOGA® commercial tools, which utilize genetic algorithms for designing
and managing energy systems based on renewable energies.
1. Introduction to Optimization techniques for sizing and management of integrated power systems
1.1. A General Overview of This Book
1.2. This Book and Its Target Audience
2. Genetic Algorithms and Other Heuristic Techniques in power systems optimization
2.1. General Perspective and State-of-the-Art of Genetic Algorithms
2.1.1 Mono-Objective Optimization
2.1.2. Multi-Objective Optimization
2.2. General Perspective and State-of-the-Art of Parallel Genetic Algorithms
2.3. Other Heuristic Techniques and Their Combination with Genetic Algorithms
3. Estimation of Natural Resources for Renewable Energy Systems
3.1. State-of-the-Art of Renewable Resource Databases
3.2. Estimating the Typical Meteorological Year of Wind Speed
3.3. Estimating the Typical Meteorological Year of Solar Irradiation
3.4. Estimating the Typical Meteorological Year of Ambient Temperature
4. Renewable Generation and Energy Storage Systems
4.1. State-of-the-Art of Renewable Generation Model and Battery Energy Storage Devices
4.2. Simulation Models of Wind Generation
4.3. Simulation Models of Solar Photovoltaic Generation
4.4. Battery Energy Storage System
4.4.1. Simulation Models of Lead-Acid Batteries
4.4.2. Simulation Models of Vanadium Redox Flow Batteries
4.4.3. Simulation Models of Lithium-Ion Batteries
4.5. Distribution System Model
4.5.1. Deterministic Power Flow Calculation
4.5.2. Probabilistic Power Flow Calculation
5. Forecasting of Electricity Prices, Demand, and Renewable Resources
5.1. State-of-the-Art of Time Series based Forecasting for Renewable Energy Systems
5.2. Electricity Price Forecasting
5.3. Electricity Demand Forecasting
5.4. Forecasting of Environmental Variables
6. Optimization of Renewable Energy Systems by Genetic Algorithms
6.1. Maximum Power Point Tracking of a Photovoltaic Generator
6.2. Rural Electrification and Vulnerability Assessment and Its Mitigation
6.3. Vulnerability Assessment of Energy Systems and Its Mitigation
6.3.1 Mono-Objective Optimization (Net Present Cost)
6.3.2. Multi-Objective Optimization (Greenhouse Gas Emissions)
6.4. Day-Ahead Battery Energy Storage System Operation
6.5. Integration of Distributed Photovoltaic Generation
6.6. Local Electricity Markets
6.7. Residential Demand Response under Real-Time Pricing
6.8. Electric Vehicle Charging Station
7. Creating Energy Systems Policy using genetic optimization techniques
7.1. State-of-the-Art of Computational Tools for Energy System Analysis
7.2. Large-Scale Electrification in Developing Countries
7.3. Massive Integration of Distributed generation in Developed Countries
- Edition: 1
- Published: September 29, 2022
- Imprint: Elsevier
- Language: English
JR
Juan Miguel Lujano Rojas
RL
Rodolfo Dufo Lopez
JN