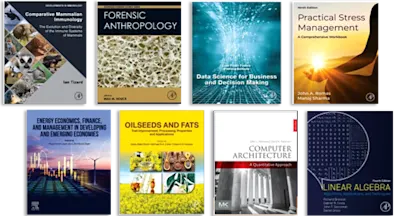
Digital Twins for Smart Cities and Villages
- 1st Edition - October 18, 2024
- Imprint: Elsevier
- Editors: Sailesh Iyer, Anand Nayyar, Anand Paul, Mohd Naved
- Language: English
- Paperback ISBN:9 7 8 - 0 - 4 4 3 - 2 8 8 8 4 - 5
- eBook ISBN:9 7 8 - 0 - 4 4 3 - 2 8 8 8 5 - 2
Digital Twins for Smart Cities and Villages provides a holistic view of digital twin technology and how it can be deployed to develop smart cities and smart villages. Smart manufa… Read more
Purchase options
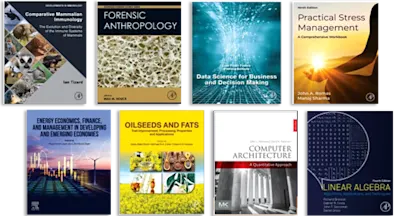
The book is thoughtfully structured, starting from the background of digital twin concepts and basic know-how to serve the needs of those new to the subject. It continues with implementation to facilitate and improve management in several urban contexts, infrastructures, and more. Global case study assessments further provide a deep characterization of the state-of-the-art in digital twin in urban and rural contexts.
- Uniquely focuses on applications for smart cities and villages, including smart services for health, education, mobility, and agriculture
- Provides use cases and practical deployment of research involved in the emerging uses of digital twins
- Discusses all pertinent issues, challenges, and possible solutions instrumental in implementing digital twins smart solutions in this context
- Edited and authored by a global team of experts in their given fields
2. Research advancements in quantum computing digital twins
3. Digital Twins Tools and Technologies
4. Future trends and research challenges in digital twins
5. Research advancements in quantum computing and digital twins
6. Digital twin model design for smart village
7. Digital village analytics using digital twins
8. Toward seamless mobility: Integrating connected and autonomous vehicles in smart cities through digital twins
9. Redefining mobility: The convergence of autonomy, technology, and connected vehicles in smart cities
10. Planning and building digital twins for smart cities
11. A dashboard framework for decision support in smart cities
12. Immersive learning trends using digital twins
13. Digital Twin and Virtual Reality, Augmented Reality, and Mixed Reality
14. Digital twins for telemedicine and personalized medicine
15. Digital twin technology in smart agriculture: Enhancing productivity and sustainability
16. Digital twins solutions for smart logistics and transportation
17. Exploring virtual smart healthcare trends using digital twins
18. Harmonizing nature and technology: The synergy of digital twin-enabled smart farming
19. Design for digital twins in smart manufacturing
20. Digital twins-enabled model for smart farming
21. Electrical digital twins-enabled smart grid
22. Digital twins in microclimate analysis: A mixed review using a science mapping approach
23. Data analytics and visualization using digital twins
24. Research trends in blockchain and digital twins
25. Blockchain-based digital twin for supply chain management: A survey
26. Rise of blockchain based digital twin: A transformative tool for new research trends
27. Exploring diverse use cases of digital twins projecting digital transformation: Unlocking potential, addressing challenges and viable solutions
- Edition: 1
- Published: October 18, 2024
- Imprint: Elsevier
- Language: English
SI
Sailesh Iyer
Dr. Sailesh Iyer has a Ph.D. (Computer Science), pursuing Post Doc from University of Louisiana, Lafayette, USA, and currently serving as a Professor with Rai University, Ahmedabad. He has more than 23 years of experience in academics, industry and corporate training. He has been awarded a Research Excellence Award for 2021 by Rai University and an Honorary Adjunct Research Scientist at Neurolabs International under Dana Brain Health Institute, Iran, from August 2022 to August 2025. He is an editor for book projects with various international publishers. He has been invited as keynote speaker in various international conferences. He has excelled in corporate training, delivered more than 100 expert talks in various AICTE sponsored STTP’s, ATAL FDP’s, reputed universities, government organized workshops, orientations and refresher courses. His research interest areas include computer vision and image processing, cybersecurity, data mining and analytics, artificial intelligence, machine learning, and blockchain.
AN
Anand Nayyar
Dr. Anand Nayyar received his Ph.D (Computer Science) from Desh Bhagat University in 2017 in Wireless Sensor Networks and Swarm Intelligence. He is currently working in Graduate School, Faculty of Information Technology- Duy Tan University, Vietnam. He has published numerous research papers in various high-impact journals and holds 10 Australian patents and 1 Indian Design to his credit in the area of Wireless Communications, Artificial Intelligence, IoT and Image Processing.
AP
Anand Paul
Anand Paul is an Associate Professor with the Biostatistics and Data Science Program at Louisiana State University Health Science Center. He obtained his Ph.D. degree from the School of Electrical and Computer Engineering at National Cheng Kung University, Taiwan, R.O.C. in 2010. His research focuses on Big Data Analytics and Mathematical Modelling of Machine Learning models, He has done extensive work on Big data/IoT based Smart Cities. Dr. Paul is the founder and director of the Centre for Resilient and Evolving Intelligence at Kyungpook National University, South Korea, where he served from 2012 to 2024. He has been recognized as one of the top 2% scientists globally by both Stanford University and Elsevier Publisher for the years 2021 and 2022. He has been an IEEE Senior Member since 2015 and has held editorial roles in prestigious publications including Editor in Chief of the International Journal of Smart Vehicles and Smart Transportation (IGI Global) from 2019 to 2021, and as Associate Editor in IEEE Access, IET Wireless Sensor Systems, ICT Express, PeerJ Computer Science, ACM Applied Computing Reviews, Cyber Physical Systems (Taylor & Francis), and International Journal of Interactive Multimedia & Artificial Intelligence. Dr. Paul also served as the track chair for Smart Human-Computer Interaction in ACM SAC from 2014 to 2019.
MN
Mohd Naved
Dr. Mohd Naved is an Associate Professor at Jaipuria Institute of Management in Noida, India, with over a decade of experience in Business Analytics, Data Science, and Artificial Intelligence. His research focuses on the applications of business analytics, data science, and artificial intelligence across various industries.