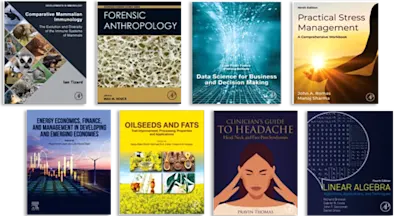
Federated Learning for Medical Imaging
Principles, Algorithms, and Applications
- 1st Edition - March 17, 2025
- Imprint: Academic Press
- Editors: Xiaoxiao Li, Ziyue Xu, Huazhu Fu
- Language: English
- Paperback ISBN:9 7 8 - 0 - 4 4 3 - 2 3 6 4 1 - 9
- eBook ISBN:9 7 8 - 0 - 4 4 3 - 2 3 6 4 2 - 6
Federated Learning for Medical Imaging: Principles, Algorithms, and Applications gives a deep understanding of the technology of federated learning (FL), the architecture of a fe… Read more
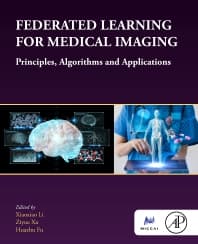
Purchase options
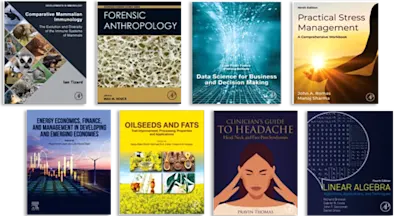
Institutional subscription on ScienceDirect
Request a sales quoteFederated Learning for Medical Imaging: Principles, Algorithms, and Applications gives a deep understanding of the technology of federated learning (FL), the architecture of a federated system, and the algorithms for FL. It shows how FL allows multiple medical institutes to collaboratively train and use a precise machine learning (ML) model without sharing private medical data via practical implantation guidance. The book includes real-world case studies and applications of FL, demonstrating how this technology can be used to solve complex problems in medical imaging. The book also provides an understanding of the challenges and limitations of FL for medical imaging, including issues related to data and device heterogeneity, privacy concerns, synchronization and communication, etc.
This book is a complete resource for computer scientists and engineers, as well as clinicians and medical care policy makers, wanting to learn about the application of federated learning to medical imaging.
- Presents the specific challenges in developing and deploying FL to medical imaging
- Explains the tools for developing or using FL
- Presents the state-of-the-art algorithms in the field with open source software on Github
- Gives insight into potential issues and solutions of building FL infrastructures for real-world application
- Informs researchers on the future research challenges of building real-world FL applications
Academic and industry researchers in biomedical engineering, computer science and electronic engineering researching into medical imaging, Masters and PhD students studying and researching medical imaging
- Title of Book
- Cover image
- Title page
- Table of Contents
- Copyright
- List of contributors
- Preface
- Part 1: Fundamental of federated learning
- Chapter 1: Introduction of federated learning
- 1.1. What is federated learning?
- 1.2. Motivation for federated learning
- 1.3. Major challenges for FL
- Chapter 2: Fundamentals of federated learning
- 2.1. Terminology
- 2.2. The definition of federated learning
- 2.3. Comparing federated learning and other training strategies
- 2.4. Taxonomy of federated learning
- 2.4.1. Horizontal federated learning (HFL)
- 2.4.2. Vertical federated learning (VFL)
- 2.4.3. Cross-device federated learning
- 2.4.4. Cross-silo federated learning
- 2.5. Collaboration topology
- 2.6. Summary
- Part 2: Advanced concepts and methods
- Introduction
- Chapter 3: Data heterogeneity: a curse and a blessing for federated learning
- 3.1. Data heterogeneity for medical imaging
- 3.2. Heterogeneity, or diversity?
