LIMITED OFFER
Save 50% on book bundles
Immediately download your ebook while waiting for your print delivery. No promo code needed.
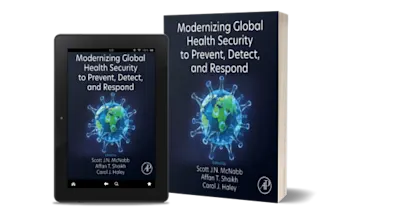
Data Mining: Practical Machine Learning Tools and Techniques, Fourth Edition, offers a thorough grounding in machine learning concepts, along with practical advice on applying… Read more
LIMITED OFFER
Immediately download your ebook while waiting for your print delivery. No promo code needed.
Data Mining: Practical Machine Learning Tools and Techniques, Fourth Edition, offers a thorough grounding in machine learning concepts, along with practical advice on applying these tools and techniques in real-world data mining situations. This highly anticipated fourth edition of the most acclaimed work on data mining and machine learning teaches readers everything they need to know to get going, from preparing inputs, interpreting outputs, evaluating results, to the algorithmic methods at the heart of successful data mining approaches.
Extensive updates reflect the technical changes and modernizations that have taken place in the field since the last edition, including substantial new chapters on probabilistic methods and on deep learning. Accompanying the book is a new version of the popular WEKA machine learning software from the University of Waikato. Authors Witten, Frank, Hall, and Pal include today's techniques coupled with the methods at the leading edge of contemporary research.
Please visit the book companion website at https://www.cs.waikato.ac.nz/~ml/weka/book.html.
It contains
Data analysts, data scientists, data architects. Business analysts, computer science students taking courses in data mining and machine learning
Part I: Introduction to data mining
Chapter 1. What’s it all about?
Chapter 2. Input: Concepts, instances, attributes
Chapter 3. Output: Knowledge representation
Chapter 4. Algorithms: The basic methods
Chapter 5. Credibility: Evaluating what’s been learned
Part II: More advanced machine learning schemes
Chapter 6. Trees and rules
Chapter 7. Extending instance-based and linear models
Chapter 8. Data transformations
Chapter 9. Probabilistic methods
Chapter 10. Deep learning
Chapter 11. Beyond supervised and unsupervised learning
Chapter 12. Ensemble learning
Chapter 13. Moving on: applications and beyond
Appendix A. Theoretical foundations
Appendix B. The WEKA workbench
IW
EF
MH
CP