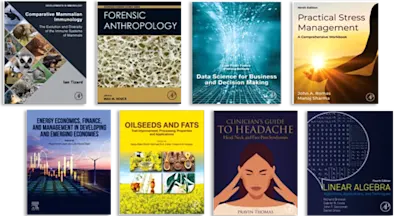
Explainable Deep Learning AI
Methods and Challenges
- 1st Edition - February 20, 2023
- Imprint: Academic Press
- Editors: Jenny Benois-Pineau, Romain Bourqui, Dragutin Petkovic, Georges Quenot
- Language: English
- Paperback ISBN:9 7 8 - 0 - 3 2 3 - 9 6 0 9 8 - 4
- eBook ISBN:9 7 8 - 0 - 3 2 3 - 9 9 3 8 8 - 3
Explainable Deep Learning AI: Methods and Challenges presents the latest works of leading researchers in the XAI area, offering an overview of the XAI area, along with several n… Read more
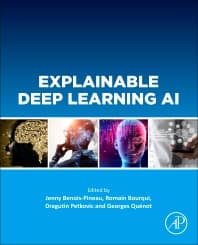
Purchase options
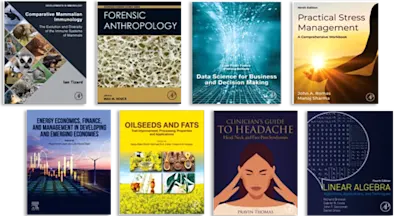
Institutional subscription on ScienceDirect
Request a sales quoteExplainable Deep Learning AI: Methods and Challenges presents the latest works of leading researchers in the XAI area, offering an overview of the XAI area, along with several novel technical methods and applications that address explainability challenges for deep learning AI systems. The book overviews XAI and then covers a number of specific technical works and approaches for deep learning, ranging from general XAI methods to specific XAI applications, and finally, with user-oriented evaluation approaches. It also explores the main categories of explainable AI – deep learning, which become the necessary condition in various applications of artificial intelligence.
The groups of methods such as back-propagation and perturbation-based methods are explained, and the application to various kinds of data classification are presented.
- Provides an overview of main approaches to Explainable Artificial Intelligence (XAI) in the Deep Learning realm, including the most popular techniques and their use, concluding with challenges and exciting future directions of XAI
- Explores the latest developments in general XAI methods for Deep Learning
- Explains how XAI for Deep Learning is applied to various domains like images, medicine and natural language processing
- Provides an overview of how XAI systems are tested and evaluated, specially with real users, a critical need in XAI
- Cover image
- Title page
- Table of Contents
- Copyright
- List of contributors
- Preface
- Chapter 1: Introduction
- Abstract
- Chapter 2: Explainable deep learning: concepts, methods, and new developments
- Abstract
- Acknowledgement
- 2.1. Introduction
- 2.2. Concepts
- 2.3. Methods
- 2.4. New developments
- 2.5. Limitations and future work
- References
- Chapter 3: Compact visualization of DNN classification performances for interpretation and improvement
- Abstract
- Acknowledgements
- 3.1. Introduction
- 3.2. Previous works
- 3.3. Proposed method for compact visualization of DNN classification performances
- 3.4. Experimental protocol
- 3.5. Results and discussion
- 3.6. Conclusion
- References
- Chapter 4: Characterizing a scene recognition model by identifying the effect of input features via semantic-wise attribution
- Abstract
- Acknowledgements
- 4.1. Introduction
- 4.2. Semantic-wise attribution
- 4.3. Experimental results
- 4.4. Conclusions
- References
- Chapter 5: A feature understanding method for explanation of image classification by convolutional neural networks
- Abstract
- Acknowledgements
- 5.1. Introduction
- 5.2. Principles of white-box explanation methods
- 5.3. Explanation methods
- 5.4. The proposed improvement – modified FEM
- 5.5. Experimental results
- 5.6. Conclusion
- References
- Chapter 6: Explainable deep learning for decrypting disease signatures in multiple sclerosis
- Abstract
- Acknowledgements
- 6.1. Introduction
- 6.2. State-of-the-art
- 6.3. Materials and methods
- 6.4. Results
- 6.5. Discussion
- 6.6. Conclusions
- References
- Chapter 7: Explanation of CNN image classifiers with hiding parts
- Abstract
- Acknowledgements
- 7.1. Introduction
- 7.2. Explanation methods
- 7.3. Recursive division approach
- 7.4. Quality of the model
- 7.5. Experimental modeling
- 7.6. Conclusion
- References
- Chapter 8: Remove to improve?
- Abstract
- Glossary
- Acknowledgements
- 8.1. Introduction
- 8.2. Previous work
- 8.3. Definitions
- 8.4. Experiments
- 8.5. Model selection
- 8.6. Discussion and conclusions
- References
- Chapter 9: Explaining CNN classifier using association rule mining methods on time-series
- Abstract
- 9.1. Introduction
- 9.2. Related work
- 9.3. Background
- 9.4. Methods
- 9.5. Evaluation metrics
- 9.6. Experimental results
- 9.7. Conclusion and future work
- References
- Chapter 10: A methodology to compare XAI explanations on natural language processing
- Abstract
- Glossary
- 10.1. Introduction
- 10.2. Related works
- 10.3. Generating explanations
- 10.4. Evaluation without end users
- 10.5. Psychometric user study
- 10.6. Conclusion
- References
- Chapter 11: Improving malware detection with explainable machine learning
- Abstract
- 11.1. Introduction
- 11.2. Background
- 11.3. Explanation methods
- 11.4. Explaining Android ransomware
- 11.5. Experimental analysis
- 11.6. Discussion
- 11.7. Conclusion
- References
- Chapter 12: Explainability in medical image captioning
- Abstract
- Acknowledgement
- 12.1. Introduction
- 12.2. Related work
- 12.3. Methodology
- 12.4. Experimental results
- 12.5. Conclusion and future work
- References
- Chapter 13: User tests & techniques for the post-hoc explanation of deep learning
- Abstract
- Acknowledgements
- 13.1. Introduction
- 13.2. Post-hoc explanations using factual examples
- 13.3. Counterfactual & semifactual explanations: images
- 13.4. Contrastive explanations: time series
- 13.5. User studies on contrastive explanations
- 13.6. Conclusions
- References
- Chapter 14: Theoretical analysis of LIME
- Abstract
- Acknowledgements
- 14.1. Introduction
- 14.2. LIME for images
- 14.3. LIME for text data
- 14.4. LIME for tabular data
- 14.5. Conclusion
- References
- Chapter 15: Conclusion
- Abstract
- Index
- Edition: 1
- Published: February 20, 2023
- Imprint: Academic Press
- No. of pages: 346
- Language: English
- Paperback ISBN: 9780323960984
- eBook ISBN: 9780323993883
JB
Jenny Benois-Pineau
RB
Romain Bourqui
DP
Dragutin Petkovic
GQ