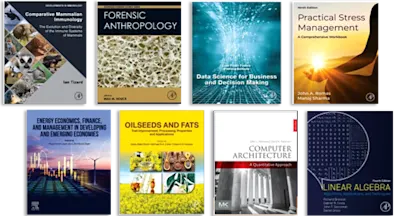
Dimensionality Reduction in Machine Learning
- 1st Edition - February 5, 2025
- Imprint: Morgan Kaufmann
- Editors: Jamal Amani Rad, Snehashish Chakraverty, Kourosh Parand
- Language: English
- Paperback ISBN:9 7 8 - 0 - 4 4 3 - 3 2 8 1 8 - 3
- eBook ISBN:9 7 8 - 0 - 4 4 3 - 3 2 8 1 9 - 0
Dimensionality Reduction in Machine Learning covers both the mathematical and programming sides of dimension reduction algorithms, comparing them in various aspects. Part One pr… Read more
Purchase options
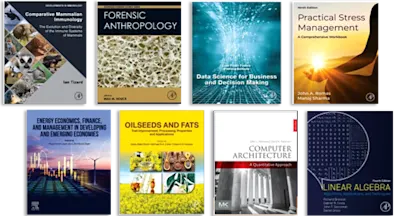
Finally, Part Four covers Deep Learning Methods for Dimension Reduction, with chapters on Feature Extraction and Deep Learning, Autoencoders, and Dimensionality reduction in deep learning through group actions. With this stepwise structure and the applied code examples, readers become able to apply dimension reduction algorithms to different types of data, including tabular, text, and image data.
- Provides readers with a comprehensive overview of various dimension reduction algorithms, including linear methods, non-linear methods, and deep learning methods
- Covers the implementation aspects of algorithms supported by numerous code examples
- Compares different algorithms so the reader can understand which algorithm is suitable for their purpose
- Includes algorithm examples that are supported by a Github repository which consists of full notebooks for the programming code
1 – Basics of Machine Learning
• Data Processing in ML
o What is Data? Feature? Pattern?
o Understanding data processing
o High Dimensional Data
• Types of Learning Problems
o Supervised Learning
o Unsupervised Learning
o Semi-Supervised Learning
o Reinforcement Learning
• Machine Leaning’s algorithms life-cycle
o 1st step: data cleaning & data preprocessing
o 2nd step: dimension reduction & feature extraction
o 3rd step: Model selection & model fitting
o 4th step: model evaluation
o Dealing with Challenges in Learning
• Python for Machine Learning
o Python and Packages Installation
2 – Essential Mathematics for Machine Learning
• Basic Algebra
o Binary Operations
o Algebraic Systems
• Linear Algebra and Matrix
o Matrix Decomposition
o Eigenvalue and Eigen Vector
• Optimization
o Unconstrained Optimization
o Constrained Optimization
3 – Feature Selection Methods
• Introduction to feature selection
o What is feature selection?
o How is it related to dimension reduction?
o Role of feature type in feature selection method
• Selection of numerical features
o ANOVA F-test Feature Selection
o Correlation Feature Selection
o Mutual Information Feature Selection
• Selection of categorical features
o Chi-Squared Feature Selection
o Mutual Information Feature Selection
• Recursive Feature Elimination
• Feature Importance
• Feature Selection in Python Using Scikit-learn
• Conclusion
Part 2: Linear Methods for Dimension Reduction
4 – Principal Component Analysis
• Introduction to PCA
• Understanding PCA algorithm
• Variants of PCA Algorithms
o Kernel PCA
o Robust PCA
• Implementing PCA in Python using Scikit-learn
• Advantages and Limitations of PCA
• Conclusion
5 – Linear Discriminant Analysis
• Introduction to linear discriminant analysis
o What is linear discriminant analysis?
o How does linear discriminant analysis work?
o Application of linear discriminant analysis
• Understanding LDA algorithm
o Prerequisite
o Fisher’s linear discriminant analysis
o Linear Algebra Explanation
• Dive into the Advanced linear discriminant analysis algorithm
o Statistical Explanation
o linear discriminant analysis compared with principal component analysis
o Quadratic Discriminant Analysis
• Implementing linear discriminant analysis algorithm
o Using LDA with Scikit-Learn
• LDA Parameter and Attribute in Scikit-Learn
o Parameter options
o Attributes options
o Worked example of linear discriminant analysis algorithm for dimensionality
o Plotting Decision boundary for Mnist dataset
o Fitting LDA algorithm on MNIST Dataset
o Future linear discriminant analysis algorithm
• Conclusion
Part 3: Non-Linear Methods for Dimension Reduction
6 – Linear Local Embedding
• Introduction
o What is nonlinear dimensionality reduction?
o Why do we need nonlinear dimensionality reduction?
o What is embedding?
o Local linearity and manifolds
• LLE algorithm
o k-Nearest-Neighbors (kNN)
o Number of neighbors in kNN algorithm
o Finding weights
o Finding coordinates
• Variations of LLE
o Inverse LLE
o Kernel LLE
o Incremental LLE
o Robust LLE
o Weighted LLE
o Landmark LLE for big data (Nystrom/LLL)
o Supervised and semi-supervised LLE
o LLE with other manifold learning methods
• Implementation and use cases
o How to implement LLE algorithms in Python?
o How to use LLE algorithms for dimensionality reduction in datasets?
