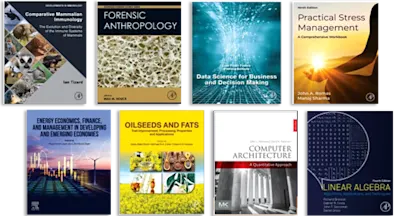
Computational Systems Biology
Inference and Modelling
- 1st Edition - March 22, 2016
- Imprint: Woodhead Publishing
- Authors: Paola Lecca, Angela Re, Adaoha Elizabeth Ihekwaba, Ivan Mura, Thanh-Phuong Nguyen
- Language: English
- Hardback ISBN:9 7 8 - 0 - 0 8 - 1 0 0 0 9 5 - 3
- eBook ISBN:9 7 8 - 0 - 0 8 - 1 0 0 1 1 5 - 8
Computational Systems Biology: Inference and Modelling provides an introduction to, and overview of, network analysis inference approaches which form the backbone of the model of… Read more
Purchase options
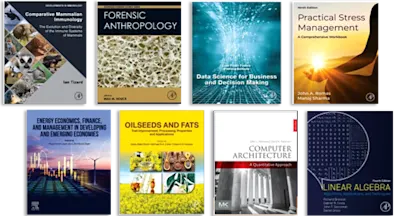
Computational Systems Biology: Inference and Modelling
provides an introduction to, and overview of, network analysis inference approaches which form the backbone of the model of the complex behavior of biological systems.This book addresses the challenge to integrate highly diverse quantitative approaches into a unified framework by highlighting the relationships existing among network analysis, inference, and modeling.
The chapters are light in jargon and technical detail so as to make them accessible to the non-specialist reader. The book is addressed at the heterogeneous public of modelers, biologists, and computer scientists.
- Provides a unified presentation of network inference, analysis, and modeling
- Explores the connection between math and systems biology, providing a framework to learn to analyze, infer, simulate, and modulate the behavior of complex biological systems
- Includes chapters in modular format for learning the basics quickly and in the context of questions posed by systems biology
- Offers a direct style and flexible formalism all through the exposition of mathematical concepts and biological applications
Students and researchers in mathematics, computer science, chemistry and biology willing to address the timely topics of network inference and analysis, model specification and simulation
- Dedication
- About the Authors
- Preface
- Acknowledgments
- Chapter 1: Overview of Biological Network Inference and Modeling of Dynamics
- Abstract
- 1.1 Introduction to Inference of Topologies, Causalities, and Dynamic Models
- 1.2 The Data
- Chapter 2: Network Inference From Steady-State Data
- Abstract
- 2.1 Median-Corrected Z Scores
- 2.2 Multiple Regression Method
- 2.3 Bayesian Variable Selection Method
- Chapter 3: Network Inference From Time-Course Data
- Abstract
- 3.1 Time-Lagged-Correlation-Based Network Inference
- 3.2 Bayesian Approaches
- 3.3 The Method of Variational Bayesian Inference
- Chapter 4: Network-Based Conceptualization of Observational Data
- Abstract
- 4.1 Biological Network Data, Sampling, and Predictability
- 4.2 Characteristics of Biological Networks
- 4.3 Module Discovery Approaches
- 4.4 Categorization of Network Inference Methods
- 4.5 Performance of Network Inference Methods
- 4.6 Comparison of Network Inference Methods
- 4.7 Applications of Network-Based Data Integration
- Chapter 5: Deterministic Differential Equations
- Abstract
- 5.1 The Rationale of Deterministic Modeling
- 5.2 Modeling Elemental and Abstract Biological Phenomena
- 5.3 Analysis of Deterministic Differential Models
- 5.4 Case Studies
- Chapter 6: Stochastic Differential Equations
- Abstract
- 6.1 Reaction Kinetics: The Molecular Approach to Kinetics
- 6.2 Stochastic Differential Equations
- Chapter 7: From Network Inference to the Study of Human Diseases
- Abstract
- 7.1 Introduction to Network Medicine
- 7.2 Databases and Tools for Network Medicine
- 7.3 A Case Study of Neurodegenerative Diseases
- 7.4 Conclusion and Perspectives
- Chapter 8: Conclusions
- Abstract
- 8.1 Network Inference, Modeling, and Simulation in the Era of Big Data and High-Throughput Experiments
- Bibliography
- Index
- Edition: 1
- Published: March 22, 2016
- Imprint: Woodhead Publishing
- Language: English
PL
Paola Lecca
AR
Angela Re
AI
Adaoha Elizabeth Ihekwaba
IM
Ivan Mura
TN