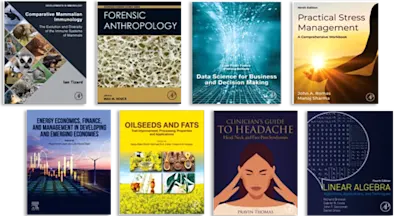
Deep Learning in Genetics and Genomics
Volume 2: Advanced Applications
- 1st Edition - November 28, 2024
- Imprint: Academic Press
- Editor: Khalid Raza
- Language: English
- Paperback ISBN:9 7 8 - 0 - 4 4 3 - 2 7 5 2 3 - 4
- eBook ISBN:9 7 8 - 0 - 4 4 3 - 2 7 5 2 4 - 1
Deep Learning in Genetics and Genomics: Vol. 2 (Advanced Applications) delves into the Deep Learning methods and their applications in various fields of studies, including geneti… Read more
Purchase options
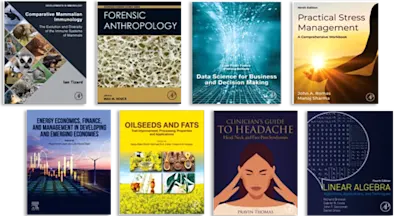
Institutional subscription on ScienceDirect
Request a sales quote- Embraces the potential that deep learning holds for understanding genome biology
- Encourages further advances in this area, extending to all aspects of genomics research
- Provides Deep Learning algorithms in genetic and genomic research
2. Deep Learning in Predictive Medicine Exemplified by AI-Mediated Flu Surveillance in USA
3. Towards Equitable Precision Medicine: Investigating the Transferability of Deep Learning Models in Clinical Genetics across Diverse Populations
4. Analysis of Genetic and Clinical Features in Neuro Disorders Using Deep Learning Models
5. Deep Learning Insights into Transcriptomics and Gene Expression Patterns analysis
6. Role of AI and Deep Learning in Clinical Cancer Genomics Allowing Targeted Therapies for Oncology
7. Deep Learning Approaches for Interpreting Non-coding Regions in Ovarian Cancer
8. Advancements in AI-driven Spatial Transcriptomics: Decoding Cellular Complexity
9. Neural Architectures for Genomic Understanding: Deep Dive into Epigenome and Chromatin Structure 10. Deep Learning in Personalized Genomics and Gene Editing
11. Deep Learning-based Model for Prediction of Prognostic Genes of Breast Cancer using Transcriptomic data
12. Deciphering the Complexity of Life: Advances in Genomic Image Analysis
13. Qualitative Study on Steganography of Genomic Image Data for Secure Data Transmission Using Deep Learning Models
14. Generative AI in Genetics: A Comprehensive Review
15. Integrating Computational Biology and Multi-Omics Data for Precision Medicine in Personalized Cancer Treatment
16. Deep Generative Models in Utilitarian and Metamorphic Genomics - Intellectual Benefits
17. Transfer Learning in High-Dimensional Genomic Data Analysis
18. Inequality in Genetic Healthcare: Bridging Gaps with Deep Learning Innovations in LMICs
19. Harmonizing Health Horizons: Bridging Research Gaps in Big Data Management for Transformative Clinical Insights
20. Bridging the Gap: Understanding Genetic Discoveries through Explainable AI
21. Explainable AI in Genetics: A Case Study
22. Deep Learning in Predicting Genetic Disorders: A Case Study
23. AI and Deep Learning in Single-Cell Omics Data Analysis: A Case Study
24. Deep Learning for Network Building and Network Analysis of Biological networks: A Case Study
25. Transformer Networks and Autoencoders in Genomics and Genetic Data Interpretation: A Case Study
- Edition: 1
- Published: November 28, 2024
- No. of pages (Paperback): 468
- Imprint: Academic Press
- Language: English
- Paperback ISBN: 9780443275234
- eBook ISBN: 9780443275241
KR
Khalid Raza
Dr. Khalid Raza is working as an Associate Professor at the Department of Computer Science, Jamia Millia Islamia, New Delhi. Earlier he worked as an “ICCR Chair Professor” at Ain Shams University, Cairo, Egypt. He has many years of teaching & research experiments in the field of Translational Bioinformatics and Computational Intelligence. He has contributed over 120 research articles in reputed Journals and Edited Books. Dr. Raza has authored/edited dozens of books published by reputed publishers. Dr. Raza is an Academic Editor of PeerJ Computer Science International Journal, and Guest Editor of the Journal Natural Product Communications. He has an active collaboration with the scientists from leading institutions of India and abroad. Recently, Dr. Raza has been featured in the list of Top 2% Scientists released by Stanford University (USA) in collaboration with Elsevier. His research interest lies in Machine Learning and its applications in Bioinformatics and Health-informatics.