LIMITED OFFER
Save 50% on book bundles
Immediately download your ebook while waiting for your print delivery. No promo code needed.
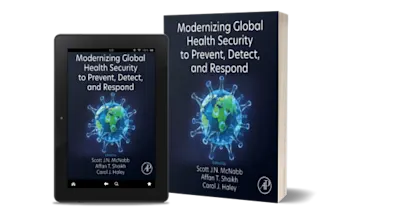
Fundamental Statistical Principles for Neurobiologists introduces readers to basic experimental design and statistical thinking in a comprehensive, relevant manner. This book is a… Read more
LIMITED OFFER
Immediately download your ebook while waiting for your print delivery. No promo code needed.
Fundamental Statistical Principles for Neurobiologists introduces readers to basic experimental design and statistical thinking in a comprehensive, relevant manner. This book is an introductory statistics book that covers fundamental principles written by a neuroscientist who understands the plight of the neuroscience graduate student and the senior investigator. It summarizes the fundamental concepts associated with statistical analysis that are useful for the neuroscientist, and provides understanding of a particular test in language that is more understandable to this specific audience, with the overall purpose of explaining which statistical technique should be used in which situation. Different types of data are discussed such as how to formulate a research hypothesis, the primary types of statistical errors and statistical power, followed by how to actually graph data and what kinds of mistakes to avoid. Chapters discuss variance, standard deviation, standard error, mean, confidence intervals, correlation, regression, parametric vs. nonparametric statistical tests, ANOVA, and post hoc analyses. Finally, there is a discussion on how to deal with data points that appear to be "outliers" and what to do when there is missing data, an issue that has not sufficiently been covered in literature.
Neuroscientists, graduate students/post-docs in biological and biomedical sciences
SS