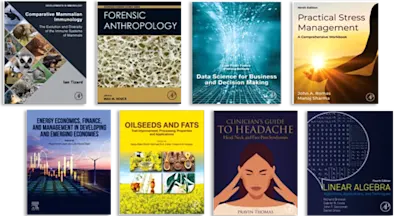
Deep Learning for Sustainable Agriculture
- 1st Edition - January 9, 2022
- Imprint: Academic Press
- Editors: Ramesh Chandra Poonia, Vijander Singh, Soumya Ranjan Nayak
- Language: English
- Paperback ISBN:9 7 8 - 0 - 3 2 3 - 8 5 2 1 4 - 2
- eBook ISBN:9 7 8 - 0 - 3 2 3 - 9 0 3 6 2 - 2
The evolution of deep learning models, combined with with advances in the Internet of Things and sensor technology, has gained more importance for weather forecasting, plant di… Read more
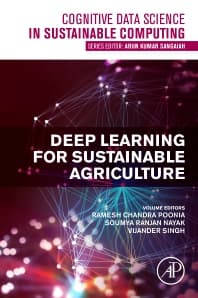
Purchase options
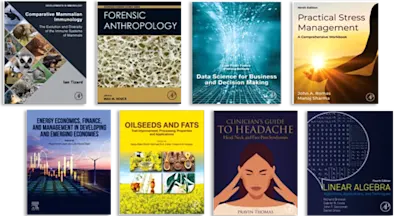
Institutional subscription on ScienceDirect
Request a sales quoteThe evolution of deep learning models, combined with with advances in the Internet of Things and sensor technology, has gained more importance for weather forecasting, plant disease detection, underground water detection, soil quality, crop condition monitoring, and many other issues in the field of agriculture. agriculture. Deep Learning for Sustainable Agriculture discusses topics such as the impactful role of deep learning during the analysis of sustainable agriculture data and how deep learning can help farmers make better decisions. It also considers the latest deep learning techniques for effective agriculture data management, as well as the standards established by international organizations in related fields. The book provides advanced students and professionals in agricultural science and engineering, geography, and geospatial technology science with an in-depth explanation of the relationship between agricultural inference and the decision-support amenities offered by an advanced mathematical evolutionary algorithm.
- Introduces new deep learning models developed to address sustainable solutions for issues related to agriculture
- Provides reviews on the latest intelligent technologies and algorithms related to the state-of-the-art methodologies of monitoring and mitigation of sustainable agriculture
- Illustrates through case studies how deep learning has been used to address a variety of agricultural diseases that are currently on the cutting edge
- Delivers an accessible explanation of artificial intelligence algorithms, making it easier for the reader to implement or use them in their own agricultural domain
Academics and Senior Graduates; university and faculty teachers, instructors and senior students in Computer Science working in the area of deep learning and agriculture. Environmentalists working in the field of agriculture science
- Cover image
- Title page
- Table of Contents
- Copyright
- Contributors
- Chapter 1: Smart agriculture: Technological advancements on agriculture—A systematical review
- Abstract
- 1: Introduction
- 2: Methodology
- 3: Role of image processing in agriculture
- 4: Role of Machine Learning in Agriculture
- 5: Role of deep learning in agriculture
- 6: Role of IoT in agriculture
- 7: Role of wireless sensor networks in agriculture
- 8: Role of data mining in agriculture
- 9: Conclusion
- References
- Chapter 2: A systematic review of artificial intelligence in agriculture
- Abstract
- 1: Precision farming
- 2: Plant disease detection
- 3: Soil health monitoring using AI
- 4: Scope and challenges of AI in agriculture
- 5: Conclusions
- References
- Chapter 3: Introduction to deep learning in precision agriculture: Farm image feature detection using unmanned aerial vehicles through classification and optimization process of machine learning with convolution neural network
- Abstract
- 1: Introduction
- 2: Deep learning overview
- 3: CNN training
- 4: Methodology
- 5: Experiment and results
- 6: Discussion
- 7: Conclusion
- References
- Chapter 4: Design and implementation of a crop recommendation system using nature-inspired intelligence for Rajasthan, India
- Abstract
- 1: Introduction
- 2: Literature survey
- 3: Proposed methodology
- 4: Results
- 5: Conclusion and future work
- References
- Further reading
- Chapter 5: Artificial intelligent-based water and soil management
- Abstract
- 1: Introduction
- 2: Applications of artificial intelligence in water management
- 3: Applications of artificial intelligence in soil management
- 4: Conclusion and recommendations for water-soil management
- References
- Chapter 6: Machine learning for soil moisture assessment
- Abstract
- 1: Introduction
- 2: Overview of machine learning
- 3: Machine learning algorithms applied in soil moisture research
- 4: Applications of machine learning for soil moisture assessment
- 5: Conclusions
- References
- Chapter 7: Automated real-time forecasting of agriculture using chlorophyll content and its impact on climate change
- Abstract
- 1: Introduction
- 2: Current status
- 3: Problem statement
- 4: Objective of the proposed work
