LIMITED OFFER
Save 50% on book bundles
Immediately download your ebook while waiting for your print delivery. No promo code needed.
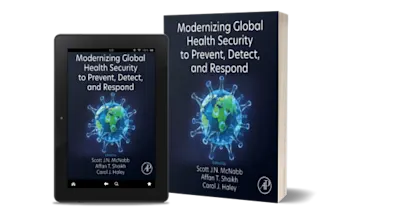
Computational Intelligence for Genomics Data presents an overview of machine learning and deep learning techniques being developed for the analysis of genomic data and the develo… Read more
LIMITED OFFER
Immediately download your ebook while waiting for your print delivery. No promo code needed.
BP
Babita Pandey completed her PhD in the field of artificial intelligence in biomedical signal processing from IIT-BHU, Varanasi. Currently, she is an Associate Professor of Computer Science at Babasaheb Bhimrao Ambedkar University, Lucknow. She has published more than 150 research papers in refereed journals and conferences. She has delivered many expert lectures for students as well as in faculty development programs. She has worked as a session chair, conference steering committee member, editorial board member, and peer reviewer in various international/national conferences. She received the “Research Excellence Award” in 2014, 2015, 2016 from Lovely Professional University and the “Research and Academic Excellence Award” in 2021, 2022 at Babasaheb Bhimrao Ambedkar University, Lucknow, India. She also received the P.D. Sethi Award 2022. She edited one book on link prediction in social networks. Her areas of research include link prediction, dimensionality reduction of genomic data, e-learning, machine learning and deep learning deployed for images.
VE
ST
Suman Lata Tripathi completed her PhD in the area of microelectronics and VLSI from MNNIT, Allahabad. She was also a remote post-doc researcher at Nottingham Trent University, London, UK in 2022. She is a Professor at Lovely Professional University with more than 19 years of experience in academics. She has published more than 89 research papers in refereed journals and conferences. She has also published 13 Indian patents and 2 copyrights. She has organized several workshops, summer internships, and expert lectures for students. She has worked as a session chair, conference steering committee member, editorial board member, and peer reviewer in international/national conferences. She received the “Research Excellence Award” in 2019 and “Research Appreciation Award” in 2020, 2021 at Lovely Professional University, India. She also received funded projects from SERB DST under the scheme TARE in the area of Microelectronics devices. She has edited or authored more than 15 books in different areas of Electronics and electrical engineering. Her areas of expertise includes microelectronics device modeling and characterization, low power VLSI circuit design, VLSI design of testing, and advanced FET design for IoT, Embedded System Design, reconfigurable architecture with FPGAs and biomedical applications.
DP
Devendra Kumar Pandey completed his PhD in Bioprocess Engineering from IIT (BHU), Varanasi. He has been a Professor at Lovely Professional University for more than 22 years. He has published more than 112 research papers in refereed journals and conferences. He received the “Research Excellence Award” in 2019 and “Research Appreciation Award” in 2020, 2021 at Lovely Professional University, India. He has also received funded projects from UPCST under the area of Medicinal Plants. His areas of expertise include Bioprocess Engineering, Medicinal Plant Biotechnology, Plant-Microbe interaction, Computational Biology.
MM