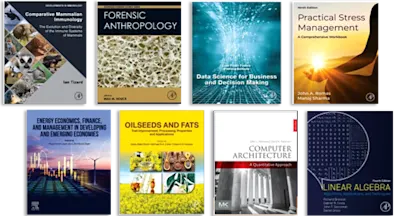
Cutting Edge Artificial Intelligence, Spatial Transcriptomics and Proteomics Approaches to Analyze Cancer
- 1st Edition, Volume 163 - September 12, 2024
- Imprint: Academic Press
- Editors: Kenneth D. Tew, Paul B. Fisher, Esha Madan, Rajan Gogna
- Language: English
- Hardback ISBN:9 7 8 - 0 - 4 4 3 - 2 9 6 5 0 - 5
- eBook ISBN:9 7 8 - 0 - 4 4 3 - 2 9 6 5 1 - 2
Cutting Edge Artificial Intelligence, Spatial Transcriptomics and Proteomics Approaches to Analyze Cancer, Volume 163 in the Advances in Cancer Research series, highlights ne… Read more
Purchase options
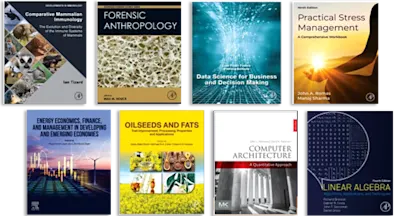
- Contains contributions that have been carefully selected based on their vast experience and expertise on the subject
- Includes updated, in-depth, and critical discussions of available information, giving the reader a unique opportunity to learn
- Encompasses a broad view of the topics at hand
2. Bioinformatics and bioactive peptides from foods: does it work together?
3. Food off-flavor volatiles generation, characterization and advances in novel strategies for mitigating off-flavor perception
4. Innovations in Food Packaging for a Sustainable and Circular economy
5. Upcycling of seafood side streams for circularity
6. Edible insects in foods
7. Effect of novel food processing technologies on Bacillus cereus spores
8. Salmonella spp. in poultry production – A review of the role of interventions along the production continuum
- Edition: 1
- Volume: 163
- Published: September 12, 2024
- Imprint: Academic Press
- Language: English
KT
Kenneth D. Tew
The Tew laboratory maintains an interest in using redox pathways as a platform to develop therapeutic strategies through drug discovery/development and biomarker identification. We interrogate how reactive oxygen and nitrogen species (ROS/RNS) impact cancer cells and develop novel drugs that impact on glutathione based pathways. Our research efforts have been integral to studies that have identified glutathione S-transferases (GST) as important in drug resistance, catalytic detoxification and as arbiters of kinase-mediated cell signaling events. In addition, we have been instrumental in defining how GSTP contributes to the process by which cells respond to ROS by selective addition of glutathione to specific protein clusters, so called S-glutathionylation. Each of these research areas has had broad impact on a number of cancer disciplines. Moreover, we have also been seminally involved in the Phase I to III clinical testing of three oncology drugs, Telcyta, Telintra and NOV-002. Other ongoing translational efforts have produced two ongoing clinical trials to measure the effectiveness of serum S-glutathionylated serine proteinase inhibitors as possible biomarkers for exposure to hydrogen peroxide mouthwashes and radiation.
PF
Paul B. Fisher
RG