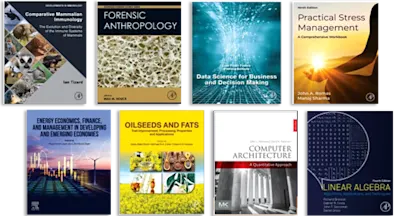
Computer Vision and Machine Intelligence for Renewable Energy Systems
- 1st Edition - September 20, 2024
- Imprint: Elsevier
- Editors: Ashutosh Kumar Dubey, Abhishek Kumar, Umesh Chandra Pati, Fausto Pedro Garcia Marquez, Vicente García-Díaz, Arun Lal Srivastav
- Language: English
- Paperback ISBN:9 7 8 - 0 - 4 4 3 - 2 8 9 4 7 - 7
- eBook ISBN:9 7 8 - 0 - 4 4 3 - 2 8 9 4 8 - 4
Computer Vision and Machine Intelligence for Renewable Energy Systems offers a practical, systemic guide to the use of computer vision as an innovative tool to support renewable… Read more
Purchase options
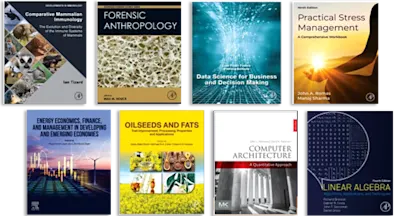
This book equips readers with a variety of essential tools and applications: Part I outlines the fundamentals of computer vision and its unique benefits in renewable energy system models compared to traditional machine intelligence: minimal computing power needs, speed, and accuracy even with partial data. Part II breaks down specific techniques, including those for predictive modeling, performance prediction, market models, and mitigation measures. Part III offers case studies and applications to a wide range of renewable energy sources, and finally the future possibilities of the technology are considered.
The very first book in Elsevier’s cutting-edge new series Advances in Intelligent Energy Systems, Computer Vision and Machine Intelligence for Renewable Energy Systems provides engineers and renewable energy researchers with a holistic, clear introduction to this promising strategy for control and reliability in renewable energy grids.
- Provides a sorely needed primer on the opportunities of computer vision techniques for renewable energy systems
- Builds knowledge and tools in a systematic manner, from fundamentals to advanced applications
- Includes dedicated chapters with case studies and applications for each sustainable energy source
1. An overview of renewable energy sources: technologies, applications and role of artificial intelligence
1.1 Introduction
1.2 Types of renewable energy sources
1.3 Role of artificial intelligence and machine learning in renewable energy system
1.4 Application of renewable energy sources
1.5 Advantages and disadvantages of renewable energy resources
1.6 Discussion
1.7 Conclusion
2. Artificial intelligence for renewable energy strategies and techniques
2.1 Introduction
2.2 Artificial intelligence in resource evaluation and forecasting
2.3 Advanced artificial intelligence techniques for renewable energy optimization artificial intelligence
2.4 Integrated framework: artificial intelligence integration in renewable energy strategies
2.5 Artificial intelligencebased predictive models for grid management
2.6 Practical applications and challenges of implementing artificial intelligence in renewable energy
2.7 Conclusion: illuminating the future of energy with artificial intelligence
3. Computer vision-based regression techniques for renewable energy: predicting energy output and performance
3.1 Introduction
3.2 Literature review
3.3 Methods
3.4 Results
3.5 Discussion
3.6 Conclusion and future work
4. Utilization of computer vision and machine learning for solar power prediction
4.1 Introduction
4.2 Solar power prediction overview
4.3 Computer vision in solar power prediction
4.4 Machine learning models for solar power prediction
4.5 Integration of computer vision and machine learning
4.6 Real-world applications and case studies
4.7 Discussion
4.8 Conclusions and future work
5. Exploring data-driven multivariate statistical models for the prediction of solar energy
5.1 Introduction
5.2 Related prior work
5.3 Solar energy forecasting framework
5.4 Results and analysis
5.5 Conclusion
6. Solar energy generation and power prediction through computer vision and machine intelligence
6.1 Introduction
6.2 Computer vision and machine intelligence for solar power prediction
6.3 Fundamentals and need of solar power prediction
6.4 Data-centric methodologies for solar energy production
6.5 Transfer learning perspective in solar energy production
6.6 Case study for implementing multistep forecasting for PV ramp-rate control
6.7 Future perspectives
6.8 Conclusions
Part II Computer vision techniques for renewable energy systems
7. A machine intelligence model based on random forest for data-related renewable energy from wind farms in Brazil
7.1 Introduction
7.2 Literature Review
7.3 Methods
7.4 Results
7.5 Discussion
7.6 Conclusions
8. Bioenergy prediction using computer vision and machine intelligence: modeling and optimization of bioenergy production
8.1 Introduction to bioenergy
8.2 Literature review
8.3 Overview of computer vision in the energy sector
8.4 Advanced deep learning techniques for bioenergy prediction
8.5 Emerging technologies in bioenergy production
8.6 Case studies and experimental results: real-world applications of bioenergy prediction
8.7 Discussion
8.8 Future research directions
8.9 Limitations of the study
8.10 Future suggestions
8.11 Conclusion
9. Artificial intelligence and machine intelligence: modeling and optimization of bioenergy production
9.1 Introduction
9.2 Biomass resource prediction
9.3 Biomass conversion processes
9.4 Biofuel property prediction
9.5 Bioenergy end-use systems
9.6 Generating data for design and optimization
9.7 Technical and interdisciplinary considerations
9.8 Conclusion
10. Advancing bioenergy: leveraging artificial intelligence for efficient production and optimization
10.1 Introduction