o Comparing the performance of LLE algorithms
o Face recognition by LLE algorithms
• Conclusion
7 – Multi-dimensional Scaling
• Basics of Multi-dimensional Scaling
o Introduction to MDS
o Data in MDS
o Proximity and Distance
• MDS models
o Metric MDS
o Trogerson’s Method
o Non-Metric MDS
o The goodness of Fit
o Individual Differences Models
o INDSCAL
o Tucker-Messick Model
o PINDIS
o Unfolding Models
o Non-metric Uni-dimensional Scaling
• Applications of MDS
o Localization
o MDS in psychology
• Conclusion
8 – t-distributed Stochastic Neighbor Embedding
• Introduction to t-SNE
o What is t-SNE?
o Why is t-SNE useful?
o Applications of t-SNE
• Understanding the t-SNE algorithm
o The t-SNE perplexity parameter
o The t-SNE objective function
o The t-SNE learning rate
o Implementing t-SNE in practice
• Visualizing high-dimensional data with t-SNE
o Visualizing high-dimensional data with t-SNE
o Choosing the right number of dimensions
o Interpreting t-SNE plots
• Advanced t-SNE techniques
o Using t-SNE for data clustering
o Combining t-SNE with other dimensionality reduction methods
• Conclusion
Part 4: Deep Learning Methods for Dimension Reduction
9 – Feature Extraction and Deep Learning
• The Revolutionary History of Deep Learning: From Biology to Simple Perceptron and Beyond
o A Brief History
o Biological Neurons
o Artificial Neurons: The Perceptron
• Deep Neural Networks
o Deep Feedforward Networks
o Convolutional Networks
• Learned Features
o Neural Networks and Representation Learning
o Visualizing Learned Features
o Deep Feature Extraction
o Deep Feature Extraction Applications
• Case Studies and examples
o Benchmark Datasets
o Feature Selection Using CNN
o RNN Feature Representation
o Feature Representing Using Other types DNN
• Conclusion
10 – Autoencoders
• Introduction to autoencoders
o Generative Modeling
o Traditional autoencoders
o Mathematics Principles
• Autoencoders for feature extraction
o Latent Variable
o Representation Learning
o Feature Learning Approaches
o Learned Features Applications
• Types of autoencoders
o Denoising Autoencoder
o Contractive Autoencoder
o Convolutional Autoencoder
o Variational Autoencoder
• Practical Approach
o Data Perspective
o Implementation Approaches
o Learning Task Case Studies
o Limitations and Challenges
• Performance Comparison
o Evaluation Metrics and Benchmark Datasets
o A Benchmark Study on ML Problems
o A Benchmark Study on Computer Vision Problems
o A Benchmark Study on Time Series Problems
• Conclusion
11 – Dimensionality reduction in deep learning through group actions
• Introduction
o Background on the need for efficient processing of highdimensional data.
o Overview of deep learning and dimensionality reduction techniques.
o Motivation for using geometric deep learning in dimensionality reduction.
• Group actions in geometric deep learning
o Overview of geometric deep learning.
o Symmetry, invariance, and equivariant neural networks.
o Explanation of group actions, their relevance, and examples in geometric learning.
o Overview of the unified model for group actions in dimensionality reduction.
• Examples of group structures and actions in geometric deep learning
o Several examples of group structures and actions for dimensionality reduction in deep learning (including new ones such as architecture, quantum computing, etc.).
o Visual and mathematical illustrations to aid in understanding the concept of group actions (new example implementation by a student and experimental results).
• Conclusion
o Summary of the main concepts covered in the chapter.
o Implications of using geometrical concepts in dimensionality reduction in deep learning.
o Discussion on limitation of current group structure and the potential for generalizing for more effective dimensionality reduction (example for correlated data and also blood group).
- Edition: 1
- Published: February 5, 2025
- Imprint: Morgan Kaufmann
- Language: English
JR
Jamal Amani Rad
Dr. Jamal Amani Rad currently works in Choice Modelling Centre and Institute for Transport Studies, University of Leeds, Leeds LS2 9JT, UK He obtained his PhD in Mathematics at the Department of Mathematics at University of Shahid Beheshti. His research interests include modelling, numerics, and analysis of partial differential equations by using meshless methods, with an emphasis on applications from finance.
SC
Snehashish Chakraverty
Dr. Snehashish Chakraverty is a Senior Professor in the Department of Mathematics (Applied Mathematics Group), National Institute of Technology Rourkela, with over 30 years of teaching and research experience. A gold medalist from the University of Roorkee (now IIT Roorkee), he earned his Ph.D. from IIT Roorkee and completed post-doctoral work at the University of Southampton (UK) and Concordia University (Canada). He has also served as a visiting professor in Canada and South Africa. Dr. Chakraverty has authored/edited 38 books and published over 495 research papers. His research spans differential equations (ordinary, partial, fractional), numerical and computational methods, structural and fluid dynamics, uncertainty modeling, and soft computing techniques. He has guided 27 Ph.D. scholars, with 10 currently under his supervision.
He has led 16 funded research projects and hosted international researchers through prestigious fellowships. Recognized in the top 2% of scientists globally (Stanford-Elsevier list, 2020–2024), he has received numerous awards including the CSIR Young Scientist Award, BOYSCAST Fellowship, INSA Bilateral Exchange, and IOP Top Cited Paper Awards. He is Chief Editor of International Journal of Fuzzy Computation and Modelling and serves on several international editorial boards.
KP