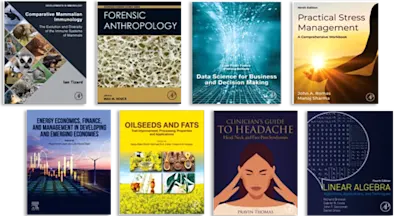
Dynamic Modelling of Time-to-Event Processes
- 1st Edition - October 1, 2025
- Imprint: Elsevier
- Author: Gangaram S. Ladde
- Editors: Gangaram S. Ladde, Emmanuel A Appiah, Jay G. Ladde
- Language: English
- Paperback ISBN:9 7 8 - 0 - 4 4 3 - 2 2 3 4 3 - 3
- eBook ISBN:9 7 8 - 0 - 4 4 3 - 2 2 3 4 2 - 6
Dynamic Modelling of Time-to-Event Processes covers an alternative dynamic modelling approach for studying time-to-event processes. This innovative approach covers some key eleme… Read more
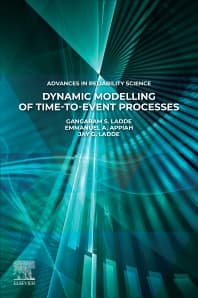
Purchase options
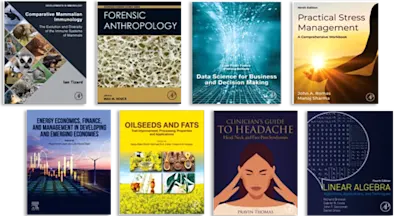
Institutional subscription on ScienceDirect
Request a sales quoteDynamic Modelling of Time-to-Event Processes covers an alternative dynamic modelling approach for studying time-to-event processes. This innovative approach covers some key elements, including the Development of continuous-time state of dynamic time-to-event processes, an Introduction of an idea of discrete-time dynamic intervention processes, Treating a time-to-event process operating/functioning under multiple time-scales formulation of continuous and discrete-time interconnected dynamic system as hybrid dynamic time-to-event process, Utilizing Euler-type discretized schemes, developing theoretical dynamic algorithms, and more.
Additional elements of this process include an Introduction of conceptual and computational state and parameter estimation procedures, Developing multistage a robust mean square suboptimal criterion for state and parameter estimation, and Extending the idea conceptual computational simulation process and applying real datasets.
- Presents a dynamic approach which does not require a closed-form survival/reliability distribution
- Provides updates that are independent of existing Maximum likelihood, Bayesian, and Nonparametric methods
- Applies to nonlinear and non-stationary interconnected large-scale dynamic systems
- Includes frailty and other models in survival analysis as case studies
Undergraduate and graduate students; Key knowledge and information needs: Calculus, some knowledge of differential equations, and probability and statistics. Interdisciplinary researchers and practitioners in the biological, medical, technological, chemical, medical and social sciences sectors.
1.1. Introduction
1.2. Preliminary Concepts and Results in Survival and Reliability analysis
1.3. Motivations for Continuous time Dynamic of Time-to-Event Processes
1.4. Illustration Existing Dynamic Model of Time-to-Event-Process
1.5. Basic Components and functions of Hybrid Dynamic Process
1.6. Notes and Comments
2. Linear Deterministic Hybrid Dynamic Modeling of Time-to-event Processes (LDHDM)
2.1. Introduction
2.2. Linear Continuous-time hybrid dynamic model Formulation
2.3. Linear Discrete-time hybrid dynamic Model Formulation
2.4. Fundamental Results for Continuous-time Dynamic processes
2.5. A Few Results for Discrete-time Hybrid Iterative Processes
2.6. Estimation of Survival and Risk Rate Parameters
2.7. Estimation of Survival and Risk States
2.8. Analysis of Multiple Censored times between Consecutive Failure Times
2.9. Notes and Comments
3. Conceptual Computational and Simulation Algorithms - LDHDM
3.1. Introduction3.2. Conceptual computational parameter and state estimation schemes
3.3. Conceptual computational simulation algorithms
3.4. Simulation Algorithm for Interconnected Hybrid Dynamic Process
3.5. Notes and Comments
4. Nonlinear Deterministic Interconnected Hybrid Dynamic Modeling for Time-to-Event Processes - INHDMTTEP
4.1. Introduction
4.2. Basic Concepts and Modifications
4.3. Motivation for Nonlinear Formulation with Illustrations
