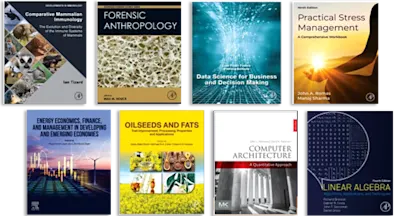
Computational Intelligence Techniques for Sustainable Supply Chain Management
- 1st Edition - May 23, 2024
- Imprint: Academic Press
- Editors: Sanjoy Kumar Paul, Sandeep Kautish
- Language: English
- Paperback ISBN:9 7 8 - 0 - 4 4 3 - 1 8 4 6 4 - 2
- eBook ISBN:9 7 8 - 0 - 4 4 3 - 1 8 4 6 5 - 9
Computational Intelligence Techniques for Sustainable Supply Chain Management presents state-of-the-art computational intelligence techniques and applications for supply chain su… Read more
Purchase options
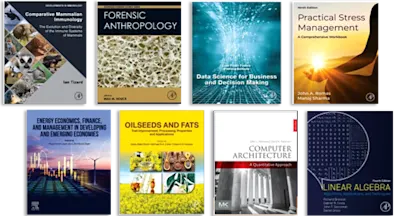
Computational Intelligence Techniques for Sustainable Supply Chain Management presents state-of-the-art computational intelligence techniques and applications for supply chain sustainability issues and logistic problems, filling the gap between general textbooks on sustainable supply chain management and more specialized literature dealing with methods for computational intelligence techniques. This book focuses on addressing problems in advanced topics in the sustainable supply chain and will appeal to practitioners, managers, researchers, students, and professionals interested in sustainable logistics, procurement, manufacturing, inventory and production management, scheduling, transportation, and supply chain network design.
- Serves as a reference on computational intelligence–enabled sustainable supply chains for graduate students in computer/data science, industrial engineering, industrial ecology, and business
- Explores key topics in sustainable supply chain informatics, that is, heuristics, metaheuristics, robotics, simulation, machine learning, big data analytics and artificial intelligence
- Provides a foundation for industry leaders and professionals to understand recent and cutting-edge methodologies and technologies in the domain of sustainable supply chain powered by computational intelligence techniques
Academicians and industry researchers and practitioners, supply chain and logistics practitioners, professionals in logistics, transportation, and distribution, production and inventory management Professionals
2. Big Data Analytics in Construction: Laying the Groundwork for Improved Project Outcomes
3. Role of UAVs in Sustainable Supply Chain: Queuing theory and Ant Colony Optimization Approach
4. Applying Machine Learning to investigate the enablers of Sustainable Supply Chain Management
5. Scenario Analysis for Long-term Planning: Stratified Decision-Making Models in Sustainable Supply Chains
6. Machine Learning Techniques for Sustainable Industrial Process Control
7. Intelligent Sustainable Infrastructure for procurement and distribution
8. Machine Learning Techniques for Route Optimizations and Logistics Management Description
9. Improving Sustainable Supply Chain through Robotics
10. Computational techniques for green procurement and production
11. Predictive big data analytics for supply chain demand forecasting
12. Bayesian Network Based on Cross Bow-tie to Analyze Differential Effects of Internal and External Risks on Sustainable Supply Chain
13. Optimizing Supply Chain Operations through Digitalization and Customer-centric Strategies: A Case Study of Bigbasket.com
14. Applications of Artificial Intelligence in Echo Global Logistics
- Edition: 1
- Published: May 23, 2024
- Imprint: Academic Press
- Language: English
SP
Sanjoy Kumar Paul
Sanjoy Kumar Paul, PhD is an Associate Professor in operations and supply chain management at the University of Technology Sydney (UTS), Sydney, Australia. He has published more than 140 articles in top-tier journals. He is also an associate editor, area editor, editorial board member, and active reviewer of several reputed journals. Dr. Paul has received several awards, including the ASOR Rising Star Award from the Australian Society for Operations Research, the Excellence in Early Career Research Award from the UTS Business School, and the Stephen Fester Prize for most outstanding thesis from UNSW. Based on his citation records in 2020, 2021 and 2022, he was included in the top 2% of scientists in author databases of standardized citation indicators. His research interests include sustainable supply chain management, supply chain resilience, applied operations research, modeling and simulation, and intelligent decision-making.
SK
Sandeep Kautish
Sandeep Kautish, PhD is Professor and Director at Apex Institute of Technology (AIT-CSE), Chandigarh University, Punjab India and an academician by choice and has more than 20 years of full-time experience in teaching and research. He has been associated with Asia Pacific University Malaysia for over five years at their TNE site at Kathmandu Nepal in the capacity of Director-Academics. He earned his doctorate degree in Computer Science on Intelligent Systems in Social Networks. He has over 100 publications and his research works have been published in highly reputed journals, i.e., IEEE Transaction of Industrial Informatics, IEEE Access, and Multimedia Tools and Applications, etc. Dr. Kautish has edited 24 books with leading publishers, i.e., Elsevier, Springer, Emerald, and IGI Global, and is an editorial member/reviewer of various reputed journals. His research interests include healthcare analytics, business analytics, machine learning, data mining, and information systems.