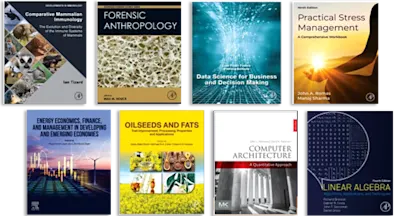
Deep Learning in Genetics and Genomics
Volume 1: Foundations and Introductory Applications
- 1st Edition - November 28, 2024
- Imprint: Academic Press
- Editor: Khalid Raza
- Language: English
- Paperback ISBN:9 7 8 - 0 - 4 4 3 - 2 7 5 7 4 - 6
- eBook ISBN:9 7 8 - 0 - 4 4 3 - 2 7 5 7 5 - 3
Deep Learning in Genetics and Genomics vol. 1, Foundations and Applications, the intersection of deep learning and genetics opens up new avenues for advancing our unders… Read more
Purchase options
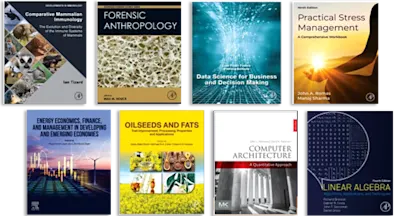
- Brings together fundamental concepts of genetics, genomics, and deep learning
- Includes how to build background of solution methodologies and design of mathematical and logical algorithms
- Delves into the intersection of deep learning and genetics, offering a comprehensive exploration of how deep learning techniques can be applied to various aspects of genomics
2. Introduction to Deep Learning
3. Decoding DNA: Deep Learning’s Impact on Genomic Exploration
4. Deep learning for Biological Data analysis
5. AI and Deep Learning in Cancer Genomics
6. Unravelling the Recent Developments in Applications and Challenges of AI in Cancer Biology: An overview
7. Unlocking the Potential of Deep Learning for Oncological Sequence Analysis: A Review
8. Deep Learning in Medical Genetics: A Review
9. Navigating the Genomic Landscape: A Deep Dive into Clinical Genetics with Deep Learning
10. Advancing Clinical Genomics: Bridging the Gap between Deep Learning Models and Interpretability for Improved Decision Support
11. Deep Learning in Clinical Genomics-based Diagnosis of Cancers
12. Predictive Precision: Unleashing Deep Learning in Comprehensive Medical Diagnosis and Treatment
13. Deep Learning in Predictive Medicine: Current State-of-the-art
14. Applications of AI in Cancer Genomics: A Way toward Intelligent Decision Systems in Healthcare
15. The Role of Deep Learning in Drug Discovery
16. Deep Learning in Drug Discovery: Revolutionizing the Path to New Medicines
17. Deep Learning Application in Genomics-based Toxicology Assessment
18. The Revolutionary Impact of Deep Learning in Transcriptomics and Gene Expression Analysis: A Genomic Paradigm Shift
19. Machine Learning Applications in Biological Network Analysis: Current State-of-the-Art
20. Data-driven Genomics: A Triad of Big Data, Cloud, and IoT in Genomics Research
21. Deep Learning in Variant Detection and Annotation
- Edition: 1
- Published: November 28, 2024
- Imprint: Academic Press
- Language: English
KR
Khalid Raza
Dr. Khalid Raza is working as an Associate Professor at the Department of Computer Science, Jamia Millia Islamia, New Delhi. Earlier he worked as an “ICCR Chair Professor” at Ain Shams University, Cairo, Egypt. He has many years of teaching & research experiments in the field of Translational Bioinformatics and Computational Intelligence. He has contributed over 120 research articles in reputed Journals and Edited Books. Dr. Raza has authored/edited dozens of books published by reputed publishers. Dr. Raza is an Academic Editor of PeerJ Computer Science International Journal, and Guest Editor of the Journal Natural Product Communications. He has an active collaboration with the scientists from leading institutions of India and abroad. Recently, Dr. Raza has been featured in the list of Top 2% Scientists released by Stanford University (USA) in collaboration with Elsevier. His research interest lies in Machine Learning and its applications in Bioinformatics and Health-informatics.