ROBOTICS & AUTOMATION
Empowering Progress
Up to 25% off Essentials Robotics and Automation titles
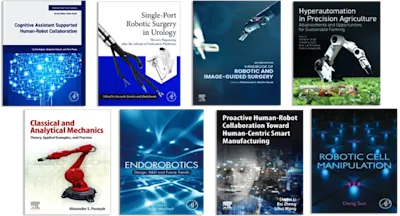
We use cookies that are necessary to make our site work. We may also use additional cookies to analyze, improve, and personalize our content and your digital experience. For more information, see our Cookie Policy.
Federated Learning for Digital Healthcare Systems critically examines the key factors that contribute to the problem of applying machine learning in healthcare systems and inves… Read more
ROBOTICS & AUTOMATION
Up to 25% off Essentials Robotics and Automation titles
Federated Learning for Digital Healthcare Systems critically examines the key factors that contribute to the problem of applying machine learning in healthcare systems and investigates how federated learning can be employed to address the problem. The book discusses, examines, and compares the applications of federated learning solutions in emerging digital healthcare systems, providing a critical look in terms of the required resources, computational complexity, and system performance.
In the first section, chapters examine how to address critical security and privacy concerns and how to revamp existing machine learning models. In subsequent chapters, the book's authors review recent advances to tackle emerging efficient and lightweight algorithms and protocols to reduce computational overheads and communication costs in wireless healthcare systems. Consideration is also given to government and economic regulations as well as legal considerations when federated learning is applied to digital healthcare systems.
AI
FX
MO
HS