- 5: Research highlights
- 6: Scientific significance of the proposed work
- 7: Materials and methods
- 8: Detailed work plan to achieve the objectives
- 9: Results and discussion
- 10: Conclusion
- References
- Chapter 8: Transformations of urban agroecology landscape in territory transition
- Abstract
- 1: Introduction
- 2: Agroecological landscapes
- 3: Agroecological practices
- 4: Agroecological territorial transformation and transition
- 5: Conclusion
- References
- Chapter 9: WeedNet: A deep neural net for weed identification
- Abstract
- 1: Introduction
- 2: Related work
- 3: WeedNet
- 4: Evaluation strategy
- 5: Experimental setup
- 6: Experimental evaluation
- 7: Conclusion
- References
- Chapter 10: Sensors make sense: Functional genomics, deep learning, and agriculture
- Abstract
- Acknowledgments
- 1: Introduction
- 2: Section I. Functional genomics
- 3: Section II. DAS networks
- 4: Section III. GRANITE and the agent-based GRANITE Network Discovery Tool
- 5: Conclusions
- References
- Chapter 11: Crop management: Wheat yield prediction and disease detection using an intelligent predictive algorithms and metrological parameters
- Abstract
- 1: Introduction
- 2: Literature review
- 3: Discussion
- 4: Conclusion and future scope
- References
- Chapter 12: Sugarcane leaf disease detection through deep learning
- Abstract
- 1: Introduction
- 2: Methodology
- 3: Experimentation
- 4: Results and discussion
- 5: Conclusion
- References
- Chapter 13: Prediction of paddy cultivation using deep learning on land cover variation for sustainable agriculture
- Abstract
- 1: Introduction
- 2: Applications of geospatial analytics for agriculture
- 3: Material analysis
- 4: System model design and implementation
- 5: System evaluation
- 6: Discussion
- 7: Conclusions
- References
- Chapter 14: Artificial intelligence-based detection and counting of olive fruit flies: A comprehensive survey
- Abstract
- Acknowledgments
- 1: Introduction
- 2: Literature survey of recognition systems
- 3: Evaluation and discussions
- 4: Conclusions
- References
- Index
- Edition: 1
- Published: January 9, 2022
- No. of pages (Paperback): 406
- No. of pages (eBook): 406
- Imprint: Academic Press
- Language: English
- Paperback ISBN: 9780323852142
- eBook ISBN: 9780323903622
RP
Ramesh Chandra Poonia
VS
Vijander Singh
SN
Soumya Ranjan Nayak
Dr. Soumya Ranjan Nayak now holds the position of Assistant Professor in the School of Computer Engineering at Kalinga Institute of Industrial Technology (KIIT) Deemed to be University, located in Odisha, India. He obtained a Doctor of Philosophy (Ph.D) and Master of Technology (M.Tech) in Computer Science and Engineering under a scholarship provided by the Ministry of Human Resource Development (MHRD) of the Government of India. These degrees were earned at CET, BPUT Rourkela, India. Prior to this, he completed a Bachelor of Technology (B. Tech) and a Diploma in Computer Science and Engineering. He has authored over 150 articles that have been published in reputable international journals and conferences such as Elsevier, Springer, World Scientific, IOS Press, Taylor & Francis, Hindawi, Inderscience, IGI Global, and others. These publications have undergone a rigorous peer-review process. In addition to the aforementioned accomplishments, the individual has authored 16 book chapters, published 6 books, and obtained 7 Indian patents (with 4 patents being granted). Furthermore, they have secured 4 International patents, all of which have been granted. The researcher's current areas of focus encompass medical picture analysis and classification, machine learning, deep learning, pattern recognition, fractal graphics, and computer vision. The author's writings have garnered over 1500 citations, with an h-index of 24 and an i10-index of 63, as reported by Google Scholar. Dr. Nayak holds the position of an associate editor for several esteemed academic journals, including the Journal of Electronic Imaging (SPIE), Mathematical Problems in Engineering (Hindawi), Journal of Biomedical Imaging (Hindawi), Applied Computational Intelligence and Soft Computing (Hindawi), and PLOS One. He is currently fulfilling the role of a guest editor for special issues of renowned academic journals such as Springer Nature, Elsevier, and Taylor & Franchise. He has been affiliated as a reviewer for numerous esteemed peer-reviewed journals, including Applied Mathematics and Computation, Journal of Applied Remote Sensing, Mathematical Problems in Engineering, International Journal of Light and Electron Optics, Journal of Intelligent and Fuzzy Systems, Future Generation Computer Systems, Pattern Recognition Letters, and others. He has additionally held the Technical Program Committee Member position for several conferences of significant worldwide recognition.