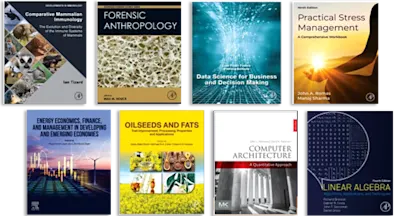
Fundamentals of Uncertainty Quantification for Engineers
Methods and Models
- 1st Edition - May 5, 2025
- Imprint: Elsevier
- Authors: Yan Wang, Anh.V. Tran, David L. Mcdowell
- Language: English
- Paperback ISBN:9 7 8 - 0 - 4 4 3 - 1 3 6 6 1 - 0
- eBook ISBN:9 7 8 - 0 - 4 4 3 - 1 3 6 6 2 - 7
Fundamentals of Uncertainty Quantification for Engineers: Methods and Models provides a comprehensive introduction to uncertainty quantification (UQ) accompanied by a wide va… Read more
Purchase options
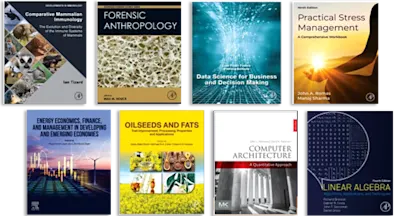
Fundamentals of Uncertainty Quantification for Engineers: Methods and Models provides a comprehensive introduction to uncertainty quantification (UQ) accompanied by a wide variety of applied examples and implementation details to reinforce the concepts outlined in the book. Sections start with an introduction to the history of probability theory and an overview of recent developments of UQ methods in the domains of applied mathematics and data science. Major concepts of copula, Monte Carlo sampling, Markov chain Monte Carlo, polynomial regression, Gaussian process regression, polynomial chaos expansion, stochastic collocation, Bayesian inference, modelform uncertainty, multi-fidelity modeling, model validation, local and global sensitivity analyses, linear and nonlinear dimensionality reduction are included. Advanced UQ methods are also introduced, including stochastic processes, stochastic differential equations, random fields, fractional stochastic differential equations, hidden Markov model, linear Gaussian state space model, as well as non-probabilistic methods such as robust Bayesian analysis, Dempster-Shafer theory, imprecise probability, and interval probability. The book also includes example applications in multiscale modeling, reliability, fatigue, materials design, machine learning, and decision making.
• Features examples from a wide variety of science and engineering disciplines (e.g., fluids, structural dynamics, materials, manufacturing, multiscale simulation)
• Discusses sampling methods, surrogate modeling, stochastic expansion, sensitivity analysis, dimensionality reduction and more
Preface
PART 1 Fundamentals of uncertainty quantification
- Uncertainty quantification for engineering decision making
- Probability and statistics in uncertainty quantification
- Sampling methods in uncertainty quantification 85
- Surrogate modeling in uncertainty quantification
- Stochastic expansion methods in uncertainty quantification
- Bayesian inference in uncertainty quantification
- Sensitivity analysis in uncertainty quantification
- Linear and nonlinear dimensionality reduction techniques in uncertainty quantification
- Applications of uncertainty quantification in engineering
PART 2 Advanced topics of uncertainty quantification
10. Stochastic processes in uncertainty quantification
11. Markov models in uncertainty quantification
12. Nonprobabilistic methods in uncertainty quantification
Index
- Edition: 1
- Published: May 5, 2025
- Imprint: Elsevier
- Language: English
YW
Yan Wang
AT
Anh.V. Tran
DM
David L. Mcdowell
David L. McDowell Ph.D. is Regents’ Professor Emeritus at the Georgia Institute of Technology, having joined Georgia Tech as a faculty member in 1983. His research focuses on multiscale modelling of materials with emphasis on multiscale modeling of the inelastic behavior of metals, microstructure-sensitive computational fatigue analysis of microstructures, methods for materials design that are robust against uncertainty, and coarse-grained atomistic modelling methods.