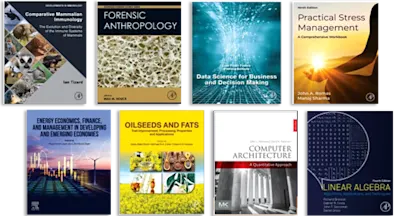
Advances in Domain Adaptation Theory
- 1st Edition - August 14, 2019
- Imprint: ISTE Press - Elsevier
- Authors: Ievgen Redko, Emilie Morvant, Amaury Habrard, Marc Sebban, Younès Bennani
- Language: English
- Hardback ISBN:9 7 8 - 1 - 7 8 5 4 8 - 2 3 6 - 6
- eBook ISBN:9 7 8 - 0 - 0 8 - 1 0 2 3 4 7 - 1
Advances in Domain Adaptation Theory gives current, state-of-the-art results on transfer learning, with a particular focus placed on domain adaptation from a theoretical point-of-… Read more
Purchase options
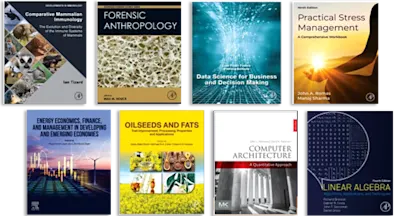
Advances in Domain Adaptation Theory gives current, state-of-the-art results on transfer learning, with a particular focus placed on domain adaptation from a theoretical point-of-view. The book begins with a brief overview of the most popular concepts used to provide generalization guarantees, including sections on Vapnik-Chervonenkis (VC), Rademacher, PAC-Bayesian, Robustness and Stability based bounds. In addition, the book explains domain adaptation problem and describes the four major families of theoretical results that exist in the literature, including the Divergence based bounds. Next, PAC-Bayesian bounds are discussed, including the original PAC-Bayesian bounds for domain adaptation and their updated version.
Additional sections present generalization guarantees based on the robustness and stability properties of the learning algorithm.
- Gives an overview of current results on transfer learning
- Focuses on the adaptation of the field from a theoretical point-of-view
- Describes four major families of theoretical results in the literature
- Summarizes existing results on adaptation in the field
- Provides tips for future research
Scientists, researchers and engineers interested in this subject area
- Edition: 1
- Published: August 14, 2019
- Imprint: ISTE Press - Elsevier
- Language: English
IR
Ievgen Redko
EM
Emilie Morvant
AH
Amaury Habrard
MS
Marc Sebban
YB