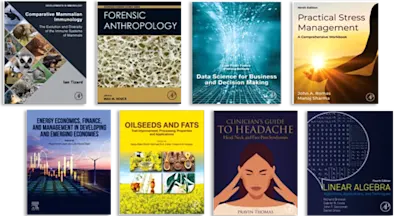
Essentials of Time Series for Financial Applications
- 1st Edition - May 29, 2018
- Imprint: Academic Press
- Authors: Massimo Guidolin, Manuela Pedio
- Language: English
- Paperback ISBN:9 7 8 - 0 - 1 2 - 8 1 3 4 0 9 - 2
- eBook ISBN:9 7 8 - 0 - 1 2 - 8 1 3 4 1 0 - 8
Essentials of Time Series for Financial Applications serves as an agile reference for upper level students and practitioners who desire a formal, easy-to-follow introduct… Read more
Purchase options
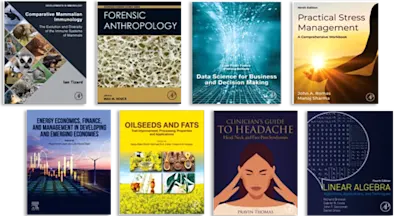
Essentials of Time Series for Financial Applications serves as an agile reference for upper level students and practitioners who desire a formal, easy-to-follow introduction to the most important time series methods applied in financial applications (pricing, asset management, quant strategies, and risk management). Real-life data and examples developed with EViews illustrate the links between the formal apparatus and the applications. The examples either directly exploit the tools that EViews makes available or use programs that by employing EViews implement specific topics or techniques. The book balances a formal framework with as few proofs as possible against many examples that support its central ideas. Boxes are used throughout to remind readers of technical aspects and definitions and to present examples in a compact fashion, with full details (workout files) available in an on-line appendix. The more advanced chapters provide discussion sections that refer to more advanced textbooks or detailed proofs.
- Provides practical, hands-on examples in time-series econometrics
- Presents a more application-oriented, less technical book on financial econometrics
- Offers rigorous coverage, including technical aspects and references for the proofs, despite being an introduction
- Features examples worked out in EViews (9 or higher)
MSc. students in Finance, Quantitative Methods (who specialize in finance), Mathematical Finance, and Engineering. Ph.D. students who need an introductory back-up to applied courses (such as Financial Econometrics graduate courses). Practitioners in derivative pricing, trading, and quantitative strategies. Asset managers, risk managers, and research analysts who focus on forecasting market quantities
1. Review of Key Concepts and Methods in Econometrics: Regressions Analysis2. Autoregressive-Moving Average (ARMA) Models and their Practical Applications.3. Vector Autoregressive Moving Average (VARMA) Models4. Unit Roots and Cointegration Methods5. Univariate Single-Factor Stochastic Volatility Models: Autoregressive Conditional Heteroskedasticity(ARCH and GARCH)6. Multivariate ARCH and GARCH and Dynamic Conditional Correlation Models7. Multi-Factor Volatility Models: Stochastic Volatility8. Models with Breaks, Recurrent Regime Switching, and Non-Linearities9. Markov Switching Models10. Realized Volatility and Covariance
- Edition: 1
- Published: May 29, 2018
- Imprint: Academic Press
- Language: English
MG
Massimo Guidolin
MP