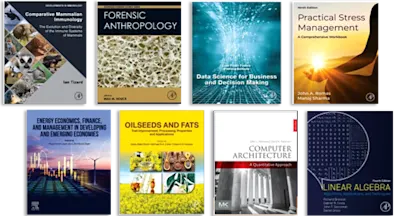
EEG-Based Diagnosis of Alzheimer Disease
A Review and Novel Approaches for Feature Extraction and Classification Techniques
- 1st Edition - April 18, 2018
- Imprint: Academic Press
- Authors: Nilesh Kulkarni, Vinayak Bairagi
- Language: English
- Paperback ISBN:9 7 8 - 0 - 1 2 - 8 1 5 3 9 2 - 5
- eBook ISBN:9 7 8 - 0 - 1 2 - 8 1 5 3 9 3 - 2
EEG-Based Diagnosis of Alzheimer Disease: A Review and Novel Approaches for Feature Extraction and Classification Techniques provides a practical and easy-to-use guide for resea… Read more
Purchase options
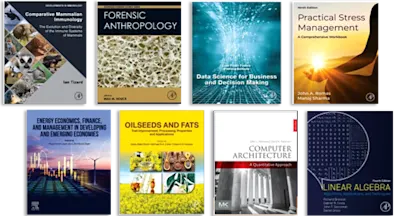
EEG-Based Diagnosis of Alzheimer Disease: A Review and Novel Approaches for Feature Extraction and Classification Techniques provides a practical and easy-to-use guide for researchers in EEG signal processing techniques, Alzheimer’s disease, and dementia diagnostics. The book examines different features of EEG signals used to properly diagnose Alzheimer’s Disease early, presenting new and innovative results in the extraction and classification of Alzheimer’s Disease using EEG signals. This book brings together the use of different EEG features, such as linear and nonlinear features, which play a significant role in diagnosing Alzheimer’s Disease.
- Includes the mathematical models and rigorous analysis of various classifiers and machine learning algorithms from a perspective of clinical deployment
- Covers the history of EEG signals and their measurement and recording, along with their uses in clinical diagnostics
- Analyzes spectral, wavelet, complexity and other features of early and efficient Alzheimer’s Disease diagnostics
- Explores support vector machine-based classification to increase accuracy
Biomedical engineers and researchers and engineers in EEG signal processing and allied domains
Chapter 1: Introduction
1.1 What is Alzheimer’s Disease?
1.2 Causes and Symptoms of the disease
1.3 Stages and Clinical Diagnosis of the Disease
1.4 Importance of Diagnosis of Alzheimer’s disease and its impact on Society
1.5 A Brief Review on Different methods used for diagnosis of Alzheimer of Alzheimer disease
1.5.1 Role of Neuroimaging based techniques in diagnosis of Alzheimer disease
1.5.2 Role of Electroencephalogram techniques in diagnosis of Alzheimer disease
1.6 Summary
Chapter 2: Electroencephalogram and Its Use in Clinical Neuroscience
2.1 Introduction
2.2 EEG Recording techniques and Measurement
2.3 EEG Rhythms and their significance
2.4 Early Diagnosis of Alzheimer disease using EEG signals
2.5 Summary
Chapter 3: Role of Different Features in Diagnosis of Alzheimer’s Disease
3.1 Introduction
3.2 What is Feature extraction?
3.3 Need of Feature Extraction in EEG signals
3.4 Linear Features
3.4.1 Spectral Features
3.4.2 Wavelet Based Features
3.5 Non-Linear Features
3.5.1 Role of Complexity based features
3.5.2 Synchrony based features
Chapter 4: Use of Complexity-Based Features in the Diagnosis of Alzheimer’s Disease
Chapter 5: Classification Algorithms in the Diagnosis of Alzheimer’s Disease
Chapter 6: Discussion and Research Challenges
- Edition: 1
- Published: April 18, 2018
- Imprint: Academic Press
- Language: English
VB