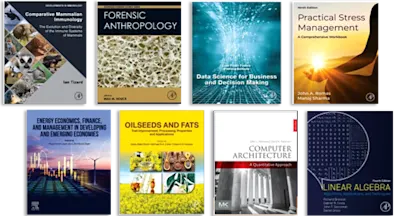
Data Architecture: A Primer for the Data Scientist
Big Data, Data Warehouse and Data Vault
- 1st Edition - November 26, 2014
- Imprint: Morgan Kaufmann
- Authors: W.H. Inmon, Daniel Linstedt
- Language: English
- Paperback ISBN:9 7 8 - 0 - 1 2 - 8 0 2 0 4 4 - 9
- eBook ISBN:9 7 8 - 0 - 1 2 - 8 0 2 0 9 1 - 3
Today, the world is trying to create and educate data scientists because of the phenomenon of Big Data. And everyone is looking deeply into this technology. But no one is lo… Read more
Purchase options
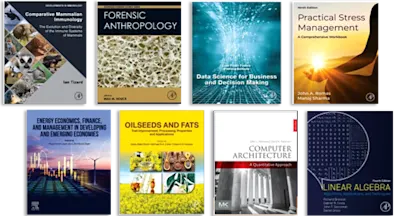
Today, the world is trying to create and educate data scientists because of the phenomenon of Big Data. And everyone is looking deeply into this technology. But no one is looking at the larger architectural picture of how Big Data needs to fit within the existing systems (data warehousing systems). Taking a look at the larger picture into which Big Data fits gives the data scientist the necessary context for how pieces of the puzzle should fit together. Most references on Big Data look at only one tiny part of a much larger whole. Until data gathered can be put into an existing framework or architecture it can’t be used to its full potential. Data Architecture a Primer for the Data Scientist addresses the larger architectural picture of how Big Data fits with the existing information infrastructure, an essential topic for the data scientist.
Drawing upon years of practical experience and using numerous examples and an easy to understand framework. W.H. Inmon, and Daniel Linstedt define the importance of data architecture and how it can be used effectively to harness big data within existing systems. You’ll be able to:
- Turn textual information into a form that can be analyzed by standard tools.
- Make the connection between analytics and Big Data
- Understand how Big Data fits within an existing systems environment
- Conduct analytics on repetitive and non-repetitive data
- Discusses the value in Big Data that is often overlooked, non-repetitive data, and why there is significant business value in using it
- Shows how to turn textual information into a form that can be analyzed by standard tools
- Explains how Big Data fits within an existing systems environment
- Presents new opportunities that are afforded by the advent of Big Data
- Demystifies the murky waters of repetitive and non-repetitive data in Big Data
Data Analysts, Business Intelligence and Data Warehousing Professionals, and Business Analysts
1.1: Corporate Data
- Abstract
- The Totality of Data Across the Corporation
- Dividing Unstructured Data
- Business Relevancy
- Big Data
- The Great Divide
- The Continental Divide
- The Complete Picture
1.2: The Data Infrastructure
- Abstract
- Two Types of Repetitive Data
- Repetitive Structured Data
- Repetitive Big Data
- The Two Infrastructures
- What’s being Optimized?
- Comparing the Two Infrastructures
1.3: The “Great Divide”
- Abstract
- Classifying Corporate Data
- The “Great Divide”
- Repetitive Unstructured Data
- Nonrepetitive Unstructured Data
- Different Worlds
1.4: Demographics of Corporate Data
- Abstract
1.5: Corporate Data Analysis
- Abstract
1.6: The Life Cycle of Data – Understanding Data Over Time
- Abstract
1.7: A Brief History of Data
- Abstract
- Paper Tape and Punch Cards
- Magnetic Tapes
- Disk Storage
- Database Management System
- Coupled Processors
- Online Transaction Processing
- Data Warehouse
- Parallel Data Management
- Data Vault
- Big Data
- The Great Divide
2.1: A Brief History of Big Data
- Abstract
- An Analogy – Taking the High Ground
- Taking the High Ground
- Standardization with the 360
- Online Transaction Processing
- Enter Teradata and Massively Parallel Processing
- Then Came Hadoop and Big Data
- IBM and Hadoop
- Holding the High Ground
2.2: What is Big Data?