- 3.3. Methods to reduce heterogeneity
- 3.3.1. Local optimization with constraints
- 3.3.2. Selective collaboration and aggregation
- 3.3.3. Combined methods
- 3.3.4. Higher-level decoupling
- 3.4. Methods to utilize diversity
- 3.5. Conclusion
- Chapter 4: Personalized federated learning strategies
- 4.1. Introduction
- 4.2. Learning global model for personalization
- 4.2.1. Meta-learning
- 4.2.2. Transfer learning
- 4.3. Learning personalized model
- 4.3.1. Clustering
- 4.3.2. Multitask learning
- 4.3.3. Knowledge distillation
- 4.4. Conclusion
- Chapter 5: Federated learning on long-tailed datasets
- 5.1. Introduction and background
- 5.2. Preliminaries and related work
- 5.2.1. Long-tailed learning
- 5.2.2. Federated long-tailed learning
- 5.3. Case studies
- 5.3.1. Gastrointestinal recognition
- 5.3.2. Skin lesion classification
- 5.4. Methodology
- 5.4.1. FedMAS
- 5.4.2. Methodology training
- 5.4.3. Evaluation metrics
- 5.5. Experimental and results
- 5.5.1. Gastrointestinal image recognition
- 5.5.2. Skin lesion classification
- 5.6. Discussion
- 5.7. Conclusion
- Chapter 6: Expanding the federated horizon: cross-domain techniques for collective intelligence
- 6.1. Introduction
- 6.2. Conceptual overview
- 6.2.1. Environment heterogeneity
- 6.2.2. Task heterogeneity
- 6.2.3. Model heterogeneity
- 6.3. A framework for cross-domain FL
- 6.3.1. Optimization approach
- 6.3.2. Architectural approach
- 6.3.3. Bringing it all together
- 6.4. Outstanding challenges and future outlook
- Part 3: Trustworthy federated learning
- Introduction
- Chapter 7: Improving performance fairness in federated learning for heterogeneous medical images
- 7.1. Introduction
- 7.2. Technical approaches for fairness in FL
- 7.2.1. Performance distribution fairness
- 7.2.2. Individual fairness
- 7.2.3. Group fairness
- 7.2.4. Contribution fairness
- 7.3. Improving performance distribution fairness by harmonizing heterogeneous medical images
- 7.3.1. Preliminaries
- 7.3.2. Amplitude normalization for local training
- 7.3.3. Weight perturbation for global aggregation
- 7.3.4. Experiments
- 7.4. Discussion and summary
- Chapter 8: Differential privacy
- 8.1. Motivation
- 8.1.1. A nontechnical primer
- 8.2. The promise of differential privacy
- 8.2.1. Approximate differential privacy
- 8.2.2. The privacy-utility tradeoff
- 8.3. Achieving differential privacy
- 8.3.1. Laplace vs Gaussian mechanism
- 8.3.2. Properties of differential privacy
- 8.3.3. Central vs local trust model
- 8.4. Differentially private machine learning
- 8.4.1. Private stochastic gradient descent
- 8.4.2. A final note of caution for deployment
- 8.5. Conclusion
- Chapter 9: Utility-privacy tradeoff in federated learning
- 9.1. Introduction
- 9.2. Differential privacy
- 9.3. Reprogrammable-FL
- 9.3.1. Model reprogramming
- 9.3.2. Training of Reprogrammable-FL
- 9.3.3. Model reprogramming in federated learning
- 9.4. Performance of Reprogrammable-FL and other fine-tuning methods
- 9.5. Alternative to DP-SGD fine-tuning
- 9.6. Recent work on DP-SGD fine-tuning
- 9.7. Challenges to DP-SGD implementation
- Chapter 10: Reliable federated learning for disease detection
- 10.1. Introduction
- 10.2. Related works
- 10.3. Methodology
- 10.3.1. Temperature-warmed evidential belief head
- 10.3.2. Confidence-aware weighting module
- 10.4. Loss function
- 10.5. Experimental results
- 10.6. Conclusion
- Chapter 11: Federated noisy client learning
- 11.1. Introduction
- 11.2. Related works
- 11.2.1. Deep learning with noisy label
- 11.2.2. Federated learning with noisy label
- 11.3. Problem statement
- 11.3.1. Federated learning with noisy client
- 11.3.2. Modeling noisy client
- 11.4. Federated noisy client learning
- 11.4.1. Data quality measurement
- 11.4.2. Noise robust aggregation
- 11.4.3. Convergence
- 11.5. Evaluation
- 11.5.1. Experimental setup
- 11.5.2. Evaluation with heterogeneous noise distributions
- 11.6. Summary
- Part 4: Real-world implementation and application
- Introduction
- Chapter 12: Real-world implementation and application of federated medical image segmentation
- 12.1. Introduction
- 12.2. Clinical applications
- 12.2.1. Brain tumor segmentation
- 12.2.2. Covid-19 lesion segmentation
- 12.2.3. Ocular image segmentation
- 12.2.4. Pancreas segmentation
- 12.3. Personalized medical image segmentation
- 12.3.1. Task personalization using multitask learning
- 12.3.2. Distribution personalization using local adaption
- 12.4. Data-efficient federated medical image segmentation
- 12.4.1. Semisupervised federated medical image segmentation
- 12.4.2. Self-supervised federated learning
- Chapter 13: Enhancing MRI reconstruction with cross-silo federated learning
- 13.1. Introduction
- 13.2. Related work
- 13.3. Methodology
- 13.3.1. FL-based MRI reconstruction
- 13.3.2. FL-MR with cross-site modeling
- 13.3.3. Training and implementation details
- 13.4. Experiments and results
- 13.4.1. Datasets
- 13.4.2. Evaluation of the generalizability
- 13.4.3. Evaluation of FL-based collaborations
- 13.4.4. Ablation study
- 13.5. Conclusion
- Chapter 14: Real-world implementation and application
- 14.1. NVFlare
- 14.2. FATE
- 14.3. OpenFL
- 14.4. Flower
- 14.5. IBM federated learning
- 14.6. Others
- 14.6.1. PySyft
- 14.6.2. TensorFlow federated
- 14.6.3. LEAF
- 14.6.4. FedScale
- 14.6.5. FedML
- 14.7. Comparison
- Chapter 15: Summary and outlook
- 15.1. Summary
- 15.2. Challenges and limitations
- 15.3. Outlook
- Bibliography
- Index
- Edition: 1
- Published: March 17, 2025
- Imprint: Academic Press
- No. of pages: 260
- Language: English
- Paperback ISBN: 9780443236419
- eBook ISBN: 9780443236426
XL
Xiaoxiao Li
ZX
Ziyue Xu
HF