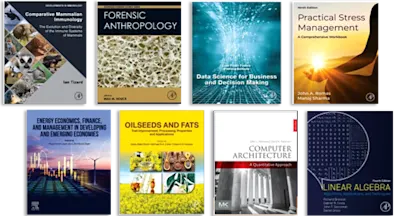
Deep Learning in Drug Design
Methods and Applications
- 1st Edition - October 1, 2025
- Imprint: Academic Press
- Editors: Qifeng Bai, Tingyang Xu, Junzhou Huang
- Language: English
- Paperback ISBN:9 7 8 - 0 - 4 4 3 - 3 2 9 0 8 - 1
- eBook ISBN:9 7 8 - 0 - 4 4 3 - 3 2 9 0 9 - 8
Deep Learning in Drug Design: Methods and Applications summarizes the most recent methods, and technological advances of deep learning for drug design, which mainly consists of mol… Read more
Purchase options
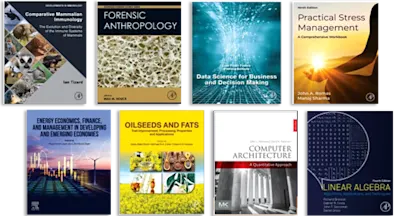
Deep Learning in Drug Design: Methods and Applications summarizes the most recent methods, and technological advances of deep learning for drug design, which mainly consists of molecular representations, the architectures of deep learning, geometric deep learning, large models, etc., as well as deep learning applications in various aspects of drug design. This book offers a comprehensive academic overview of deep learning in drug design. It begins with molecular representations, CNNs, GNNs, Transformers, generative models, explainable AI, large models, etc. Next, it covers deep learning applications like protein structure prediction, molecular interactions, ADMET prediction, antibody design, and so on. Finally, a separate chapter is dedicated to the introduction of the ethics and regulation of artificial intelligence in drug design. This book is ideal for readers aiming to learn and implement deep learning methods and applications in drug design and related fields.
Deep Learning in Drug Design: Methods and Applications is particularly helpful to undergraduate, graduate, and doctoral students in need of a practical guide to the principles of the discipline. Established researchers in the area will benefit from the detailed case studies and algorithms presented.
Deep Learning in Drug Design: Methods and Applications is particularly helpful to undergraduate, graduate, and doctoral students in need of a practical guide to the principles of the discipline. Established researchers in the area will benefit from the detailed case studies and algorithms presented.
- Introduces the basic theories, current methods, and principles of deep learning for drug design
- Presents the major application fields of drug design based on deep learning including protein folding, retrosynthesis prediction, molecular generation, molecular docking, and ADMET prediction, among others
- Details explainable artificial intelligence for drug design models
Undergraduates, graduate students, doctoral students, postdoctoral students, and professors who would like to understand deep learning and artificial intelligence for drug design may be applied to a range of research topics in pharmaceutical science, biochemistry, mathematics and computing, and chemistry
Part 1: Deep Learning Theories and Methods for Drug Design
1. Molecular Representations in Deep Learning
2. CNNs in Drug Design
3. GNNs in Drug Design
4. RNNs and LSTM in Drug Design
5. Deep Reinforcement Learning in Drug Design
6. Transformer and Drug Design
7. Generative Models for Drug Design
8. Geometric Graph Learning for Drug Design
9. Contrastive Learning and Pre-training Models for Drug Discovery
10. Transfer Learning, Knowledge Distillation, and Meta-Learning for Drug Discovery
11. Explainable Artificial Intelligence for Drug Design Models
12. Large Language Models for Drug Design
Part 2: Deep Learning Applications in Drug Design
13. Deep Learning for Protein Secondary Structure Prediction
14. Deep Learning in Protein Structure Prediction
15. Deep Learning in Molecular Interactions
16. Deep Learning in Chemical Synthesis and Retrosynthesis
17. Deep Learning for ADMET Prediction
18. Deep Learning for Toxicity Prediction
19. Deep Learning for TCR-pMHC Binding
20. Deep Learning for B Cell Epitope Prediction and Receptor
21. Deep Learning for Antigen-specific Antibody Design
1. Molecular Representations in Deep Learning
2. CNNs in Drug Design
3. GNNs in Drug Design
4. RNNs and LSTM in Drug Design
5. Deep Reinforcement Learning in Drug Design
6. Transformer and Drug Design
7. Generative Models for Drug Design
8. Geometric Graph Learning for Drug Design
9. Contrastive Learning and Pre-training Models for Drug Discovery
10. Transfer Learning, Knowledge Distillation, and Meta-Learning for Drug Discovery
11. Explainable Artificial Intelligence for Drug Design Models
12. Large Language Models for Drug Design
Part 2: Deep Learning Applications in Drug Design
13. Deep Learning for Protein Secondary Structure Prediction
14. Deep Learning in Protein Structure Prediction
15. Deep Learning in Molecular Interactions
16. Deep Learning in Chemical Synthesis and Retrosynthesis
17. Deep Learning for ADMET Prediction
18. Deep Learning for Toxicity Prediction
19. Deep Learning for TCR-pMHC Binding
20. Deep Learning for B Cell Epitope Prediction and Receptor
21. Deep Learning for Antigen-specific Antibody Design
- Edition: 1
- Published: October 1, 2025
- Imprint: Academic Press
- Language: English
QB
Qifeng Bai
Qifeng Bai is a professor in School of Basic Medical Sciences of Lanzhou University. He is also an associate editor in the journal named Frontiers in Chemistry. He is interested in drug design by developing new algorithms, software, machine learning, and deep learning. He is also good at conformation transition studies of receptors (e.g. kinases and G protein-coupled receptors) by performing molecular dynamics simulations. He has developed the software MolAICal which has been widely used to design drugs based on deep learning and traditional algorithms.
Affiliations and expertise
Lanzhou University, ChinaTX
Tingyang Xu
Tingyang Xu, a Senior Researcher at Tencent AI Lab's AI for Science Center, earned his Ph.D. from The University of Connecticut and his Bachelor's degree from Shanghai Jiaotong University. His research encompasses deep learning applications for de novo drug design, molecular property prediction, and molecular dynamics simulation, and he has significantly contributed to the development of advanced graph neural networks for tasks like subgraph recognition and node classification. His work has been published in top-tier data mining and machine learning conferences, including NeurIPS, ICML, SIGKDD, AAAI, VLDB, the Journal of Parallel and Distributed Computing (JPDC), Internet of Things (IoT), and Annuals of Surgery. Additionally, Dr. Xu has served as a reviewer for prestigious conferences and journals, including ICML, NeurIPS, KDD, WWW, AAAI, PR, and TKDE, and as the Industrial Track Chair for BIBM 2019.
Affiliations and expertise
Tencent AI Lab, ChinaJH
Junzhou Huang
Junzhou Huang is the Jenkins Garrett Professor in the Computer Science and Engineering department at the University of Texas at Arlington. He received the Ph.D. degree in Computer Science at Rutgers, the State University of New Jersey. His major research interests include machine learning, computer vision, medical image analysis, and bioinformatics. His research has been recognized by several awards including UT STARs Award, NSF CAREER Award, Google TensorFlow Model Garden Award, IBM Watson Emerging Leaders, four Best Paper Awards (MICCAI'10, FIMH'11, STMI'12, and MICCAI'15) as well as two Best Paper Nominations (MICCAI'11 and MICCAI'14). He is a Fellow of AIMBE.
Affiliations and expertise
University of Texas at Arlington, USA