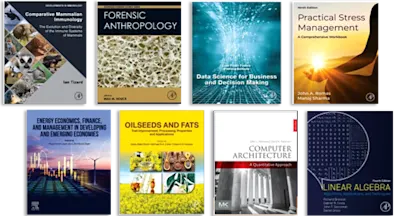
Fundamentals of AI for Medical Education, Research and Practice
- 1st Edition - January 20, 2025
- Imprint: Academic Press
- Author: Sameer Mohommed Khan
- Language: English
- Paperback ISBN:9 7 8 - 0 - 4 4 3 - 3 3 5 8 4 - 6
- eBook ISBN:9 7 8 - 0 - 4 4 3 - 3 3 5 8 5 - 3
Fundamentals of AI for Medical Education, Research and Practice provides a comprehensive introduction on all aspects of AI application in healthcare, ranging from medical education… Read more
Purchase options
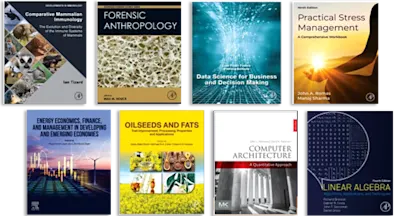
- Introduces the basic aspects of artificial intelligence to readers
- Presents practical applications of AI to enhance the quality of healthcare
- Encompasses wide range of topics in all the aspects of healthcare
- Immensely useful to get an overall idea about AI and what to expect from it in the future
1. Timeline of Artificial Intelligence (AI)
2. Understanding Artificial Intelligence (AI) in Health care and education
3. Overview of AI Technologies and Their Impact on Healthcare
4. Ethical and Regulatory Considerations in AI Adoption in Healthcare
Part II: AI in Medical Education
5. Integration of AI into Medical Curriculum: Challenges and Opportunities
6. AI-enabled Simulation and Virtual Learning Environments
7. AI-based Personalized Learning in Medical Education
8. Assessment with AI in Medical Training
Part III: AI in Healthcare Research
9. AI in Clinical Research: Transforming Methodologies and Discoveries
10. Data Science and AI Techniques in Healthcare Research
11. AI-driven Drug Discovery and Development
12. Predictive Analytics and AI in Epidemiological Studies
Part IV: AI in Clinical Practice
13. AI in Diagnostics: Enhancing Accuracy and Efficiency
14. AI-enabled Decision Support Systems in Clinical Practice
15. Robotics and AI-assisted Surgery: Advancements and Applications
16. Personalized Medicine and AI-driven Treatment Plans
Part V: Future Directions and Challenges
17. AI in Telemedicine and Remote Patient Monitoring
18. Emerging Trends in AI in Healthcare: Innovations and Opportunities
19. Challenges and Limitations of AI Adoption in Healthcare
20. Training the Future Healthcare Workforce for AI Integration
Part VI: Case Studies and Practical Applications
21. Case Studies: Real-world Examples of AI Implementation in Healthcare
22. Practical Exercises: Hands-on Activities for Applying AI Concepts in Healthcare
- Edition: 1
- Published: January 20, 2025
- Imprint: Academic Press
- Language: English
SK
Sameer Mohommed Khan
Dr. Sameer Khan is an esteemed Assistant Professor within the Department of Physiology at the College of Medicine, University of Bisha, in Saudi Arabia. With a distinguished career spanning over 12 years, Dr. Khan has been dedicated to enriching the minds of both undergraduate and postgraduate students through his expertise in human physiology and medical education, where he specializes in interprofessional education with vast experience in both traditional and outcome-based curriculum. He has been a part of various college committees, actively involved in curriculum planning, designing, and mapping. Recognized for his academic excellence, Dr. Khan was honored as the most outgoing postgraduate and bestowed with a silver medal for his exceptional performance by the Department of Physiology, KMC Mangalore. Dr. Khan's commitment to advancing medical knowledge extends beyond the classroom with publications of “Practical Physiology: A New Approach” (Jaypee) and “Fundamentals of AI for Medical Education, Research and Practice” (Elsevier).