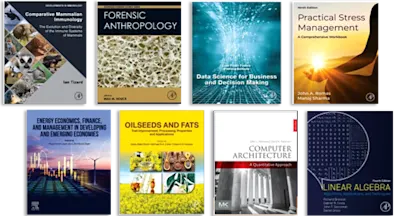
Diagnosing Musculoskeletal Conditions using Artificial Intelligence and Machine Learning to Aid Interpretation of Clinical Imaging
- 1st Edition - November 16, 2024
- Imprint: Academic Press
- Editors: Rakesh Kumar, Meenu Gupta, Ajith Abraham, Paul Antony
- Language: English
- Paperback ISBN:9 7 8 - 0 - 4 4 3 - 3 2 8 9 2 - 3
- eBook ISBN:9 7 8 - 0 - 4 4 3 - 3 2 8 9 3 - 0
Bone deformations can lead to musculoskeletal disorders and negatively impact on individual quality of life. Early and accurate detection of bone deformation is crucial for… Read more
Purchase options
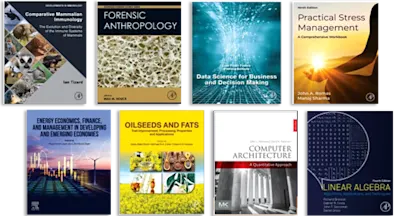
- Presents fundamental concepts and analysis of machine learning algorithms and diagnoses in bone deformation
- Addresses human health issues related to bone deformation using a different machine learning algorithm
- Provides innovative, new approaches for machine learning in musculoskeletal conditions with its future directions
2. Identification and Classification of Musculoskeletal Conditions using Artificial Intelligence and Machine Learning
3. Early-stage detection of osteoarthritis of the joints (hip and knee) using Machine Learning
4. Bone cancer classification and detection using Machine Learning techniques
5. Identification of the risk of osteoporosis in older Vietnamese women using Artificial Intelligence and Machine Learning
6. Identification of Paget's Disease in the Human body using Artificial Intelligence and Machine Learning
7. Identification of Osteonecrosis using Artificial Intelligence and Machine Learning
8. Identification and Classification of Rheumatoid Arthritis using Artificial Intelligence and Machine Learning
9. Early detection approach for Analysis of Osteoarthritis using Artificial Intelligence and Machine Learning
10. Pre-analysis of ankylosing spondylitis using Machine Learning
11. Analysis and identification of gout flares using Machine Learning
12. Impact of Amyloidosis on Bones and its relationship with dementia
13. Real-world application of Machine learning in Bone Deformities identification
14. Conclusion
- Edition: 1
- Published: November 16, 2024
- Imprint: Academic Press
- Language: English
RK
Rakesh Kumar
Dr. Rakesh Kumar is working as a Professor in the Department of Computer Science Engineering at Chandigarh University, Punjab, India. He did his Ph.D. from Punjab Technical University, Jalandhar, in Computer Science and Engineering in 2017. He has published many authored books with reputed publishers. His research interests are IoT, Machine Learning, and Natural Language Processing. A total of 19+ Years of Academic / Research Experience with more than 100 Publications in various National/International Conferences and Journals. He also organized three international conferences under the banner of IEEE Explore and AIP publisher.
MG
Meenu Gupta
Dr. Meenu Gupta is an Associate Professor at the UIE-CSE Department, Chandigarh University, India. She completed her Ph.D. in Computer Science and Engineering with an emphasis on Traffic Accident Severity Problems from Ansal University, Gurgaon, India, in 2020. She has more than 15 years of teaching experience. Her research areas cover Machine Learning, Intelligent Systems, and Data mining, with a specific interest in Artificial Intelligence, Image Processing and Analysis, Smart Cities, Data Analysis, and Human/Brain-machine Interaction (BMI). She has five edited and four authored books. She has also authored or co-authored more than 20 book chapters and over 80 papers in refereed international journals and conferences. She has five filled patents and was awarded the best faculty and department researcher in 2021 and 2022.
AA
Ajith Abraham
Dr. Ajith Abraham is the Vice Chancellor at Sai University, Chennai. Before joining Sai University, he held the position of vice chancellor at prominent institutions and was also the founding director of Machine Intelligence Research Labs (MIR Labs), a non-profit scientific network for innovation and research excellence with headquarters in Seattle, USA. Dr. Abraham has completed research projects valued at over $110 million as an investigator or co-investigator from the United States, the European Union, Italy, the Czech Republic, France, Malaysia, China, and Australia. He has worked in a multidisciplinary setting for more than 35 years and has authored or co-authored more than 1,500+ research publications in artificial intelligence and related applications in the industry. A handful of his publications have been translated into Chinese and Russian, and one of his books has been translated into Japanese. The Scopus database has approximately 1,400 papers indexed, whereas the Thomson Web of Science has over 1,000 publications indexed.
In addition to other esteemed universities, Dr. Abraham has worked with researchers from MIT (USA), the University of Cambridge (UK), Harvard University (USA), and Oxford University (UK). According to Google Scholar, Dr. Abraham possesses over 63,000 scholarly citations with an H-index of over 118. He has delivered over 250 conference plenary talks and tutorials in more than 20 countries. From 2008 to 2021, Dr. Abraham chaired the IEEE Systems, Man, and Cybernetics Society Technical Committee on Soft Computing, which had more than 200 members. From 2011 to 2013, he represented Europe as a Distinguished Lecturer for the IEEE Computer Society (USA). Dr. Abraham is continuously listed in the Stanford/Elsevier list, highlighting the top 2% of the most cited scientists across the globe. Based on 2024 data, ScholarGPS listed Dr. Abraham as one of the world’s top 0.01% cited scientists in the engineering and computer science fields.
From 2016 to 2021, Dr. Abraham worked as the chief editor of Engineering Applications of Artificial Intelligence (EAAI) at Elsevier, New York. EAAI is one of the oldest journals (founded in 1988) in the artificial intelligencedomain. Additionally, he sat on the editorial boards of more than 15 international journals indexed by Thomson ISI. Dr. Abraham received his Ph.D. degree in artificial intelligence from Monash University, Melbourne, Australia (2001), a Master of Science degree from Nanyang Technological University, Singapore (1998), and a B.Tech (Hons) degree from the University of Calicut in 1990.
PA
Paul Antony
Dr. Paul Antony is working as Senior and Lead consultant in Department of Orthopaedics at Apollo Super speciality Hospital Muscat, Oman. He did his postgraduate degree from Christian Medical college, Ludhiana from Panjab University in 1998 and did his SICOT & AO fellowship in shoulder and knee surgeries from Germany . He has more than 24 years experience in the field of arthroplasty and arthroscopic surgeries of shoulder, knee and hip joints.