Computational Intelligence Methods for Sentiment Analysis in Natural Language Processing Applications
- 1st Edition - January 19, 2024
- Editor: D. Jude Hemanth
- Language: English
- Paperback ISBN:9 7 8 - 0 - 4 4 3 - 2 2 0 0 9 - 8
- eBook ISBN:9 7 8 - 0 - 4 4 3 - 2 2 0 1 0 - 4
Computational Intelligence for Sentiment Analysis in Natural Language Processing Applications provides a solution to this problem through detailed technical coverage of AI-bas… Read more
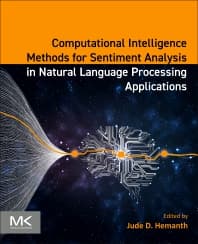
Purchase options
Institutional subscription on ScienceDirect
Request a sales quoteComputational Intelligence for Sentiment Analysis in Natural Language Processing Applications provides a solution to this problem through detailed technical coverage of AI-based Sentiment Analysis methods for various applications. The book's authors provide readers with an in-depth look at the challenges and associated solutions, including case studies and real-world scenarios from across the globe. Development of scientific and enterprise applications are covered that will aid computer scientists in building practical/real-world AI-based Sentiment Analysis systems. With the vast increase in Big Data, computational intelligence approaches have become a necessity for Natural Language Processing and Sentiment Analysis in a wide range of decision-making application areas.
- Includes basic concepts, technical explanations, and case studies for in-depth explanation of the Sentiment Analysis
- Aids computer scientists in developing practical/real-world AI-based Sentiment Analysis systems
- Provides readers with real-world development applications of AI-based Sentiment Analysis, including transfer learning for opinion mining from pandemic medical data, sarcasm detection using neural networks in human-computer interaction, and emotion detection using the random-forest algorithm
Computer Scientists and researchers in Computational Intelligence and Machine Learning, specifically in the field of developing Natural Language Processing algorithms and applications. As such, academics, researchers, and professionals in a variety of research fields who work with AI, algorithms, and Machine Learning and their applications to Sentiment Analysis will be a target audience. Linguistics researchers, social science researchers, management science researchers, government and non-government organizations, data analysts, and engineers working on NLP and Sentiment Analysis
1. Role of Machine Learning in Sentiment Analysis: Trends, Challenges and Future Directions Vidyasagar Shetty and Shabari Shedthi 2. A Comparative analysis of Machine Learning and Deep Learning Techniques for Aspect-based Sentiment Analysis Getzi Jeba Leelipushpam Paulraj, Theresa V. Cherian, Joyce Beryl Princess and Immanuel Johnraja Jebadurai 3. A systematic survey on Text-based Dimensional Sentiment Analysis: Advancements, Challenges and Future directions Saroj Date and Sahin Deshmukh 4. A model of time in Natural Linguistic Reasoning Daniela López De Luise and Sebastian Cippitelli 5. Hate speech detection using LSTM network from Twitter speech data Ravi Shekhar Tiwari 6. Enhanced Performance of Drug Review Classification from Social Network by ADASYN Training and NLP Techniques P.M. Lavanya and E Sasikala 7. Emotion Detection from Text Data using Machine Learning for Human Behavior Analysis Muskan Garg and Chandni Saxena 8. Optimization of Effectual Sentiment Analysis in Film Reviews using Machine Learning Techniques S. Balamurugan 9. Deep Learning for Double Negative Detection in Text Data for Customer Feedback Analysis on a Product Deepika Ghai, Suman Lata Tripathi, Ramandeep Sandhu, Ranjit Kaur, Mohammad Faiz and Gurleen Kaur Walia 10. Sarcasm Detection using Deep Learning in Natural Language Processing Santhanam Lakshmi 11. Abusive comment detection in Tamil Language using Deep Learning Vedika Gupta, Deepawali Sharma and Vivek Kumar Singh 12. Implementation of Sentiment Analysis in Stock Market Prediction using variants of GARCH models Vijayalakshmi V 13. A Metaheuristic Harmony Search Optimization Based Approach for Hateful and Offensive Speech Detection in social media S. Saroja and S Haseena
- No. of pages: 294
- Language: English
- Edition: 1
- Published: January 19, 2024
- Imprint: Morgan Kaufmann
- Paperback ISBN: 9780443220098
- eBook ISBN: 9780443220104
DH
D. Jude Hemanth
Dr. D. Jude Hemanth is currently working as a professor in Department of ECE, Karunya University, Coimbatore, India. He also holds the position of “Visiting Professor” in Faculty of Electrical Engineering and Information Technology, University of Oradea, Romania. He also serves as the “Research Scientist” of Computational Intelligence and Information Systems (CI2S) Lab, Argentina; LAPISCO research lab, Brazil; RIADI Lab, Tunisia; Research Centre for Applied Intelligence, University of Craiova, Romania and e-health and telemedicine group, University of Valladolid, Spain.
Dr. Hemanth received his B.E degree in ECE from Bharathiar University in 2002, M.E degree in communication systems from Anna University in 2006 and Ph.D. from Karunya University in 2013. He has published 37 edited books with reputed publishers such as Elsevier, Springer and IET. His research areas include Computational Intelligence and Image processing. He has authored more than 200 research papers in reputed SCIE indexed International Journals and Scopus indexed International Conferences.
Affiliations and expertise
Professor, ECE Department, Karunya Institute of Technology and Sciences, Coimbatore, IndiaRead Computational Intelligence Methods for Sentiment Analysis in Natural Language Processing Applications on ScienceDirect