- Abstract
- Another Definition
- Large Volumes
- Inexpensive Storage
- The Roman Census Approach
- Unstructured Data
- Data in Big Data
- Context in Repetitive Data
- Nonrepetitive Data
- Context in Nonrepetitive Data
2.3: Parallel Processing
- Abstract
2.4: Unstructured Data
- Abstract
- Textual Information Everywhere
- Decisions Based on Structured Data
- The Business Value Proposition
- Repetitive and Nonrepetitive Unstructured Information
- Ease of Analysis
- Contextualization
- Some Approaches to Contextualization
- MapReduce
- Manual Analysis
2.5: Contextualizing Repetitive Unstructured Data
- Abstract
- Parsing Repetitive Unstructured Data
- Recasting the Output Data
2.6: Textual Disambiguation
- Abstract
- From Narrative into an Analytical Database
- Input into Textual Disambiguation
- Mapping
- Input/Output
- Document Fracturing/Named Value Processing
- Preprocessing a Document
- Emails – A Special Case
- Spreadsheets
- Report Decompilation
2.7: Taxonomies
- Abstract
- Data Models and Taxonomies
- Applicability of Taxonomies
- What is a Taxonomy?
- Taxonomies in Multiple Languages
- Dynamics of Taxonomies and Textual Disambiguation
- Taxonomies and Textual Disambiguation – Separate Technologies
- Different Types of Taxonomies
- Taxonomies – Maintenance Over Time
3.1: A Brief History of Data Warehouse
- Abstract
- Early Applications
- Online Applications
- Extract Programs
- 4GL Technology
- Personal Computers
- Spreadsheets
- Integrity of Data
- Spider-Web Systems
- The Maintenance Backlog
- The Data Warehouse
- To an Architected Environment
- To the CIF
- DW 2.0
3.2: Integrated Corporate Data
- Abstract
- Many Applications
- Looking Across the Corporation
- More Than One Analyst
- ETL Technology
- The Challenges of Integration
- The Benefits of a Data Warehouse
- The Granular Perspective
3.3: Historical Data
- Abstract
3.4: Data Marts
- Abstract
- Granular Data
- Relational Database Design
- The Data Mart
- Key Performance Indicators
- The Dimensional Model
- Combining the Data Warehouse and Data Marts
3.5: The Operational Data Store
- Abstract
- Online Transaction Processing on Integrated Data
- The Operational Data Store
- ODS and the Data Warehouse
- ODS Classes
- External Updates into the ODS
- The ODS/Data Warehouse Interface
3.6: What a Data Warehouse is Not
- Abstract
- A Simple Data Warehouse Architecture
- Online High-Performance Transaction Processing in the Data Warehouse
- Integrity of Data
- The Data Warehouse Workload
- Statistical Processing from the Data Warehouse
- The Frequency of Statistical Processing
- The Exploration Warehouse
4.1: Introduction to Data Vault
- Abstract
- Data Vault 2.0 Modeling
- Data Vault 2.0 Methodology Defined
- Data Vault 2.0 Architecture
- Data Vault 2.0 Implementation
- Business Benefits of Data Vault 2.0
- Data Vault 1.0
4.2: Introduction to Data Vault Modeling
- Abstract
- A Data Vault Model Concept
- Data Vault Model Defined
- Components of a Data Vault Model
- Data Vault and Data Warehousing
- Translating to Data Vault Modeling
- Data Restructure
- Basic Rules of Data Vault Modeling
- Why We Need Many-to-Many Link Structures
- Hash keys Instead of Sequence Numbers
4.3: Introduction to Data Vault Architecture
- Abstract
- Data Vault 2.0 Architecture
- How NoSQL Fits into the Architecture
- Data Vault 2.0 Architecture Objectives
- Data Vault 2.0 Modeling Objective
- Hard and Soft Business Rules
- Managed SSBI and the Architecture
4.4: Introduction to Data Vault Methodology
- Abstract
- Data Vault 2.0 Methodology Overview
- CMMI and Data Vault 2.0 Methodology
- CMMI Versus Agility
- Project Management Practices and SDLC Versus CMMI and Agile
- Six Sigma and Data Vault 2.0 Methodology
- Total Quality Management
4.5: Introduction to Data Vault Implementation
- Abstract