4.4. Formulation of Large-scale Nonlinear Hybrid Dynamic Model
4.5. Derivation of Theoretical Interconnected Discrete-time Dynamic Algorithm – IDATTEDS
4.6. Theoretical Parameters and State Estimations
4.6.1. Theoretical Parameter Estimations of Multiple Censored and Admittance between Two Consecutive Failure Times
4.6.2. Parameter and State Estimation for Totally Discrete-time Hybrid Dynamic Model
4.6.3. Modified Local lagged Adaptive Generalized Method of Moments (LLGMM) Parameter and State Estimation Schemes
4.7. Change-point Data Analysis Problem
4.8. Notes and Comments
5. Conceptual Computational and Simulation Algorithms for INHDMTTEP
5.1. Introduction
5.2. Data Collection and Coordination with Iterative Processes
5.3. Data Decomposition, Reorganization, and Aggregation Process
5.4. Conceptual Computational Parameter and State Estimation Schemes - IDATTEDS
5.5. Conceptual Computational State Simulation Schemes – IDATTEDS
5.6. Modified LLGMM Conceptual Computational Simulation Schemes and Algorithms
5.7. Notes and Comments
6. Stochastic Hybrid Dynamic Modeling for Time-to-event Processes - SIHDMTTEP
6.1. Introduction
6.2. Motivation and Formulation of Stochastic Hybrid Dynamic Model
6.3. Fundamental Results for Stochastic Hybrid Dynamic Processes
6.4. Theoretical Conceptual Parameter and State Estimation Schemes – SIDANTTEDS
6.5. Fundamental Conceptual Computational Discrete-time Data Observation Systems
6.6. Development of Discrete time Conceptual Computational Dynamic State and Parameter Estimation Problem
6.7. Theoretical Parameter Estimations of Multiple Censored and Admittance between Two Consecutive Failure Times
6.8. Modified LLGMM conceptual computational parameter and state estimation.
6.9. Change-point Data Analysis Problem6.10. Notes and Comments
7. Conceptual Computational and Simulation Algorithms for SIHDMTTEP
7.1. Introduction
7.2. Data Collection Coordination with Iterative Processes
7.3. Data Decomposition, Reorganization, and Aggregation Process
7.4. Conceptual Computational Parameter and State Estimation Schemes – SIDANTTTEDS
7.5. Conceptual Computational State Simulation Schemes – SIDANTTEDS
7.6. Modified LLGMM Conceptual Computational Simulation Schemes Algorithms
7.7. Notes and Comment
8. Application to Time-to-Event Datasets
8.1. Introduction
8.2. Case study: Application of IDATTEDS to Time-to-Event Datasets
8.3. Case study: Application of Modified LLGMM to Time-to-Event Datasets
8.3.1. Application of Deterministic Modified LLGMM to Time-to-Event Datasets
8.3.2. Application of Stochastic Modified LLGMM to Time-to-Event Datasets
8.4. Notes and Comments
9. Statistical Comparative Analysis with Existing Methods
9.1. Introduction
9.2. Comparison of LHDDM with Existing Methods
9.3. Comparison of IDATTEDS with Existing Methods
9.4. Comparison of Modified LLGMM with Existing Methods
9.4.1. Comparison of Deterministic Modified LLGMM with Existing Methods
9.4.2. Comparison of Stochastic Modified LLGMM with Existing Methods
9.5. Notes and Comments
10. Case Studies
10.1. Introduction
10.2. Adoption and Replacement for Succeeding Generation of High Technology Products
10.3. Application of Time-to-event Processes for Evaluating Demand Side Management
10.4. Generalized Network Externality Process
10.5. Application of Time-to-event Processes to Marketing Decision Making
10.6. Role and Scope Mathematical Sciences
10.7. Baseline and Marshall-Olkin-type Modified Distributions
10.8. Notes and Comments
- Edition: 1
- Published: October 1, 2025
- Imprint: Elsevier
- Language: English
- Paperback ISBN: 9780443223433
- eBook ISBN: 9780443223426
GS
Gangaram S. Ladde
EA
Emmanuel A Appiah
JG
Jay G. Ladde
GS