10.2 Overview of artificial intelligence and its use in bioenergy prediction
10.3 AI tools to forecast the properties of biomass feedstock
10.4 Prediction and optimization of biochemical conversion technologies using artificial intelligence
10.5 Prediction and optimization of thermochemical conversion technologies using artificial intelligence
10.6 Conclusion
11. Image acquisition and processing techniques for crucial component of renewable energy technologies: mapping of rare earth element-bearing peralkaline granites
11.1 Introduction
11.2 Literature review
11.3 Materials and methods
11.4 Results and discussion
11.5 Conclusion
12. Energy storage using computer vision: control and optimization of energy storage
12.1 Introduction
12.2 Background
12.3 Fundamentals of energy storage systems
12.4 Integration of computer vision in energy storage
12.5 Challenges and Considerations
12.6 Current energy storage status
12.7 Discussion on government initiatives
12.8 Conclusion
13. Classification techniques for renewable energy: identifying renewable energy sources and features
13.1 Historical background of energy
13.2 Introduction
13.3 Classification of energy
13.4 Sources of energy
13.5 Technology-based classification criteria
13.6 Storage technology of renewable energy
13.7 Parameters for different renewable energy sources
13.8 Role of renewable energy in sustainable development
13.9 Importance of renewable energy
13.10 Challenges of renewable energy techniques
13.11 Identifying techniques for renewable energy sources and features
13.12 The environmental impacts of renewable energy sources
13.13 Reducing overall energy demand and complementing renewable energy adoption
13.14 Interconnection of renewable energy with other sectors
13.15 Emerging technologies in renewable energy and potential challenges
13.16 A global overview, including regional analysis of renewable energy
13.17 Comparative results using graphs and tables to demonstrate the utilization of computer vision and machine learning for the classification
13.18 Conclusions
13.19 Discussion
13.20 Future directions
14. Machine learning in renewable energy: classification techniques for identifying sources and features
14.1 Introduction
14.2 Literature review
14.3 Procedures for classification
14.4 Identification and characterization of features
14.5 Case studies and applications
14.6 Challenges and prospects for the future
14.7 Conclusion
15. Advancing the frontier: hybrid renewable energy technologies for sustainable power generation
15.1 Introduction
15.2 Conventional methods used for the development of RE resources
15.3 Renewable energy technologies
15.4 Challenges
15.5 Conclusion
16. Transfer learning for renewable energy: fine-tuning and domain adaptation
16.1 Introduction
16.2 Renewable energy sources
16.3 Artificial intelligence
16.4 Deep learning in renewable energy through sample articles
16.5 Materials and methods
16.6 Discussion and conclusions
Part III Renewable energy sources and computer vision opportunities
17. Exploring the artificial intelligence in renewable energy: a bibliometric study using R Studio and VOSviewer
17.1 Introduction
17.2 Literature review
17.3 Methodology
17.4 Findings
17.5 Discussion
17.6 Conclusion
18. Future directions of computer vision and AI for renewable energy: trends and challenges in renewable energy research and applications
18.1 Introduction
18.2 Computer vision and AI in renewable energy
18.3 Recent advances in computer vision for renewable energy
18.4 Artificial intelligence applications in renewable energy
18.5 Future trends in computer vision and AI for renewable energy
18.6 Challenges and limitations
18.7 Conclusion and future directions
- Edition: 1
- Published: September 20, 2024
- Imprint: Elsevier
- Language: English
AD
Ashutosh Kumar Dubey
AK
Abhishek Kumar
Dr. Abhishek Kumar is Assistant Director and Associate Professor in the Department of Computer Science and Engineering at Chandigarh University, Punjab, India. He completed his PhD in computer science at the University of Madras (India), and previously worked as a post-doctorate fellow in computer science at Ingenium Research Group, based at the Universidad de Castilla-La Mancha in Spain. He has been teaching in academia for more than 13 years, and has over 160 publications in peer reviewed national and international journals, books, and conferences. His research area includes artificial intelligence, renewable energy applications, image processing, computer vision, data mining, and machine learning.
UP
Umesh Chandra Pati
Umesh Chandra Pati is a Professor in the Department of Electronics and Communication Engineering at the National Institute of Technology, India. He has authored/edited two books and published over 100 articles in peer-reviewed international journals and conference proceedings. He has also guest-edited special issues of Cognitive Neurodynamics and International Journal of Signal and Imaging System Engineering. Dr. Pati has filed 2 Indian patents. Besides other sponsored projects, he is currently associated with a high value IMPRINT project “Intelligent Surveillance Data Retriever (ISDR) for Smart City Applications”, an initiative of the Ministries of Education, and Housing and Urban Affairs in the Government of India. His current areas of research include Computer Vision, Artificial Intelligence, the Internet of Things (IoT), Industrial Automation, and Instrumentation Systems.
FG
Fausto Pedro Garcia Marquez
VG
Vicente García-Díaz
AS