- Implementation Overview
- The Importance of Patterns
- Reengineering and Big Data
- Virtualize Our Data Marts
- Managed Self-Service BI
5.1: The Operational Environment – A Short History
- Abstract
- Commercial Uses of the Computer
- The First Applications
- Ed Yourdon and the Structured Revolution
- System Development Life Cycle
- Disk Technology
- Enter the Database Management System
- Response Time and Availability
- Corporate Computing Today
5.2: The Standard Work Unit
- Abstract
- Elements of Response Time
- An Hourglass Analogy
- The Racetrack Analogy
- Your Vehicle Runs as Fast as the Vehicle in Front of It
- The Standard Work Unit
- The Service Level Agreement
5.3: Data Modeling for the Structured Environment
- Abstract
- The Purpose of the Road Map
- Granular Data Only
- The Entity Relationship Diagram
- The DIS
- Physical Database Design
- Relating the Different Levels of the Data Model
- An Example of the Linkage
- Generic Data Models
- Operational Data Models and Data Warehouse Data Models
5.4: Metadata
- Abstract
- Typical Metadata
- The Repository
- Using Metadata
- Analytical Uses of Metadata
- Looking at Multiple Systems
- The Lineage of Data
- Comparing Existing Systems to Proposed Systems
5.5: Data Governance of Structured Data
- Abstract
- A Corporate Activity
- Motivations for Data Governance
- Repairing Data
- Granular, Detailed Data
- Documentation
- Data Stewardship
6.1: A Brief History of Data Architecture
- Abstract
6.2: Big Data/Existing Systems Interface
- Abstract
- The Big Data/Existing Systems Interface
- The Repetitive Raw Big Data/Existing Systems Interface
- Exception-Based Data
- The Nonrepetitive Raw Big Data/Existing Systems Interface
- Into the Existing Systems Environment
- The “Context-Enriched” Big Data Environment
- Analyzing Structured Data/Unstructured Data Together
6.3: The Data Warehouse/Operational Environment Interface
- Abstract
- The Operational/Data Warehouse Interface
- The Classical ETL Interface
- The Operational Data Store/ETL Interface
- The Staging Area
- Changed Data Capture
- Inline Transformation
- ELT Processing
6.4: Data Architecture – A High-Level Perspective
- Abstract
- A High-Level Perspective
- Redundancy
- The System of Record
- Different Communities
7.1: Repetitive Analytics – Some Basics
- Abstract
- Different Kinds of Analysis
- Looking for Patterns
- Heuristic Processing
- The Sandbox
- The “Normal” Profile
- Distillation, Filtering
- Subsetting Data
- Filtering Data
- Repetitive Data and Context
- Linking Repetitive Records
- Log Tape Records
- Analyzing Points of Data
- Data Over Time
7.2: Analyzing Repetitive Data
- Abstract
- Log Data
- Active/Passive Indexing of Data
- Summary/Detailed Data
- Metadata in Big Data
- Linking Data
7.3: Repetitive Analysis
- Abstract
- Internal, External Data
- Universal Identifiers
- Security
- Filtering, Distillation
- Archiving Results
- Metrics
8.1: Nonrepetitive Data
- Abstract
- Inline Contextualization
- Taxonomy/Ontology Processing
- Custom Variables
- Homographic Resolution
- Acronym Resolution
- Negation Analysis
- Numeric Tagging
- Date Tagging
- Date Standardization
- List Processing
- Associative Word Processing
- Stop Word Processing
- Word Stemming
- Document Metadata
- Document Classification
- Proximity Analysis
- Functional Sequencing within Textual ETL
- Internal Referential Integrity
- Preprocessing, Postprocessing
8.2: Mapping
- Abstract
8.3: Analytics from Nonrepetitive Data
- Abstract
- Call Center Information
- Medical Records
9.1: Operational Analytics
- Abstract
- Transaction Response Time
10.1: Operational Analytics
- Abstract
11.1: Personal Analytics
- Abstract
12.1: A Composite Data Architecture
- Abstract
- Edition: 1
- Published: November 26, 2014
- Imprint: Morgan Kaufmann
- Language: English
WI
W.H. Inmon
DL